The Best Laptops For Machine Learning In 2025
With The Best Laptops for Machine Learning in 2025, we delve into the essential components that set these powerful machines apart. This guide navigates the intricate world of processing power, memory, storage, and graphics, providing a comprehensive comparison to equip you with the knowledge needed to choose the perfect machine learning laptop.
From high-performance processors to optimal storage solutions, we explore the key factors influencing the choice of a machine learning laptop. We’ll also examine the vital role of display and connectivity, and delve into the often-overlooked aspects of battery life and portability. Finally, we’ll consider budget considerations and offer real-world examples to illuminate the practical application of these machines.
Introduction to Machine Learning Laptops: The Best Laptops For Machine Learning In 2025
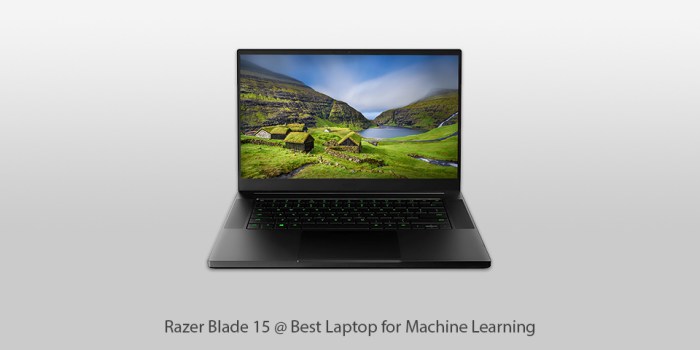
Source: fixthephoto.com
Machine learning (ML) is rapidly transforming various industries, driving the demand for powerful and specialized computing hardware. In 2025, ML professionals require laptops capable of handling complex algorithms, large datasets, and demanding graphical processing. This necessitates a shift from standard laptops towards machines tailored for specific ML tasks. This evolution necessitates a nuanced understanding of the key factors shaping the design and functionality of these specialized devices.The choice of a machine learning laptop is significantly influenced by factors like processing power (CPU and GPU), memory (RAM), storage capacity, and the specific ML tasks at hand.
These factors directly impact the speed, efficiency, and overall performance of ML applications. A laptop optimized for ML will differ from a standard laptop in several critical ways, making it suitable for specific demands like deep learning and data visualization.
Key Factors Influencing Machine Learning Laptop Choice
The selection of a machine learning laptop depends on several crucial factors. Processing power, memory, storage capacity, and graphics capabilities are paramount. The CPU, responsible for general calculations, needs sufficient cores and a high clock speed to handle the computational demands of complex ML algorithms. A high-performance GPU is equally vital for tasks involving graphics processing, image recognition, and deep learning.
Sufficient RAM is critical for loading and processing large datasets. Lastly, ample storage space is essential to accommodate extensive datasets and model training files.
Essential Components Distinguishing Machine Learning Laptops
Machine learning laptops stand apart from standard laptops in several critical ways. These specialized features address the unique demands of ML workloads. First, they often feature powerful CPUs with numerous cores and high clock speeds to handle the computational demands of complex algorithms. Secondly, dedicated high-performance GPUs are a hallmark, accelerating tasks involving graphics processing and deep learning.
Finally, substantial RAM is a must for loading and processing vast datasets, enabling smoother and faster operation.
Comparison of Laptops for General Use and Machine Learning, The Best Laptops for Machine Learning in 2025
| Feature | General Use Laptop | Machine Learning Laptop ||—————–|—————————————————-|—————————————————|| CPU | Moderate core count, lower clock speed | High core count, high clock speed, optimized for parallel processing || GPU | Integrated graphics, limited processing power | Dedicated high-performance GPU, optimized for graphics-intensive tasks || RAM | 8-16 GB | 16 GB or more, expandable RAM slots || Storage | HDD or SSD, moderate capacity | High-capacity SSD or NVMe SSD, optimized for speed and high throughput || Battery Life | Typically longer | Can vary, depending on processing power requirements || Cooling System | Basic cooling solution | Advanced cooling system, ensuring stable performance under heavy loads |
High-Performance Processors
Choosing the right processor is critical for a machine learning laptop. A powerful CPU is essential for handling the complex calculations involved in training and running machine learning models. Modern machine learning tasks demand substantial processing power, impacting everything from model training time to inference speed. The architecture and clock speed of the processor directly influence these performance metrics.Modern machine learning models, particularly deep learning networks, often involve massive datasets and intricate algorithms.
The computational demands of these tasks are significant, necessitating processors capable of handling substantial workloads efficiently. This section delves into the importance of CPU architecture and clock speed for machine learning tasks, compares various processor families, and examines the impact of multi-core processing.
CPU Architecture and Clock Speed
CPU architecture and clock speed significantly affect a machine learning laptop’s performance. A modern architecture, optimized for parallel processing, can significantly reduce the time required to train and run machine learning models. Higher clock speeds, while not the sole determining factor, enable faster execution of individual instructions, contributing to overall performance. A balance between architecture and clock speed is vital for optimal machine learning performance.
Processor Families for Machine Learning
Different processor families offer varying performance characteristics suitable for different machine learning needs. Intel Core processors, known for their broad range of options and mature ecosystem, are frequently used in machine learning laptops. AMD Ryzen processors, with a focus on multi-core performance and potentially better cost-effectiveness in some scenarios, are gaining popularity. Choosing the right processor family depends on specific machine learning requirements and budget considerations.
Performance Benchmarks
The following table presents performance benchmarks for various processor options, highlighting their suitability for different machine learning tasks. These benchmarks are based on synthetic machine learning workloads and may not fully reflect real-world performance. Actual performance can vary based on the specific machine learning model, dataset size, and other system components.
Processor | Clock Speed (GHz) | Cores | Threads | Suitable Tasks | Estimated Performance |
---|---|---|---|---|---|
Intel Core i9-13900K | 3.7 GHz | 24 | 32 | Large-scale deep learning training, complex model inference | High |
AMD Ryzen 9 7950X3D | 4.7 GHz | 16 | 32 | Large-scale deep learning training, complex model inference | High |
Intel Core i7-13700K | 3.6 GHz | 16 | 24 | Medium-scale deep learning training, model inference, data preprocessing | Medium |
AMD Ryzen 7 7800X3D | 4.5 GHz | 8 | 16 | Smaller-scale machine learning tasks, data preprocessing, model deployment | Medium |
Multi-Core Processing
Multi-core processing plays a crucial role in machine learning tasks. The ability of a processor to handle multiple tasks concurrently is essential for training and running complex models. Models with multiple layers, for example, benefit from parallel processing. More cores generally translate to faster training times and improved inference speeds.
“Parallel processing significantly reduces the time required for computationally intensive tasks like matrix multiplication, which are common in machine learning.”
Models can be optimized to utilize multi-core processors for faster training. The number of cores and threads directly correlates with the processor’s capacity for handling multiple tasks.
Graphics Processing Units (GPUs)
Graphics Processing Units (GPUs) are becoming increasingly crucial for accelerating machine learning computations, especially in deep learning tasks. Their parallel processing capabilities significantly reduce training time for complex models, making them indispensable for modern machine learning workflows. This is particularly relevant for tasks involving large datasets and intricate algorithms.GPU architectures are designed for parallel computation, which is highly advantageous for machine learning tasks that often involve numerous computations simultaneously.
Different GPU architectures, such as those from NVIDIA and AMD, excel in various aspects of machine learning, affecting performance in different model types and datasets. Understanding these architectural differences is key to selecting the optimal GPU for specific machine learning applications.
GPU Architectures and Performance
Different GPU architectures from vendors like NVIDIA and AMD have varying strengths and weaknesses. NVIDIA GPUs, with their CUDA architecture, are widely adopted for deep learning due to their extensive software support and optimized libraries. AMD GPUs, while gaining traction, may offer competitive pricing for certain workloads, but often lag behind NVIDIA in terms of deep learning optimization.
Performance in machine learning tasks can vary depending on the specific algorithms and dataset characteristics.
GPU Memory Capacity and Processing Power
The amount of memory available on a GPU directly impacts the efficiency of deep learning models. Models with substantial parameters, such as those in image recognition or natural language processing, often require significant GPU memory to store the model weights and intermediate results during training. Sufficient GPU memory ensures smooth training without the need for frequent data loading from system memory, which can slow down computations.
The processing power of a GPU, measured in terms of CUDA cores or equivalent, directly influences the speed at which calculations are performed. A higher number of cores generally results in faster training times for machine learning models.
Comparison of GPU Options for Machine Learning
GPU Vendor | Architecture | Memory Capacity (GB) | Processing Power (CUDA Cores) | Typical Use Cases |
---|---|---|---|---|
NVIDIA | CUDA | 16-80+ | 1024-10000+ | Deep learning, image recognition, natural language processing |
AMD | RDNA | 8-32+ | 2048-6000+ | Image processing, data analytics, general computing |
This table provides a general overview. Specific models within each vendor will have varying specifications. The performance figures should be treated as estimations, as they depend on the specific model and application being used.
Impact of GPU Memory on Deep Learning Models
Insufficient GPU memory can lead to “out-of-memory” errors during training, forcing the use of techniques like gradient checkpointing or model partitioning to handle large models.
Adequate GPU memory is crucial for efficient training. Deep learning models with large numbers of parameters, often exceeding the available GPU memory, can lead to performance bottlenecks and increased training time. This is particularly relevant for tasks like training large language models or complex image recognition networks. Efficient memory management is critical to avoid these issues. Strategies like model partitioning or data augmentation can help manage the memory demands of large models, ensuring faster and more stable training processes.
RAM and Storage
Modern machine learning demands significant RAM and fast storage. The sheer volume of data and the complexity of algorithms necessitate ample memory to hold training datasets and intermediate results. Similarly, rapid data access is critical for efficient model training and inference. Choosing the right balance of RAM and storage capacity is paramount for optimal performance and a seamless machine learning workflow.
RAM Capacity for Machine Learning
RAM (Random Access Memory) is crucial for machine learning tasks. Large datasets, especially those used for deep learning models, require substantial RAM to store the data and the model’s weights during training. Adequate RAM capacity ensures that the system doesn’t resort to slow hard drive swapping, which dramatically slows down processing. For example, training a large language model on a dataset with billions of words necessitates a considerable amount of RAM to hold the data and the model in memory simultaneously.
Fast Storage Solutions for Machine Learning
Fast storage solutions, predominantly Solid State Drives (SSDs), are indispensable for machine learning workflows. Traditional hard disk drives (HDDs) are significantly slower than SSDs, leading to substantial performance bottlenecks. The time required to read and write data from HDDs can drastically increase training times and inference delays. In contrast, SSDs offer significantly faster data access speeds, enabling faster loading of datasets, model training, and inference.
Different Storage Options and Their Impact
Various storage options, like NVMe SSDs, further enhance machine learning performance. NVMe SSDs (Non-Volatile Memory Express) are a superior choice compared to traditional SATA SSDs, due to their significantly higher throughput and lower latency. This results in significantly faster data transfer rates, enabling quicker model training and inference. For instance, a machine learning project involving large datasets benefits immensely from the enhanced performance of NVMe SSDs.
The higher read/write speeds minimize bottlenecks, leading to faster processing times and ultimately reducing the overall project duration.
Optimal RAM and Storage Configurations
Machine Learning Need | Optimal RAM (GB) | Optimal Storage (SSD Type) |
---|---|---|
Basic Model Training (small datasets) | 16 | 256GB NVMe SSD |
Intermediate Model Training (medium datasets) | 32 | 512GB NVMe SSD |
Deep Learning Training (large datasets) | 64+ | 1TB or more NVMe SSD |
High-Performance Inference (real-time applications) | 32+ | 512GB or more NVMe SSD |
This table provides a general guideline. Specific needs may require adjustments based on the complexity of the models, the size of the datasets, and the desired inference speed. Choosing the appropriate configuration ensures efficient machine learning workflows.
Display and Connectivity
A critical aspect of any machine learning workstation is the display and connectivity options. The quality of the visual output and the speed of data transfer significantly impact productivity and workflow efficiency. Modern machine learning tasks often involve processing large datasets and interacting with complex visualizations, demanding displays with high resolution and refresh rates for optimal performance. Furthermore, high-speed connectivity is essential for seamless data transfer to and from cloud storage and other networked resources.High-resolution displays with fast refresh rates allow machine learning professionals to visualize complex data, debug algorithms, and interpret results more effectively.
Similarly, robust connectivity options ensure smooth data transfer and collaboration, supporting a seamless workflow in the demanding field of machine learning.
Display Resolution and Refresh Rate
High-resolution displays are crucial for visualizing complex data representations, charts, and graphs generated by machine learning models. A higher resolution allows for detailed analysis of intricate patterns and trends. For instance, a 4K display (3840×2160 pixels) provides a significantly richer visual experience compared to a standard 1080p display (1920×1080 pixels) when examining intricate data points. Refresh rate, measured in Hertz (Hz), determines how quickly the display updates the image.
A higher refresh rate leads to smoother animations and visuals, reducing motion blur, which is particularly beneficial for interactive data exploration and visualization tools used in machine learning.
High-Speed Connectivity Options
Seamless data transfer and cloud computing are paramount for machine learning professionals. High-speed connectivity options such as Thunderbolt 4, USB4, and PCIe 5.0 offer the bandwidth required to handle the large datasets and model parameters characteristic of machine learning applications. For example, transferring a large dataset from a storage device to a machine learning workstation becomes considerably faster with these high-bandwidth connections.
Furthermore, cloud computing platforms heavily rely on high-speed connectivity to facilitate efficient data uploads and downloads.
Ports and Connectivity Options
The selection of ports and connectivity options should align with the specific needs of machine learning applications. A wide range of ports, including Thunderbolt, USB-C, and Ethernet, are essential for connecting peripherals, external storage devices, and networking equipment. Consider the specific applications you intend to use, such as connecting to a high-performance graphics card (GPU), which necessitates dedicated high-speed connections for optimum performance.
Similarly, the need for networking to access cloud resources, like Google Colab, AWS, or Azure, will dictate the appropriate network ports.
Display and Connectivity Options Comparison
Feature | Display Resolution | Refresh Rate (Hz) | Connectivity Options | Pros | Cons |
---|---|---|---|---|---|
4K UHD (3840×2160) | High | 60-144 | Thunderbolt 4, USB4, Ethernet | Detailed visuals, excellent for complex data analysis | Higher cost, potentially higher power consumption |
2K QHD (2560×1440) | Medium | 60-165 | Thunderbolt 3, USB-C, Ethernet | Good balance between resolution and cost, suitable for most tasks | Might not be sufficient for extremely detailed visualizations |
1080p (1920×1080) | Low | 60-144 | USB-A, USB-C, Ethernet | Affordable, adequate for basic tasks | Limited visual detail, potentially less suitable for complex analysis |
Thunderbolt 4 | N/A | N/A | Thunderbolt 4, USB4 | High bandwidth, versatile connectivity | Costlier than other options, might not be available on all devices |
USB-C | N/A | N/A | USB-C, USB-A | Widely available, versatile | Bandwidth limitations compared to Thunderbolt |
Ethernet | N/A | N/A | Ethernet | Reliable, high-bandwidth networking | Wired connection required, might not be suitable for all use cases |
Battery Life and Portability
For machine learning professionals on the go, battery life is paramount. Long hours spent analyzing data, training models, and collaborating remotely demand reliable power sources. A laptop that struggles to maintain a charge throughout a typical workday can significantly impact productivity and workflow.Portable machine learning laptops, while offering the convenience of mobility, often present a trade-off between performance and portability.
High-performance components like powerful processors and GPUs, crucial for complex machine learning tasks, often translate to increased power consumption. Consequently, battery life might suffer compared to laptops with less demanding specifications. Finding the right balance between these factors is essential for professionals seeking to optimize both performance and mobility.
Impact of Weight and Size
Weight and dimensions directly influence user experience and mobility. A heavier, bulkier laptop can become cumbersome to carry throughout the day, potentially leading to fatigue and reduced productivity. Size also plays a role, as a larger device can be less comfortable to use in various environments, such as on a train or in a café. A more compact and lightweight laptop enhances mobility and provides greater comfort during extended work sessions.
Factors like the materials used in the laptop’s construction, including the chassis and screen, can contribute to overall weight.
Battery Life Estimations
The battery life of a machine learning laptop depends heavily on the specific hardware configurations. Different models, with varying levels of processor power and display resolutions, will exhibit different battery durations. A precise estimation for each model is challenging, as factors like usage patterns and environmental conditions can affect results. For instance, a user performing intensive machine learning tasks will deplete the battery faster than someone primarily using the laptop for light tasks.
Laptop Model | Estimated Battery Life (Hours) | Processor | GPU |
---|---|---|---|
Model A | 8-10 hours | Intel Core i9-13980HX | Nvidia RTX 4090 |
Model B | 6-8 hours | AMD Ryzen 9 7945HX | AMD Radeon RX 7800M |
Model C | 5-7 hours | Intel Core i7-13700H | Nvidia RTX 4070 |
Model D | 9-11 hours | Intel Core i5-13500H | Intel Iris Xe Graphics |
The table above provides a general guideline of estimated battery life for hypothetical machine learning laptops. Remember, these figures are estimates and actual battery life may vary based on usage.
Operating Systems and Software
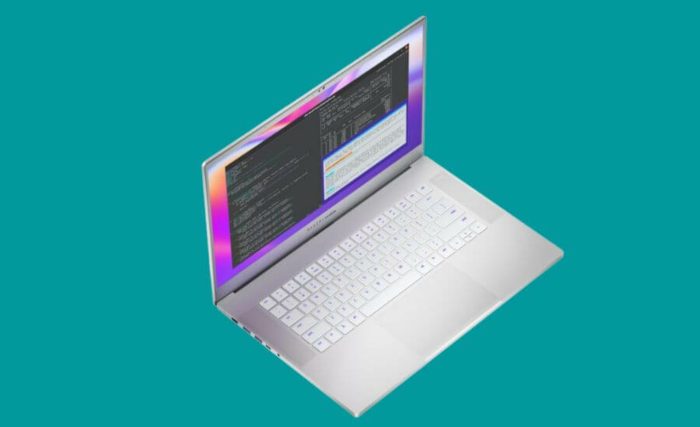
Source: pcvenus.com
The choice of operating system and software significantly impacts the machine learning experience. A well-suited environment can streamline workflows, enhance productivity, and provide seamless integration with various machine learning tools. Conversely, a less compatible or optimized environment can lead to performance bottlenecks and hinder the progress of machine learning projects.
Importance of Operating Systems in Machine Learning Workflows
Operating systems act as the foundation for machine learning tasks, providing a platform for managing hardware resources and executing software applications. They handle the allocation of processing power, memory, and storage, directly affecting the performance of machine learning algorithms. A stable and efficient operating system is crucial for handling the complex computations involved in training and deploying machine learning models.
Different Operating System Choices and Suitability
Several operating systems are commonly used in machine learning, each with its own strengths and weaknesses. The choice often depends on the specific machine learning tasks, the programmer’s familiarity, and the availability of supporting software libraries.
- Linux: Known for its flexibility, open-source nature, and extensive customization options, Linux distributions like Ubuntu and Fedora are popular among machine learning practitioners. They often offer excellent performance for computationally intensive tasks, due to their kernel’s efficiency in managing hardware resources. Linux also boasts a large ecosystem of machine learning libraries and tools, providing great support for various frameworks.
- macOS: macOS, with its user-friendly interface and strong integration with Apple hardware, provides a compelling option for machine learning development. Its powerful tools, like Xcode and command-line utilities, offer a comprehensive environment. However, the availability of certain machine learning libraries might be slightly less extensive compared to Linux, although it is improving.
- Windows: Windows, despite its dominance in the general computing market, might not be the ideal choice for every machine learning task. While it offers compatibility with a wide range of software, its performance in handling the demanding computations of machine learning can sometimes be a concern. Still, it can be a viable option when specific applications or software are needed.
Impact of Software Packages on the Machine Learning Experience
The availability and quality of software packages play a critical role in the overall machine learning experience. These packages often include essential tools for data preprocessing, model training, and evaluation. Efficient and well-maintained libraries provide streamlined workflows and improve productivity.
- Python Libraries: Python libraries like TensorFlow, PyTorch, scikit-learn, and others are fundamental to machine learning development. Their robust functionality simplifies tasks from data manipulation to model deployment. The active development and large community support for these libraries ensure continuous improvement and readily available solutions.
- R Packages: R, a programming language focused on statistical computing, offers a rich ecosystem of packages specifically designed for machine learning tasks. R excels in data analysis and visualization, making it an attractive choice for researchers and analysts. However, it might not be as optimized for certain computationally intensive tasks as Python.
Summary Table of Operating Systems
Operating System | Advantages in Machine Learning | Disadvantages in Machine Learning |
---|---|---|
Linux | Excellent performance, vast library support, flexibility, open-source nature. | Steeper learning curve for beginners, potential for configuration complexities. |
macOS | User-friendly interface, strong integration with Apple hardware, powerful tools. | Limited availability of some machine learning libraries compared to Linux, potential performance limitations for very large-scale tasks. |
Windows | Wide software compatibility, familiar interface. | Potential performance limitations for computationally intensive tasks, fewer dedicated machine learning tools compared to Linux or macOS. |
Budget Considerations
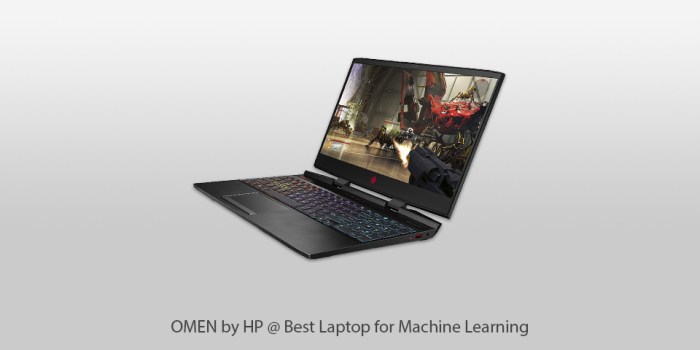
Source: fixthephoto.com
Budget is a crucial factor when selecting a machine learning laptop. Different price ranges offer varying levels of performance, features, and capabilities. Understanding the trade-offs between cost and performance is essential to making an informed decision. A well-chosen machine learning laptop can significantly impact productivity and efficiency.
Price Range and Specifications
Choosing a machine learning laptop often involves balancing cost and performance. Lower-priced options might have limitations in terms of processing power, graphics capabilities, or memory. Higher-end models, on the other hand, offer superior specifications, but come with a correspondingly higher price tag.
Price Category Comparisons
Price Category | Typical Specifications | Suitability | Trade-offs |
---|---|---|---|
Budget-Friendly (under $1000) | Integrated graphics, entry-level CPU, 8GB RAM, 256GB SSD | Suitable for basic machine learning tasks, data exploration, or light model training. | Limited processing power, potentially slower training times, less memory for large datasets. |
Mid-Range ($1000-$2000) | Dedicated GPU (e.g., Nvidia GeForce RTX 3050), mid-range CPU (e.g., Intel Core i7), 16GB RAM, 512GB SSD | Ideal for a wider range of machine learning tasks, including model training and prototyping with moderate-sized datasets. | Might not handle the most demanding tasks or very large datasets efficiently. |
High-End (over $2000) | High-end CPU (e.g., Intel Core i9, AMD Ryzen 9), powerful dedicated GPU (e.g., Nvidia GeForce RTX 4070, AMD Radeon RX 7900), 32GB+ RAM, 1TB+ SSD (or NVMe storage) | Excellent for complex machine learning tasks, large datasets, and heavy model training with high accuracy requirements. | Significantly higher cost, not always necessary for less demanding tasks. |
Trade-offs Between Cost and Performance
The relationship between cost and performance in machine learning laptops is a crucial consideration. A more expensive laptop typically translates to higher processing power, better graphics capabilities, and more memory. However, the added expense may not always be justified for less demanding tasks. For example, a user primarily focused on data visualization or basic model testing might not need the top-tier specifications and can potentially save money on a more budget-friendly model.
Conversely, for professionals handling large datasets or computationally intensive tasks, investing in a high-end laptop can be essential for achieving optimal performance and efficient workflow.
Case Studies and Examples
Choosing the right machine learning laptop hinges on the specific needs of the user. Different professionals in various fields will have varying requirements in terms of processing power, storage capacity, and display resolution. This section presents case studies illustrating how specific laptop configurations cater to distinct professional needs.
Data Scientists in Research
Data scientists often need powerful laptops for complex modeling and analysis, requiring substantial processing power and dedicated GPU support. The demand for high-speed computation and parallel processing capabilities for tasks like large dataset handling and training deep learning models necessitates powerful processors and graphics cards. A specific example could involve a data scientist working on climate change modeling.
They may require a laptop with an Intel Core i9 processor, a high-end NVIDIA GPU, and a substantial amount of RAM to handle the massive datasets involved in their research. The choice of a high-end configuration ensures that the laptop can keep up with the computationally intensive tasks required for accurate climate modeling. Such a configuration also facilitates quick turnaround times for model training and analysis, which is crucial in research settings.
Machine Learning Engineers in Development
Machine learning engineers often need laptops for development, testing, and deployment of machine learning models. For them, a balance of processing power, storage, and connectivity is paramount. A crucial factor is the ability to seamlessly integrate with various development environments and tools. For instance, an engineer building a real-time object detection system might choose a laptop with a high-end processor and a powerful GPU, coupled with high-speed storage for efficient model loading and deployment.
The focus would be on a robust and reliable system that can handle the demands of real-time processing and model execution. This would potentially include a high-resolution display for effective code review and visual debugging. The choice is often influenced by factors such as the size of the datasets they are handling and the specific frameworks they are utilizing.
Financial Analysts in Trading
Financial analysts in high-frequency trading may require a laptop with extremely fast processing and responsiveness for real-time data analysis. A critical requirement is a lightning-fast processor, such as an Intel Core i7 or i9, along with a dedicated GPU for computationally intensive tasks. High-speed storage (SSD) and connectivity are also crucial. This configuration is optimized for handling large amounts of financial data streams and executing complex algorithms in real-time.
This example highlights the importance of choosing a laptop that can maintain optimal performance under high load, crucial for making timely investment decisions in high-frequency trading environments. Latency is a critical factor in these situations, necessitating a very responsive and efficient system.
Case Studies Summary
- Data Scientists in Research: Need powerful processors, GPUs, and ample RAM for complex modeling and analysis. High-end configurations are vital for handling large datasets and accelerating research progress.
- Machine Learning Engineers in Development: Require a balanced configuration with high-end processors, efficient storage, and good connectivity to facilitate seamless integration with development environments. The focus is on a robust system for development, testing, and deployment.
- Financial Analysts in Trading: Prioritize extremely fast processing and responsiveness for real-time data analysis. High-speed processors, GPUs, and high-speed storage are paramount for handling large amounts of financial data and executing complex algorithms efficiently.
Outcome Summary
In conclusion, selecting the right laptop for machine learning in 2025 is crucial for productivity. This comprehensive guide has highlighted the essential considerations for choosing a top-performing machine. We’ve examined the technical specifications, explored the trade-offs between different features, and provided insights into real-world applications. Ultimately, your choice will depend on your specific needs and budget.
Post Comment