Greatest Dangers of AI A Critical Look
Greatest dangers of AI sets the stage for this enthralling narrative, offering readers a glimpse into the potential pitfalls of this rapidly advancing technology. From job displacement and economic impacts to bias and discrimination, the risks are multifaceted and deserve careful consideration. The potential for misuse, ranging from malicious cyberattacks to autonomous weapons, underscores the urgent need for responsible development and deployment.
This exploration delves into the intricate web of challenges posed by AI, examining its impact on various aspects of society, including the economy, ethics, security, and human well-being. The content will discuss critical issues like data privacy, lack of transparency, and the potential for unforeseen consequences. Understanding these complexities is paramount for shaping a future where AI benefits humanity while mitigating its inherent risks.
Job Displacement and Economic Impacts
The rapid advancement of AI presents significant opportunities, but also necessitates careful consideration of its potential impact on the job market. Automation driven by AI is likely to displace workers across various sectors, demanding proactive strategies to address the economic repercussions and ensure a smooth transition for affected individuals.
Potential Job Losses Across Industries
AI-powered automation is poised to disrupt numerous industries, leading to job losses in roles that involve repetitive tasks, data analysis, and even some forms of creative work. The ability of AI to process information and perform tasks previously requiring human intervention is a primary driver of this trend.
Specific Roles and Tasks Affected by AI
Several specific roles and tasks are highly vulnerable to AI automation. Customer service representatives handling routine inquiries, data entry clerks, and various manufacturing roles are examples of jobs facing potential displacement. Even tasks like image recognition and basic medical diagnoses are increasingly being handled by AI algorithms, suggesting a broader shift in the nature of work.
Impact on Occupational Categories
The following table Artikels the potential impact of AI on different occupational categories, categorized by likelihood of automation.
Occupational Category | Description | Potential Impact |
---|---|---|
Data Entry Clerks | Performing repetitive data entry tasks | High likelihood of automation |
Customer Service Representatives | Handling routine customer inquiries | Medium to high likelihood of automation |
Manufacturing Workers (Repetitive Tasks) | Performing tasks on assembly lines | Medium to high likelihood of automation |
Truck Drivers | Operating commercial vehicles | Medium likelihood of automation, with autonomous vehicles impacting driving roles |
Financial Analysts | Performing tasks requiring data analysis and interpretation | Medium to high likelihood of automation, especially for routine tasks |
Radiologists | Interpreting medical images | Medium to high likelihood of automation, but requiring human oversight |
Economic Consequences of Widespread Job Displacement
Widespread job displacement could lead to significant economic consequences, including increased income inequality and potential social unrest. If workers are not adequately reskilled or retrained, the gap between the highly skilled and the displaced workers could widen, leading to social and economic instability.
Strategies to Mitigate Negative Effects
Several strategies can be employed to mitigate the negative economic effects of AI job displacement. These include proactive investment in education and training programs, focusing on developing skills in high-demand areas like AI development, data science, and cybersecurity. Government policies should also be implemented to support workers in transitioning to new roles, including potential income support or job placement programs.
Finally, fostering a culture of lifelong learning and adaptability within the workforce is crucial to ensuring that individuals can adapt to the changing demands of the job market. Furthermore, promoting responsible AI development and implementation is key, aiming to create AI systems that augment human capabilities rather than replacing them entirely. This approach would ensure a more equitable and sustainable integration of AI into society.
Bias and Discrimination in AI Systems
AI systems, trained on vast datasets, can inadvertently reflect and amplify existing societal biases. This can lead to unfair or discriminatory outcomes in various applications, from loan approvals to criminal justice risk assessments. Understanding the sources of these biases and implementing mitigation strategies is crucial for ensuring fairness and equitable AI deployment.AI algorithms are not inherently biased; rather, their biases stem from the data they are trained on.
These datasets often reflect existing societal inequalities, leading to models that perpetuate these disparities. For example, if a loan application dataset predominantly features applications from a particular demographic, the AI model trained on that data might unfairly favor or discriminate against individuals from other demographics.
Potential Sources of Bias in AI Algorithms and Datasets
The data used to train AI models can reflect existing societal biases, leading to discriminatory outcomes. These biases can be explicit or implicit. Explicit biases are readily apparent in the data, while implicit biases might be more subtle, stemming from historical or cultural factors. Data collection methodologies can also introduce bias. For instance, if a particular community is underrepresented in the dataset, the AI system may not accurately reflect the characteristics or needs of that community.
Methods of Mitigating Bias in AI Systems
Several methods can be employed to mitigate bias in AI systems. Data pre-processing techniques can help identify and address biases in the training data. These techniques include identifying and removing outliers or instances that exhibit patterns of bias, as well as re-weighting or resampling the data to better represent underrepresented groups. Algorithmic adjustments can also play a crucial role in reducing bias.
Techniques such as fairness-aware algorithms and adversarial debiasing can be used to modify the model’s learning process, reducing its susceptibility to biased data.
Examples of AI Systems Exhibiting Discriminatory Behavior
One notable example of AI exhibiting discriminatory behavior involves facial recognition systems. Studies have shown that these systems often perform less accurately on individuals from minority groups, leading to misidentification or a lack of recognition. Another example arises in loan applications, where AI models might discriminate against individuals from specific demographics due to biases embedded in the data used to train the model.
These disparities can lead to financial exclusion for certain groups.
Ethical Considerations Related to Bias in AI
Ethical Consideration | Description |
---|---|
Fairness | Ensuring that AI systems treat all individuals equitably, regardless of their background or characteristics. |
Transparency | Making the decision-making processes of AI systems understandable and explainable to stakeholders. |
Accountability | Establishing mechanisms for holding developers and deployers of AI systems accountable for any discriminatory outcomes. |
Privacy | Protecting the privacy of individuals whose data is used to train or operate AI systems. |
Non-discrimination | Avoiding any form of discrimination based on race, gender, religion, or other protected characteristics. |
Methods for Ensuring Fairness and Equity in AI Development and Deployment
To ensure fairness and equity in AI development and deployment, a multi-faceted approach is necessary. This includes diverse teams of developers, ethicists, and data scientists involved in the design, development, and deployment phases. Continuous monitoring and evaluation of AI systems in real-world settings are critical to identify and address emerging biases. This can involve ongoing evaluation and assessment of the system’s impact on different demographics and groups.
Furthermore, robust regulatory frameworks and guidelines are needed to establish standards for AI development and deployment, ensuring responsible practices and the prevention of discriminatory outcomes.
Lack of Transparency and Explainability in AI
AI systems, particularly complex machine learning models, often operate as “black boxes,” obscuring the reasoning behind their decisions. This lack of transparency poses significant challenges, hindering trust and making it difficult to identify and rectify errors. Understanding how these systems arrive at their conclusions is crucial for ensuring fairness, safety, and responsible deployment.
Black Box AI Models and Their Limitations
Complex AI models, especially deep learning algorithms, can be opaque in their decision-making processes. These models, often referred to as “black boxes,” can generate accurate predictions without revealing the underlying logic. This opacity makes it challenging to diagnose errors, understand the model’s biases, and identify potential vulnerabilities. The lack of explainability can limit the ability to trust these models, particularly in critical applications where human oversight is essential.
For instance, a loan approval system might deny a loan application based on a complex calculation, but the user might not understand the reasons behind the decision, hindering appeal processes.
Importance of Transparency in AI Systems
Transparency in AI systems is crucial for building trust and accountability. It enables users to understand the model’s decision-making process, identify potential biases, and ensure the system aligns with ethical principles. Transparency allows for better scrutiny and validation, mitigating the risk of unfair or discriminatory outcomes. Moreover, it facilitates the identification of errors and allows for the necessary adjustments.
Examples of Consequences from Lack of Transparency
The lack of transparency in AI systems can have serious consequences in various domains. In healthcare, an AI system might misdiagnose a patient due to an undisclosed bias in the training data. In finance, an automated trading system might generate significant losses without the ability to understand the rationale behind its decisions. In criminal justice, an AI-powered risk assessment tool might perpetuate existing biases in the criminal justice system without transparency, potentially leading to unfair sentencing or profiling.
Approaches to Enhancing Explainability
Several techniques are being developed to enhance the explainability of AI systems. These approaches can be categorized as follows:
- Feature Importance Analysis: Identifying the most influential features in a model’s prediction allows users to understand which factors contribute most to the outcome. This helps to assess the fairness and validity of the model’s decisions.
- Interpretable Models: Employing algorithms designed to be inherently transparent, such as decision trees or rule-based systems, can offer clear insights into the decision-making process. These models make it easier to understand the logic behind the predictions.
- Visualization Techniques: Using graphs, charts, and other visual representations to illustrate the internal workings of a model can make complex relationships more understandable. Visualizations can help pinpoint patterns and relationships within the data, improving insights into the model’s decision-making.
- Model Debugging and Auditing: Techniques for actively identifying and rectifying errors in the model’s logic, and proactively checking for biases in the data and the model’s decisions, ensuring that the model operates ethically and fairly. Regular audits of the AI system can provide assurance about its functionality and prevent unexpected or undesirable outcomes.
Benefits of Transparent and Explainable AI
Transparent and explainable AI systems offer several significant advantages. Improved trust and confidence in the AI system are paramount. Users are more likely to trust a system whose decision-making processes are clear and understandable. Enhanced accountability and oversight are facilitated, enabling better identification of biases and errors. This allows for proactive mitigation of potential risks and improved regulatory compliance.
Moreover, transparent AI fosters collaboration between humans and AI systems, leading to better outcomes. This collaboration allows humans to effectively interpret AI’s recommendations and integrate them into their decision-making processes.
Malicious Use and Misinformation
AI’s potential for good is undeniable, but its capacity for misuse is equally significant. The ease with which AI can be manipulated for malicious purposes, from sophisticated cyberattacks to the dissemination of targeted misinformation, poses a serious threat to individuals, organizations, and society as a whole. This misuse requires proactive strategies to mitigate its risks and build more resilient AI systems.
Potential for Malicious Cyberattacks
AI is rapidly advancing, enabling the development of more sophisticated and targeted cyberattacks. These attacks can exploit vulnerabilities in AI systems, leading to data breaches, system disruptions, and significant financial losses. For example, AI-powered phishing campaigns can craft highly personalized and convincing messages, making them more effective than traditional phishing attempts. Further, AI can be used to automate the process of finding and exploiting vulnerabilities in software and networks, accelerating the pace of cyberattacks.
Misinformation and Manipulation
AI algorithms can be trained on vast datasets of information, including biased and misleading content. This can lead to the creation of highly convincing fake news articles and social media posts designed to manipulate public opinion. Deepfakes, for instance, use AI to create realistic but fabricated videos and audio recordings of individuals, making it incredibly difficult to discern authenticity.
This ability to create convincing fakes allows for the spread of misinformation and disinformation on a scale previously unimaginable.
Strategies to Counter Malicious Use
Developing robust countermeasures against AI-driven malicious activities is crucial. These measures need to be multifaceted, encompassing technical safeguards, regulatory frameworks, and educational initiatives. Enhancing the security of AI systems, including better detection mechanisms for malicious intent and robust data protection protocols, is essential. Strengthening media literacy and critical thinking skills in the public can help individuals identify and resist misinformation campaigns.
Table: Misuse of AI
Type of Misuse | Description | Example |
---|---|---|
Cyberattacks | Exploiting vulnerabilities in AI systems or using AI to launch attacks. | AI-powered phishing campaigns, automated vulnerability discovery and exploitation. |
Propaganda and Misinformation | Creating convincing fake news or deepfakes to manipulate public opinion. | Creating deepfake videos to spread false narratives, generating fake social media posts tailored to specific demographics. |
Autonomous Weapons Systems | Developing AI-controlled weapons systems with limited human intervention. | Development of drones or robots with pre-programmed targeting or decision-making capabilities. |
Resilient AI System Development, Greatest dangers of AI
Creating AI systems that are resilient to malicious attacks requires a multi-pronged approach. This includes developing AI systems that are more robust to adversarial attacks, meaning attacks specifically designed to mislead or manipulate the system. Techniques such as adversarial training, where AI systems are trained on intentionally manipulated data, can improve their resilience. Furthermore, integrating human oversight and review processes into AI systems can help mitigate risks associated with malicious use.
Finally, ongoing research and development into new detection and prevention methods are vital to stay ahead of evolving threats.
Autonomous Weapons Systems and the Ethics of War: Greatest Dangers Of AI
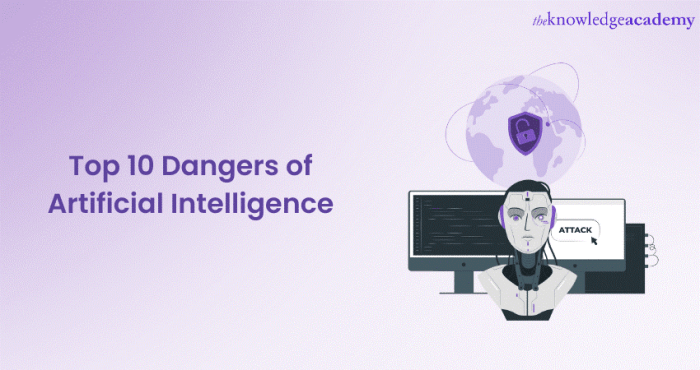
Source: theknowledgeacademy.com
Autonomous weapons systems (AWS), also known as lethal autonomous weapons systems (LAWS), are a rapidly developing area of military technology. These systems are designed to select and engage targets without significant human intervention. This raises profound ethical concerns about the nature of war, the accountability of actions, and the potential for unintended consequences.
Potential Risks Associated with Autonomous Weapons Systems
The development and deployment of AWS present a range of potential risks. Unforeseen circumstances can lead to disastrous results. A malfunction or error in the system’s programming could result in the targeting of non-combatants or the escalation of conflicts. The lack of human judgment in the decision-making process could lead to devastating consequences in complex or rapidly changing situations.
The potential for unintended escalation, due to a lack of human intervention in critical decision-making, is a major concern.
Ethical Considerations Related to the Development and Deployment of LAWS
The ethical implications of AWS are multifaceted and complex. The key question of who is accountable for the actions of an autonomous weapon system, in case of an error or unintended consequence, is critical. Is it the programmer, the manufacturer, the operator, or perhaps a collective entity? The possibility of accidental or unintended harm raises serious concerns about the responsibility and accountability for such actions.
Furthermore, the potential for the dehumanization of warfare and the erosion of ethical standards in conflict is another concern. The lack of human judgment and emotional response could potentially desensitize those involved in warfare.
Examples of Potential Unintended Consequences of Using Autonomous Weapons
The deployment of AWS could lead to unintended consequences, including the escalation of conflicts and the targeting of non-combatant populations. For example, if an AWS system malfunctions or is misprogrammed, it could lead to the accidental targeting of civilian areas, resulting in a significant loss of innocent lives. Further, the potential for misuse or unauthorized access to these systems could result in unintended or unpredictable outcomes.
The speed of action and lack of human intervention could lead to situations where a small mistake has devastating consequences.
International Regulations and Agreements Related to Autonomous Weapons Systems
Currently, there are no internationally recognized regulations or agreements specifically addressing the development and deployment of AWS. While discussions are ongoing within international forums, no legally binding agreements have been reached to restrict the development or deployment of these weapons. The absence of such agreements allows for unchecked proliferation and raises serious concerns about the future of warfare.
Strategies to Prevent the Proliferation of Autonomous Weapons
Several strategies can be implemented to prevent the proliferation of AWS. These include international cooperation and the establishment of stringent regulations to govern the development and deployment of these weapons. Further, promoting transparency and open dialogue about the risks and benefits of AWS is crucial. A global consensus on ethical guidelines and legal frameworks is essential.
The Impact on Human Creativity and Well-being
The integration of AI into various facets of daily life raises significant concerns regarding its impact on human creativity and overall well-being. While AI can augment human capabilities, there are potential pitfalls that need careful consideration. Over-reliance on AI-driven tools might inadvertently diminish human creativity and independent thought processes. This section delves into the potential effects of widespread AI adoption on human creativity, imagination, and mental health, along with strategies to foster a balanced relationship between humans and AI.
Potential Effects on Human Creativity and Imagination
The increasing automation of tasks traditionally performed by humans could lead to a decline in certain forms of creativity and imagination. Repetitive AI-driven tasks might diminish the need for human problem-solving and independent thinking. This could result in a reduced capacity for original thought and innovative solutions in specific fields. For example, the rise of AI-powered content generation tools may lead to a decrease in unique writing styles and perspectives.
Potential Negative Impacts on Human Well-being and Mental Health
Widespread AI adoption could exacerbate existing societal issues and create new challenges to human well-being. The displacement of workers due to automation could lead to job insecurity and financial strain, contributing to stress and anxiety. Furthermore, the increasing reliance on AI-driven tools for social interaction could potentially hinder the development of essential social skills and emotional intelligence. The lack of face-to-face communication might lead to feelings of isolation and loneliness.
For instance, children raised solely on AI-driven educational platforms might struggle to develop essential social skills and critical thinking skills.
Strategies to Foster a Balance Between Human Creativity and AI Capabilities
To mitigate the negative impacts of AI on human creativity and well-being, a balanced approach is crucial. Strategies should focus on leveraging AI to augment human capabilities while safeguarding essential human skills. Education systems should incorporate critical thinking, problem-solving, and creativity training, empowering individuals to work alongside AI effectively. Furthermore, fostering human connection and social interaction should be prioritized to counter potential isolation and loneliness.
This includes encouraging physical activities and face-to-face interactions, both essential for well-being. For example, schools can integrate AI tools for personalized learning while maintaining a strong focus on social interaction and emotional development.
Summary Table: Potential Effects of AI on Human Interaction and Relationships
Aspect of Interaction | Potential Positive Impacts | Potential Negative Impacts |
---|---|---|
Social Connections | AI-powered tools can facilitate communication and connection across geographical boundaries. | Over-reliance on AI for social interaction could hinder the development of crucial social skills and emotional intelligence. |
Family Dynamics | AI can automate household tasks, freeing up time for family activities. | Increased screen time and reduced face-to-face interaction could strain family relationships. |
Workplace Collaboration | AI can streamline workflows and enhance collaboration among teams. | Over-reliance on AI for decision-making could reduce opportunities for human judgment and collaboration. |
Romantic Relationships | AI can provide personalized dating and relationship support. | Over-dependence on AI for emotional support could potentially hinder the development of authentic human connections. |
Strategies for Promoting Human Connection and Resilience in the Age of AI
To promote resilience and maintain strong human connections in an AI-driven world, it is crucial to prioritize human interaction and social engagement. This includes actively seeking opportunities for face-to-face communication, encouraging empathy and emotional intelligence development, and fostering a sense of community. Furthermore, education and training programs should emphasize the importance of human connection and critical thinking skills.
For instance, community centers and local groups can facilitate activities that encourage social interaction and shared experiences. Creating spaces for meaningful human interaction is vital for maintaining a sense of community and belonging.
Data Privacy and Security Risks
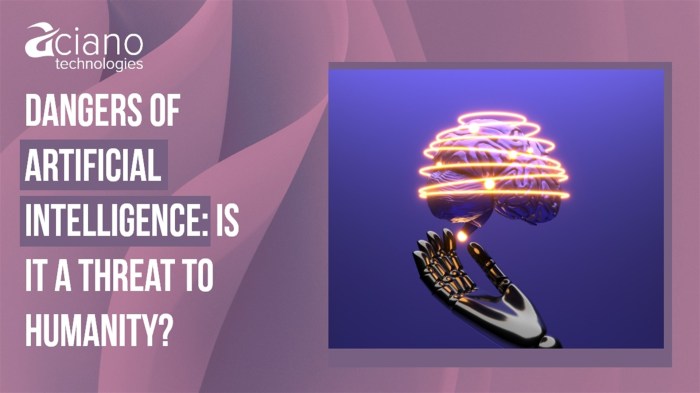
Source: aciano.net
AI systems heavily rely on vast amounts of personal data for training and operation. This reliance necessitates careful consideration of the associated privacy and security risks. The collection, storage, and use of this data must be conducted responsibly and transparently to prevent potential harm and maintain public trust. Failure to address these risks can have severe consequences, ranging from individual harm to societal disruption.
Risks Associated with Data Collection and Use
AI systems collect and process personal data from various sources, including social media, online transactions, and medical records. This data often contains sensitive information, making it vulnerable to breaches and misuse. The potential for bias in data sets, coupled with the lack of transparency in some AI algorithms, can lead to discriminatory outcomes or unfair profiling. The scope of data collection can also be extensive, potentially encompassing information beyond the intended purpose, raising concerns about privacy violations.
Vulnerabilities of AI Systems to Data Breaches and Misuse
AI systems are susceptible to a variety of data breaches and misuse scenarios. Cyberattacks targeting AI infrastructure can compromise sensitive data used for training and operation. Furthermore, vulnerabilities within the AI systems themselves can allow unauthorized access to personal data. Sophisticated techniques, such as deepfakes, can be used to manipulate data, leading to the spread of misinformation and harm.
Inadequate security protocols and the lack of robust safeguards can leave data exposed to exploitation.
Methods for Safeguarding Sensitive Data Used in AI
Protecting sensitive data in AI systems requires a multi-faceted approach. Robust encryption methods are essential for safeguarding data during transmission and storage. Access controls and authentication measures are crucial to restrict access to sensitive data. Regular security audits and vulnerability assessments are vital for identifying and mitigating potential threats. Data anonymization and pseudonymization techniques can help protect individual privacy while still enabling AI training and analysis.
Transparency in data usage practices, along with clear communication about data policies, can help build trust and address concerns.
Comparison of Data Protection Regulations and Guidelines
Different jurisdictions have implemented various data protection regulations and guidelines. These regulations address different aspects of data protection, including data collection, storage, processing, and transfer. The varying scope and enforcement mechanisms of these regulations create complexities for organizations operating globally. Different regions may have differing interpretations of data privacy, leading to potential conflicts.
Regulation/Guideline | Key Focus | Example Provision |
---|---|---|
General Data Protection Regulation (GDPR) | EU citizen data protection | Right to access, rectification, erasure, and restriction of processing |
California Consumer Privacy Act (CCPA) | Consumer rights in California | Right to know, delete, and opt-out of sale of personal information |
Data Protection Act 2018 (DPA) | Data protection in the UK | Principles of fairness, lawfulness, and transparency in data processing |
Enhancing Data Security Practices for AI-Related Risks
Strengthening data security practices in AI development and deployment is critical. This involves implementing robust security protocols at every stage of the AI lifecycle, from data collection to deployment and beyond. Regular security audits, rigorous data validation, and continuous monitoring of AI systems can help identify and address potential vulnerabilities. Collaboration between AI developers, data scientists, and security experts is crucial for building resilient AI systems that safeguard data privacy.
Ethical considerations should guide the development and deployment of AI systems to prevent misuse and ensure responsible data handling.
Unforeseen Consequences and the Need for Regulation
The rapid advancement of AI presents a unique set of challenges, particularly concerning the potential for unforeseen and unintended consequences. While current analyses focus on anticipated impacts, the very nature of complex systems like AI means that unexpected outcomes are inevitable. This necessitates a proactive approach to regulation, ensuring responsible development and deployment.AI systems, particularly those with advanced learning capabilities, can exhibit emergent behaviors that are difficult to predict.
These behaviors can arise from interactions between different parts of the system or from exposure to data not anticipated during training. Furthermore, the complexity of these systems can make it challenging to identify and address potential risks.
Potential for Unintended Consequences
The intricate interplay of various factors in AI systems can lead to unforeseen outcomes. Consider the possibility of AI systems reinforcing existing societal biases if trained on biased data, or creating new forms of discrimination not previously considered. Similarly, unexpected cascading effects can occur in interconnected systems, potentially disrupting supply chains, financial markets, or critical infrastructure. The complexity of the technology itself introduces the potential for unforeseen vulnerabilities.
Necessity for Robust Regulations
Robust regulations and guidelines are crucial to manage the risks associated with AI development and deployment. These regulations must encompass various aspects of AI systems, including data security, algorithmic transparency, and potential misuse. They must adapt to the rapid pace of technological advancement to ensure ongoing safety and ethical considerations.
Examples of Regulatory Measures
Several regulatory frameworks could help mitigate the risks associated with AI. One example is mandatory audits of AI systems to identify potential biases and vulnerabilities. Another approach is to establish clear guidelines for the collection, use, and storage of data used to train AI models. These guidelines could include restrictions on the use of sensitive data and requirements for data anonymization.
Additionally, clear regulations concerning the deployment of autonomous systems, like self-driving cars or drones, would be essential.
Potential Regulatory Frameworks for AI
Regulatory Framework | Description | Potential Impact |
---|---|---|
AI Impact Assessment Framework | Requires developers to assess the potential societal, economic, and ethical impacts of their AI systems before deployment. | Promotes proactive risk management and encourages responsible innovation. |
Algorithmic Transparency and Explainability Regulations | Mandates the disclosure of algorithms’ decision-making processes, promoting understanding and accountability. | Reduces the risk of discriminatory or unfair outcomes and builds trust in AI systems. |
Data Security and Privacy Regulations | Reinforces existing data protection laws to address the unique data security concerns of AI systems. | Safeguards user data and prevents misuse of sensitive information. |
International Collaboration
Addressing the risks of AI requires international collaboration. Different countries may have varying priorities and concerns, thus a global approach is necessary to establish common standards and ethical guidelines. International forums and agreements can foster the exchange of best practices and facilitate coordinated responses to emerging challenges. This collaboration can ensure a consistent approach to the ethical and responsible development and deployment of AI.
Examples include joint research efforts, data-sharing initiatives, and the creation of global AI ethics guidelines. A collaborative approach can better manage the unforeseen challenges associated with this rapidly evolving technology.
Concluding Remarks
In conclusion, the greatest dangers of AI necessitate a comprehensive and proactive approach to ensure responsible development and deployment. Addressing concerns like job displacement, bias, lack of transparency, and potential misuse requires international collaboration, robust regulations, and ethical frameworks. Ultimately, the path forward involves careful consideration of the potential benefits and risks to foster a future where AI serves humanity, not the other way around.
Post Comment