Google Scholar vs AI Search Tools A Comparison
Google Scholar vs AI search tools are revolutionizing academic research. Traditional methods face challenges in keeping pace with the volume and complexity of information. This exploration delves into the features, limitations, and user experiences of both, examining how AI-powered search tools might enhance or replace Google Scholar in the future.
The comparison examines indexing methodologies, data accuracy, and potential biases inherent in each approach. It analyzes strengths and weaknesses of Google Scholar, contrasting it with emerging AI tools for academic research. Ultimately, the analysis aims to provide researchers with a comprehensive understanding of these tools, empowering them to make informed decisions about which approach best suits their specific needs and research questions.
Introduction to Academic Search
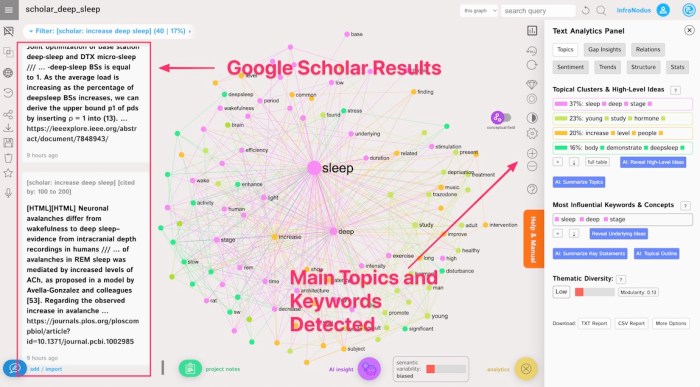
Source: noduslabs.com
Academic research forms the bedrock of knowledge advancement across disciplines. It involves rigorous investigation, analysis, and critical evaluation of existing theories and evidence to generate new insights and understanding. The need for efficient tools to navigate this vast body of research is paramount, allowing scholars to quickly and effectively access relevant information for their studies.The historical context of academic search is marked by significant evolution.
Initially, researchers relied on library catalogs, physical journals, and laborious manual methods to locate pertinent material. The advent of digital technologies and the internet revolutionized academic research, enabling researchers to access a global repository of information through online databases and search engines. This shift has led to a greater demand for sophisticated search tools capable of filtering and retrieving relevant information from the vast digital landscape.
General Search Engines vs. Specialized Academic Search Engines
General search engines, like Google, index a broad spectrum of content, encompassing websites, news articles, and blogs, in addition to academic publications. Specialized academic search engines, such as Google Scholar, are specifically designed to index and retrieve scholarly articles, research papers, theses, and other academic resources. The crucial distinction lies in the focus and the quality of the indexed material.
General search engines often return a mixture of high-quality and less-credible content, while academic search engines prioritize peer-reviewed publications and scholarly works.
Comparison of Features and Functionalities
Feature | General Search Engines (e.g., Google) | Academic Search Engines (e.g., Google Scholar) |
---|---|---|
Content Focus | Broad range of content, including websites, news articles, and blogs. | Primarily scholarly articles, research papers, theses, and other academic resources. |
Indexing Methodology | Based on web crawling and indexing of web pages. | Focuses on indexing academic databases and repositories. |
Citation Tracking | Limited or no direct citation tracking. | Typically includes citation information, enabling researchers to trace the influence of a particular work. |
Peer Review Filtering | No explicit filtering for peer-reviewed content. | Often prioritizes or displays peer-reviewed articles more prominently. |
Search Refinements | Limited search options for specific academic disciplines or publication types. | Advanced search options to filter by discipline, publication date, author, and other relevant criteria. |
Accuracy and Reliability | Content quality varies greatly. | Prioritizes and often displays more credible and reliable scholarly resources. |
Google Scholar
Google Scholar, a prominent online search engine, serves as a valuable resource for researchers seeking academic literature. It aims to provide a comprehensive collection of scholarly articles, books, theses, and other academic works. However, its effectiveness in the academic research landscape is not without limitations.Google Scholar’s primary strength lies in its vast coverage of academic material. Its comprehensive indexing allows users to quickly locate relevant resources across diverse disciplines.
Despite this strength, limitations exist in terms of the types of resources indexed, the accuracy of results, and the complexity of certain searches. Understanding these strengths and weaknesses is crucial for effective academic research.
Core Functionalities
Google Scholar’s core functionalities include indexing and searching academic publications from various sources. Its ability to identify relevant content across different disciplines makes it a quick initial search tool. The strength lies in its broad coverage, while its weakness is in the depth of analysis or nuanced search capabilities.
Indexing and Retrieval Methods
Google Scholar employs a complex indexing process to retrieve academic resources. It crawls various sources, including university websites, research repositories, and publishers’ databases. This method ensures a wide range of publications are accessible, but can lead to inconsistencies in the quality and accuracy of the indexed information. The indexing process is not always perfect, and outdated or inaccurate information might be included.
Effective and Ineffective Retrieval Examples
Google Scholar effectively retrieves readily available articles and books. It’s strong at providing citations and basic metadata for many research outputs. However, it struggles with specialized, newly published, or less accessible materials. For example, conference proceedings or grey literature might be incompletely indexed, or not present at all. Further, the results often need filtering and evaluation to ensure accuracy and relevance.
Strengths and Weaknesses of Google Scholar
Search Type | Strength | Weakness |
---|---|---|
General Search | Quickly identifies relevant articles across disciplines. | May return numerous irrelevant results requiring extensive filtering. |
Citation-Based Search | Efficiently finds related publications. | May miss newer or less cited publications in certain fields. |
Source Type | Indexes a wide range of academic publications (journals, books, etc.). | Quality of indexing varies across sources, leading to discrepancies in the retrieved information. |
Citation Information | Provides basic citation details. | Citation information may be incomplete or inaccurate, requiring manual verification. |
AI Search Tools
AI-powered search tools are rapidly transforming the landscape of academic research, offering new ways to access and process information. These tools leverage machine learning algorithms to analyze vast datasets, identifying patterns and connections that might be missed by traditional search methods. This allows researchers to uncover relevant information more efficiently and gain deeper insights into complex topics.These tools are still under development, but their potential to revolutionize research is significant.
Early adopters are reporting improved efficiency and effectiveness in their research endeavors. However, it is crucial to be aware of both the potential benefits and limitations of this emerging technology.
Emerging AI-Powered Search Tools
A variety of AI-powered search tools are emerging, each with its unique approach to information retrieval. These tools are designed to sift through massive academic databases, identify key concepts, and provide summaries of research articles. Examples include tools integrated with existing research platforms, as well as standalone applications designed specifically for academic search.
Potential Benefits of AI in Academic Research
AI search tools can significantly enhance academic research in several ways. Improved efficiency in information retrieval is a key benefit. Researchers can quickly identify relevant articles and resources, saving valuable time. AI can also help researchers understand complex relationships between different concepts, leading to more insightful analysis and potentially leading to novel discoveries. Moreover, AI can provide personalized recommendations based on a researcher’s specific interests and needs, enabling a more targeted and efficient research process.
Potential Limitations of AI in Academic Research
While AI offers significant potential, it’s essential to acknowledge its limitations. AI search tools are trained on existing data, and their ability to understand nuances in academic language or identify emerging trends may be limited. Bias in the training data can also lead to skewed results. Furthermore, the interpretation of the results generated by these tools requires critical evaluation by the researcher.
Researchers should not solely rely on AI for analysis; their own expertise and critical thinking are still crucial.
Indexing Methods of AI Search Tools
AI search tools employ various indexing methods to organize and retrieve information. These methods often combine traditional -based indexing with more sophisticated techniques. Natural language processing (NLP) allows the tools to understand the context and meaning of words and phrases within research papers. This enables the tools to identify relevant documents even if they do not contain the exact s used in the search query.
Machine learning algorithms are often employed to identify patterns and relationships between different concepts, leading to more accurate and comprehensive search results.
Comparison of Indexing Methods
Different AI search tools utilize varying indexing strategies, each with its strengths and weaknesses. Some tools might excel at identifying specific s but struggle with contextual understanding. Others might be more effective at uncovering relationships between concepts but less accurate in identifying relevant documents based on specific s. The effectiveness of each tool depends heavily on the specific dataset it was trained on and the nature of the search query.
Ultimately, a researcher should be familiar with the strengths and weaknesses of different tools to select the most appropriate one for their research needs.
Comparative Analysis
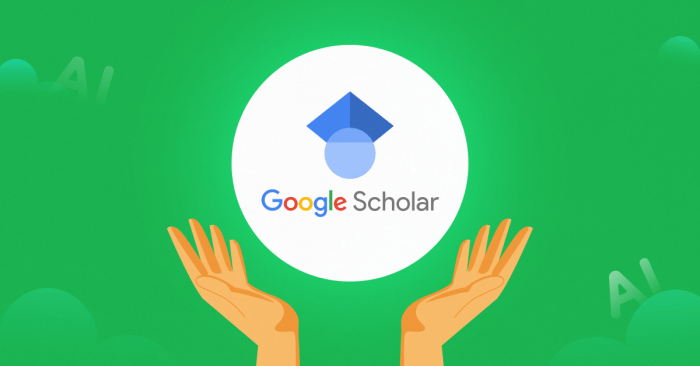
Source: guvi.in
Google Scholar and AI-powered search tools represent distinct approaches to academic research. Google Scholar, a widely used resource, relies on indexing existing publications. AI tools, conversely, leverage sophisticated algorithms and machine learning to analyze vast datasets and identify relevant information in novel ways. This comparison highlights the strengths and weaknesses of each approach, demonstrating when each tool excels.Both Google Scholar and AI tools have their place in the academic research landscape.
Google Scholar remains a valuable resource for readily available scholarly articles, while AI tools are increasingly used for more complex research tasks requiring analysis and synthesis of information from various sources. Understanding the nuances of each tool is crucial for researchers to optimize their research process.
Strengths and Weaknesses of Google Scholar
Google Scholar is a robust resource for locating publicly available academic papers. Its strength lies in its comprehensive indexing of research articles, conference proceedings, and other scholarly works. However, Google Scholar’s approach is fundamentally based on matching and citation analysis. This can lead to a lack of nuanced understanding of complex research topics and a potential over-reliance on popular or easily accessible publications.
Strengths and Weaknesses of AI Search Tools
AI search tools are emerging as powerful alternatives or complements to traditional methods. Their strengths include advanced natural language processing (NLP) capabilities, enabling the analysis of complex research questions and the identification of subtle connections between different research papers. However, the accuracy of AI search tools is dependent on the quality and comprehensiveness of the data they are trained on, and these tools may lack the historical context and depth of understanding that a seasoned researcher brings to the table.
Specific Use Cases
Google Scholar excels in tasks requiring a broad overview of existing literature on a given topic. It’s ideal for identifying foundational works, establishing background knowledge, and finding specific articles based on s. AI tools, in contrast, are more effective in tasks requiring in-depth analysis, synthesis of information, and identification of patterns or relationships across various sources. These tools are particularly valuable for literature reviews, identifying emerging trends, and creating summaries of complex topics.
Examples of AI Search Tools Improving upon Google Scholar, Google Scholar vs AI search tools
AI search tools can improve upon Google Scholar by providing more nuanced and comprehensive search results. For example, instead of simply returning a list of articles matching s, an AI tool can identify related concepts, suggest relevant research questions, and even provide summaries or critiques of the research. Some AI search tools can even predict future research trends based on current patterns in the literature.
Comparative Table
Feature | Google Scholar | AI Search Tool 1 (e.g., CiteSeerX) | AI Search Tool 2 (e.g., Semantic Scholar) |
---|---|---|---|
Ease of Use | High | Medium | Medium-High |
Depth of Analysis | Low | Medium | High |
Synthesis of Information | Limited | Moderate | High |
Identifying Emerging Trends | Limited | Moderate | High |
User Needs (Basic Research) | Excellent | Good | Excellent |
User Needs (Complex Research) | Fair | Excellent | Excellent |
Task Complexity (Literature Review) | Moderate | High | High |
User Experience and Interface
The user experience (UX) and interface design significantly impact how researchers interact with academic search tools. A well-designed interface can streamline the search process, making it easier to find relevant information. Conversely, a poorly designed interface can lead to frustration and wasted time. This section explores the UX and interface elements of Google Scholar and AI-powered search tools, comparing their effectiveness and ease of use.
Google Scholar Interface
Google Scholar employs a straightforward, familiar interface, mirroring the design of other Google products. Its simplicity is often lauded for its ease of use. Users can easily input s, refine searches with advanced operators, and browse results. The presentation of results is clear, highlighting key information like author, publication date, and journal. A strength lies in the seamless integration with other Google services, which can be helpful for researchers already familiar with the Google ecosystem.
However, it may lack some of the sophisticated filtering options found in specialized databases.
AI-Powered Search Tool Interface
AI-powered search tools are increasingly incorporating innovative interface designs. These tools often leverage natural language processing (NLP) to allow for more conversational and intuitive queries. Some platforms incorporate visual aids and interactive elements to present search results, such as diagrams or timelines. The potential of these tools lies in their ability to anticipate user needs and provide more contextually relevant results.
However, the complexity of these interfaces can sometimes lead to a steeper learning curve for users not accustomed to AI-driven interactions.
Comparison of Search Interfaces
The effectiveness of a search interface hinges on the specific needs of the researcher. Google Scholar’s straightforward design suits users familiar with its platform. AI-powered tools offer the promise of more nuanced searches, but may require users to adjust to a new way of interacting with the search process.
Interface Element Comparison
Feature | Google Scholar | Example AI Search Tool (Hypothetical) |
---|---|---|
Search Input | Simple text box for s and operators | Advanced search bar, incorporating natural language processing; allows for more nuanced queries with context and intent recognition. |
Result Display | Clear presentation of author, publication date, and journal. | Interactive result display; includes visual aids (e.g., timelines, diagrams) to contextualize the results and enhance understanding. |
Refinement Options | Basic filtering (e.g., publication date, file type) | Advanced filtering based on AI-driven analysis of the content (e.g., specific concepts, research methodologies, author expertise). |
Navigation | Simple navigation; typical web-page structure. | Intuitive navigation; user experience designed for ease of exploration of complex topics, including interlinked topics and related concepts. |
Data Accuracy and Bias: Google Scholar Vs AI Search Tools
The accuracy and reliability of search results are paramount in academic research. However, both Google Scholar and AI search tools are susceptible to biases inherent in their underlying datasets and algorithms. Understanding these biases is crucial for researchers to critically evaluate search results and ensure that their conclusions are not skewed by problematic data representations.
Potential Biases in Datasets
The datasets used by Google Scholar and AI search tools often reflect existing societal biases. These biases can manifest in various forms, such as uneven representation of different research areas, geographic locations, or academic institutions. For example, a dataset predominantly populated by research from Western nations might underrepresent studies from developing countries, potentially leading to a skewed understanding of global phenomena.
Similarly, research from specific institutions might be disproportionately highlighted, giving them an undue prominence in search results. Understanding the origins and characteristics of the datasets is essential for recognizing their potential for bias.
Bias in Search Algorithm
The algorithms used by these tools further amplify potential biases present in the underlying data. Algorithms, designed to prioritize certain information or rank results based on specific criteria, can inadvertently perpetuate existing biases. For example, algorithms that emphasize citations or publication dates might disproportionately favour established researchers or research published in prestigious journals. Consequently, emerging research from lesser-known institutions or newer fields might be overlooked.
This phenomenon can be especially concerning in emerging fields or interdisciplinary research, as it might hinder the discovery of novel perspectives or approaches.
Bias in User Input
User input, although less directly related to the tool itself, can also influence search results and introduce biases. Researchers’ own implicit biases can affect the s they use, leading to a skewed set of results that reflects their pre-existing notions. Similarly, the phrasing of a search query might implicitly favor certain viewpoints or perspectives, leading to a biased presentation of information.
These biases are not inherent to the tools themselves, but rather represent the human element in the research process, which researchers must actively strive to mitigate.
Comparative Analysis of Data Accuracy and Reliability
While both Google Scholar and AI search tools strive for accuracy, their methodologies differ, impacting their reliability. Google Scholar relies primarily on citations and publication metadata, while AI search tools use more complex algorithms that analyze text and other data points. The accuracy and reliability of AI search tools depend heavily on the quality and representativeness of their training data.
Google Scholar, while potentially less sophisticated in its approach, provides a broader overview of published research, potentially offering a more comprehensive, if less nuanced, understanding of the research landscape.
Potential Implications of Biases in Search Results
Biased search results can lead to inaccurate conclusions and misrepresentations of the research landscape. Researchers relying on these tools might overlook important perspectives or critical counterarguments. Furthermore, the propagation of biased results can reinforce existing stereotypes or create new ones, ultimately hindering the advancement of knowledge. Researchers should always critically assess the results and seek to validate the information from multiple sources to mitigate the risks of biased interpretations.
Potential Sources of Bias
Source of Bias | Google Scholar | AI Search Tools |
---|---|---|
Data Source | Uneven geographic representation, skewed citation patterns, dominance of Western scholarship. | Bias reflected in the training data, potentially skewed representation of specific research areas, geographic or institutional biases. |
Algorithm | Emphasis on citations and publication dates might favour established researchers and institutions. | Algorithm prioritization of certain s or text patterns might reinforce existing biases in the data. Potential for bias in the ranking algorithm. |
User Input | Researcher’s own implicit biases influence search queries. | Researcher’s implicit biases influence search queries. The phrasing of a search query might implicitly favor certain perspectives. |
Future Trends and Implications
The landscape of academic research is undergoing a rapid transformation, driven by the evolution of search technologies. The interplay between traditional academic search tools like Google Scholar and emerging AI-powered alternatives is creating a dynamic environment that promises both significant opportunities and challenges for researchers. Understanding these trends and implications is crucial for navigating the future of scholarly discovery.The future of academic search tools will likely be shaped by advancements in AI, particularly in natural language processing (NLP).
This technology allows for more sophisticated understanding and interpretation of research queries, enabling more precise and relevant results. Furthermore, the integration of machine learning algorithms will likely enhance the ability of these tools to identify emerging trends and patterns within the vast body of academic literature.
Future Development of Academic Search Tools
AI-powered search tools are expected to become increasingly sophisticated, incorporating advanced NLP techniques. This will result in more nuanced search capabilities, allowing researchers to extract more precise information from complex texts. The tools will also likely become more personalized, adapting to individual research styles and preferences. Real-world examples of this personalization can be observed in popular search engines like Google, which adapt search results based on user history and preferences.
Potential Impact of AI on the Academic Research Landscape
AI’s influence on academic research is multifaceted. It has the potential to significantly accelerate the research process by automating tasks such as literature review and data analysis. This automation could free researchers to focus on higher-level tasks, like interpretation and synthesis. The increased efficiency and accessibility of research information can foster collaboration among researchers, bridging geographical and institutional barriers.
An example of this is seen in the use of AI-powered tools to analyze large datasets, which allows researchers to identify patterns and insights that might otherwise be missed.
Summary of Trends in Academic Search Tool Development
Several key trends are emerging in the development of academic search tools. A central trend is the increasing use of AI-powered functionalities. This encompasses improved search accuracy, personalized recommendations, and automated analysis of research findings. Another trend is the move towards greater integration with other academic resources and platforms. This includes providing links to related databases, journals, and repositories.
Furthermore, a focus on enhanced user experience, with intuitive interfaces and seamless navigation, is becoming increasingly important. This can be seen through the evolution of user interfaces from simple searches to more sophisticated, query-based systems.
Examples of AI Integration Enhancing Research
The integration of AI in search tools can significantly enhance the research process in several ways. One example is the use of AI to automatically summarize research articles. This allows researchers to quickly grasp the core arguments and findings of numerous publications. Furthermore, AI can identify relevant research papers based on intricate relationships between concepts, going beyond simple matches.
Another example is the ability to analyze research papers for biases in methodology or assumptions. This is especially useful for critical analysis and understanding the limitations of the research.
End of Discussion
In conclusion, Google Scholar and AI search tools represent distinct approaches to academic research. While Google Scholar remains a valuable resource for its broad reach and established indexing, AI search tools offer innovative solutions with potential to streamline research and improve user experience. The future likely involves a combination of these tools, with AI tools augmenting Google Scholar’s capabilities.
Further development and refinement of AI tools will likely be crucial for optimizing their accuracy, addressing potential biases, and enhancing the user experience to facilitate more efficient academic research.
Post Comment