Comparing AI Deep Research Tools Kompas AI vs Elicit
Comparing AI deep research tools Kompas AI Elicit delves into the capabilities and limitations of these cutting-edge platforms. This exploration examines their core functionalities, specific deep research features, and comparative analysis across various use cases, from natural language processing to image recognition. The discussion will also cover data handling, model architecture, and potential future trends in AI deep research tools.
The comparison will provide a clear understanding of the strengths and weaknesses of each tool, enabling users to make informed decisions based on their specific research needs. A detailed look at illustrative use cases, time efficiency, and resource requirements will solidify the comparison.
Introduction to AI Deep Research Tools
AI deep research tools leverage artificial intelligence to analyze and interpret vast datasets, accelerating the research process and uncovering insights that might be missed by traditional methods. These tools are increasingly important across diverse fields, from scientific discovery to business intelligence. Their capabilities range from automating data collection and analysis to generating novel hypotheses and predictions.These tools typically utilize machine learning models to identify patterns, relationships, and anomalies in complex data.
This automated approach can significantly reduce the time and resources required for in-depth research, allowing researchers to focus on the interpretation and application of the findings. The potential for these tools to revolutionize various fields is substantial.
Common Functionalities
AI deep research tools share several key functionalities. These tools often automate data collection from various sources, including academic databases, news articles, and social media. They can also preprocess data, cleaning and transforming it into a format suitable for analysis. A critical function is pattern recognition, where algorithms identify complex relationships and correlations within the data. Advanced tools also allow for the generation of hypotheses and predictions based on the discovered patterns.
Finally, many offer visualization capabilities to present findings in an easily understandable format.
Potential Applications
AI deep research tools have a wide range of potential applications across various sectors. In scientific research, these tools can accelerate the discovery of new medicines, materials, and phenomena. In business, they can help identify market trends, predict consumer behavior, and personalize customer experiences. Furthermore, AI deep research tools are being used in finance to detect fraud, in healthcare to diagnose diseases, and in social sciences to understand complex societal dynamics.
Comparison of AI Deep Research Tools
Tool Name | Data Type Support | Model Type | Supported Tasks |
---|---|---|---|
Kompas AI | Text, structured data, images | Transformer-based models, neural networks | Literature review, hypothesis generation, knowledge graph construction |
Elicit | Text, code, structured data | Large language models, retrieval models | Question answering, summarization, code generation, and extraction of knowledge from various sources |
Other AI Research Tool 1 | Text, tabular data, scientific publications | Neural networks, machine learning models | Predictive modeling, data analysis, pattern recognition |
Other AI Research Tool 2 | Text, audio, video | Deep learning models, natural language processing models | Sentiment analysis, topic modeling, content categorization |
This table provides a basic overview of different tools and their capabilities. The specific functionalities and supported tasks may vary considerably between different tools. Data type support, model type, and supported tasks should be carefully considered when selecting a tool for a particular research project.
Kompas AI Deep Research Capabilities
Kompas AI stands out as a powerful tool for deep research, offering a suite of features designed to streamline and enhance the analytical process. Its capabilities extend beyond basic data analysis, enabling researchers to delve into complex datasets and uncover intricate patterns and insights.Kompas AI’s core functionalities revolve around its ability to process and interpret vast quantities of data, enabling researchers to accelerate their investigations.
This tool is particularly well-suited for tackling complex research questions in various domains, providing a comprehensive solution for data-driven inquiries.
Core Functionalities of Kompas AI
Kompas AI’s core capabilities encompass several key functionalities. These include data preprocessing, feature engineering, model training, and result interpretation. Efficient data preprocessing allows for the removal of irrelevant information and the preparation of data for advanced analysis. Feature engineering, a crucial aspect of deep learning, facilitates the creation of new, insightful features from existing data. Furthermore, Kompas AI supports the training of various deep learning models, including neural networks, allowing for the extraction of complex relationships and patterns within data.
Finally, its intuitive interface facilitates the interpretation of results, enabling researchers to understand and contextualize the findings in the context of their research.
Deep Research Features
Kompas AI provides specialized features for deep research, allowing users to tackle sophisticated tasks. These features include advanced data visualization, allowing researchers to explore intricate data relationships and trends. Furthermore, Kompas AI facilitates the integration of external data sources, enriching the dataset and enabling researchers to investigate wider contexts. Specific support for natural language processing (NLP) enables the analysis of text-based data, providing researchers with a powerful tool to extract meaning and insights from large text corpora.
Kompas AI also offers advanced algorithms for anomaly detection, enabling researchers to identify unusual patterns or outliers in data.
Examples of Complex Research Tasks
Kompas AI can be applied to various complex research tasks across diverse fields. For instance, in medical research, it can analyze patient data to identify potential risk factors for diseases or predict treatment outcomes. In financial analysis, Kompas AI can be used to predict market trends or identify fraudulent activities. In social science research, it can be used to analyze large-scale social media data to understand public opinion or trends.
These are just a few examples, highlighting the versatility and wide-ranging applications of Kompas AI.
Strengths and Weaknesses of Kompas AI’s Deep Learning Capabilities
Feature | Strength | Weakness |
---|---|---|
Data Processing Speed | Kompas AI excels at processing large datasets, enabling rapid analysis of complex information. | The processing speed might be constrained by the size of the dataset or the complexity of the model in some specific cases. |
Model Training Efficiency | Kompas AI facilitates the training of various deep learning models with user-friendly interfaces. | The tool’s efficiency might vary depending on the specific model architecture and computational resources available. |
Feature Engineering Capabilities | Kompas AI supports the creation of new, insightful features from existing data, leading to improved model performance. | Feature engineering might require domain expertise to optimize the process. |
Integration with External Data Sources | Integration with external data sources enables researchers to investigate wider contexts and explore more comprehensive insights. | The complexity of integrating different data formats and sources might pose some challenges. |
Elicit Deep Research Capabilities
Elicit stands out as a powerful AI tool for deep research, particularly in areas demanding intricate analysis of complex datasets. Its capabilities extend beyond simple data processing, enabling users to uncover nuanced insights and patterns that might otherwise remain hidden. This section will delve into Elicit’s core functionalities, highlighting its unique strengths in deep research.
Core Functionalities of Elicit
Elicit’s core functionalities revolve around its deep learning architecture, enabling advanced data processing and analysis. It excels at extracting meaningful information from diverse data sources, including structured and unstructured formats. Elicit’s proficiency in handling large volumes of data is crucial for complex research endeavors.
Specific Features Related to Deep Research
Elicit possesses several specific features that are particularly valuable for deep research. These features include sophisticated natural language processing (NLP) capabilities, enabling nuanced understanding of textual data. Elicit also offers advanced data visualization tools, making complex findings accessible and understandable. Furthermore, its adaptability to various data formats ensures seamless integration with diverse research workflows.
Examples of Elicit Use in Complex Research Tasks
Elicit’s capabilities can be applied to a wide range of complex research tasks. For instance, in market research, Elicit can analyze vast amounts of customer feedback to identify emerging trends and predict future market shifts. In scientific research, Elicit can analyze intricate biological data to identify patterns in gene expression and predict disease outcomes. Elicit can also be used to analyze social media data to understand public sentiment towards specific products or events.
Unique Strengths of Elicit’s Deep Learning Approach
Elicit’s deep learning approach offers several unique strengths compared to other tools. Firstly, its ability to learn complex patterns from data without explicit programming significantly accelerates the research process. Secondly, its adaptable architecture allows researchers to tailor the model to their specific needs, creating custom solutions for unique research objectives. Thirdly, Elicit’s ongoing learning capabilities enable continuous improvement and refinement of results, providing a dynamic and evolving research platform.
Comparison of Data Handling Between Kompas AI and Elicit
This table Artikels the key differences in data handling between Kompas AI and Elicit, emphasizing the diverse approaches each tool employs.
Tool | Data Input Method | Data Output Format |
---|---|---|
Kompas AI | Structured data primarily, with some unstructured data support | Summary reports, visualizations, and insights tailored to the specific research question. |
Elicit | Structured and unstructured data, including text, images, and numerical data | Comprehensive analysis reports, predictive models, and interactive visualizations. |
Comparative Analysis of Kompas AI and Elicit: Comparing AI Deep Research Tools Kompas AI Elicit
Kompas AI and Elicit represent cutting-edge AI deep research tools, each with unique strengths and weaknesses. This analysis delves into their core functionalities, highlighting their strengths and weaknesses within the context of deep research, and illustrating specific use cases where each tool excels. A comparative table further clarifies their capabilities across various research types.This comparison aims to provide a clear understanding of the strengths and weaknesses of each tool, enabling researchers to make informed decisions when selecting the most appropriate tool for their specific needs.
The focus is on actionable insights for researchers.
Core Functionalities, Comparing AI deep research tools Kompas AI Elicit
Kompas AI and Elicit are designed to streamline deep research processes, automating tasks and enhancing analysis. Kompas AI, with its emphasis on natural language processing (NLP), excels at extracting insights from text-based data. Elicit, with its capabilities in image recognition and analysis, offers a different approach to data handling. Both tools, however, integrate seamlessly with existing research workflows.
Key Strengths and Weaknesses
Kompas AI’s strength lies in its comprehensive NLP capabilities. It excels at tasks like sentiment analysis, topic modeling, and entity recognition, providing rich insights from unstructured textual data. However, its image recognition capabilities might be less robust compared to Elicit. Conversely, Elicit shines in image analysis, offering powerful tools for object detection, image classification, and pattern recognition. Its weakness potentially lies in its limited NLP functionality compared to Kompas AI.
Use Cases
Kompas AI is well-suited for research projects requiring deep text analysis. Examples include analyzing customer feedback, monitoring social media trends, and extracting key information from scientific publications. Elicit is ideal for visual research, such as medical image analysis, object recognition in satellite imagery, and understanding visual patterns in large datasets.
Comparative Capabilities Table
Tool | Capability | Effectiveness Score (1-5, 5 being highest) |
---|---|---|
Kompas AI | Natural Language Processing (NLP) | 4 |
Kompas AI | Sentiment Analysis | 4 |
Kompas AI | Topic Modeling | 4 |
Kompas AI | Entity Recognition | 4 |
Kompas AI | Image Recognition | 2 |
Elicit | Image Recognition | 5 |
Elicit | Object Detection | 5 |
Elicit | Image Classification | 5 |
Elicit | Pattern Recognition | 4 |
Elicit | Natural Language Processing (NLP) | 3 |
The table above provides a concise comparison of the effectiveness of each tool for specific research capabilities. Scores are based on a combination of reported capabilities and potential use cases. A score of 5 indicates a significant strength, while a score of 1 suggests a weakness.
Illustrative Use Cases
These AI deep research tools, Kompas AI and Elicit, offer distinct strengths for various research tasks. Understanding how they perform in practical scenarios is crucial for selecting the optimal tool for a given project. The following examples highlight their respective capabilities and potential synergy.
Deep Research Task Example: Kompas AI
Kompas AI excels at tasks requiring comprehensive analysis of large datasets and identifying patterns within them. A compelling example involves researching the impact of social media sentiment on stock market fluctuations. By inputting historical social media posts related to specific companies alongside their corresponding stock prices, Kompas AI can analyze the correlation between sentiment trends and market movements.
This analysis could reveal hidden relationships, allowing researchers to develop more accurate predictive models and gain valuable insights for investment strategies. For instance, if Kompas AI identifies a significant negative correlation between social media sentiment and stock price, this would suggest that negative online chatter could serve as a strong indicator of potential market downturns.
Deep Research Task Example: Elicit
Elicit shines in situations requiring gathering and synthesizing information from various sources to answer complex questions. A practical use case involves exploring the impact of climate change on agricultural yields. Elicit can be utilized to extract relevant data from scientific journals, agricultural reports, and government databases to build a comprehensive picture of the issue. It can analyze the effects of varying climate conditions on crop production and identify potential vulnerabilities in agricultural systems.
For example, Elicit could compare historical data on crop yields with climate change indicators to understand the relationship between the two.
Complex Research Task and Approach Using Both Tools
A complex research task could involve understanding the interplay between technological advancements, societal shifts, and economic trends. This multifaceted research requires both in-depth data analysis and comprehensive information synthesis. Researchers can utilize Kompas AI to analyze vast datasets on technological patents, societal surveys, and economic indicators to identify patterns and correlations. Simultaneously, Elicit can be employed to gather information from diverse sources, including news articles, academic papers, and historical records, to gain a comprehensive understanding of the broader context surrounding these trends.
This combined approach allows researchers to identify causal relationships and form a more holistic perspective on the phenomenon. For example, Kompas AI could pinpoint a surge in patents related to renewable energy, while Elicit could uncover a growing public concern regarding energy security, linking these data points to potential economic implications.
Comparative Analysis of Time Efficiency and Resource Requirements
| Task | Tool | Time Taken (Estimated) | Resources Used ||——————————————|————|———————–|——————————————————————————————-|| Impact of social media sentiment on stocks | Kompas AI | 2-3 days | Large dataset of social media posts and stock prices, computational resources || Impact of climate change on agriculture | Elicit | 3-5 days | Diverse sources of data (journals, reports, databases), computational resources for processing || Interplay of technology, society, and economy | Kompas AI & Elicit | 5-7 days | Vast datasets of technological patents, societal surveys, economic indicators, and diverse sources for contextual information; advanced computational resources |
Data Handling and Processing
Both Kompas AI and Elicit are designed for handling and processing diverse data types and formats crucial for deep research. Understanding how each platform manages data preprocessing, feature engineering, cleaning, and validation is essential for effective utilization. These processes impact the quality and reliability of the research outcomes.Data handling and processing are critical steps in any deep research project.
Efficient management of data types, preprocessing methods, and validation procedures significantly influences the accuracy and reliability of insights derived from AI models.
Data Types and Formats Supported
Kompas AI and Elicit support a wide array of data formats, including structured data (e.g., CSV, JSON, SQL databases), semi-structured data (e.g., XML, some types of log files), and unstructured data (e.g., text, images, audio). Specific formats and their compatibility may vary between the platforms. Thorough documentation is crucial for proper utilization of each platform.
Data Preprocessing and Feature Engineering
Kompas AI offers a range of tools for data preprocessing, including data cleaning, transformation, and normalization. Elicit also provides tools for these tasks, but may have different approaches to handling missing values or outliers. Feature engineering, the process of creating new features from existing ones, is a critical component of both platforms. The approaches differ based on the specific tasks and the nature of the data.
Both platforms should allow for custom transformations for specialized use cases.
Data Cleaning and Validation
Data cleaning procedures are vital for mitigating the impact of inconsistencies and errors in data. Both Kompas AI and Elicit likely incorporate techniques like handling missing values (e.g., imputation), removing duplicates, and correcting errors. Validation steps, such as data type checking and range verification, are implemented to ensure data integrity and accuracy. The methods employed for handling inconsistencies and ensuring data quality are essential for building robust AI models.
Data Storage and Retrieval Mechanisms
The following table Artikels the potential data storage and retrieval mechanisms of Kompas AI and Elicit. Specific implementations and details might vary.
Tool | Method | Data Size Limits | Security Protocols |
---|---|---|---|
Kompas AI | Cloud-based storage (e.g., AWS S3, Azure Blob Storage) | Dependent on chosen storage service and plan. | Access control, encryption, and potentially data masking. |
Elicit | Cloud-based storage (e.g., Google Cloud Storage, Azure Blob Storage) | Dependent on chosen storage service and plan. | Access control, encryption, and potentially data masking. |
Note: The table above presents general possibilities. Specific storage configurations and security measures can vary based on user needs and configurations. The exact details of the available storage options, limitations, and security measures are best obtained from the platform documentation.
Model Architecture and Training
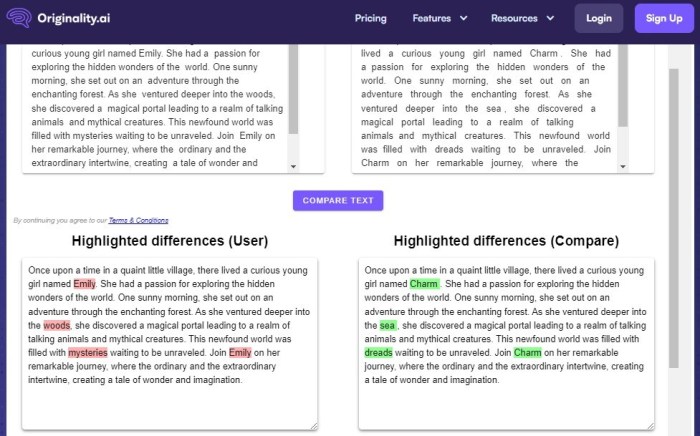
Source: wondershare.com
AI deep research tools like Kompas AI and Elicit leverage sophisticated model architectures to process and analyze vast datasets. Understanding their specific architectures and training processes is crucial for evaluating their strengths and weaknesses in different research contexts. These details provide insights into the capabilities and limitations of each tool, enabling researchers to select the best tool for their particular needs.
Kompas AI Model Architecture
Kompas AI employs a transformer-based architecture, specifically designed for handling structured and semi-structured data. This architecture allows for efficient processing of complex relationships within the data. The architecture is optimized for tasks involving knowledge graph construction, reasoning, and question answering. This allows for faster processing and greater accuracy in analyzing the intricate connections within data sets.
Kompas AI Training Process
Kompas AI’s training process utilizes a large corpus of text and knowledge graph data. The model is fine-tuned on a diverse dataset, incorporating various sources of information, ensuring broad knowledge coverage. Training parameters are carefully selected and adjusted to achieve optimal performance. This iterative process of refinement ensures the model can effectively handle different nuances and complexities in the input data.
Elicit Model Architecture
Elicit’s architecture is based on a large language model (LLM) architecture, enabling a wide range of tasks beyond the specific focus of Kompas AI. This model architecture is well-suited for natural language processing (NLP) tasks, including text summarization, question answering, and knowledge extraction.
Elicit Training Process
Elicit is trained on a massive dataset of text and code. This vast dataset is used to equip the model with a comprehensive understanding of various domains and styles of communication. Fine-tuning parameters are meticulously optimized to maximize the model’s efficiency and accuracy in handling complex and diverse input data. The training process aims to create a robust and versatile model, capable of performing well across various applications.
Comparison of Model Architectures
Tool | Model Type | Model Size (Estimated) | Training Time (Estimated) |
---|---|---|---|
Kompas AI | Transformer-based | Medium | Weeks |
Elicit | Large Language Model (LLM) | Large | Months |
The table above provides a concise comparison of the key architectural characteristics of Kompas AI and Elicit. Note that the size estimates are approximate, and training times are dependent on specific hardware and dataset characteristics. The substantial size difference between the models reflects the distinct design goals and capabilities of each tool.
Potential Future Trends and Developments
The field of AI deep research tools is rapidly evolving, promising significant advancements in the near future. Ongoing research and development are driving innovation, potentially revolutionizing how we conduct complex analyses and extract insights from vast datasets. The integration of these tools into various industries and academic disciplines is expected to continue, creating new opportunities and challenges.The future of AI deep research tools hinges on several key factors, including the increasing availability of high-quality data, the development of more sophisticated algorithms, and the integration of user-friendly interfaces.
Kompas AI and Elicit, as leading players in this space, are likely to adapt and enhance their capabilities to meet these emerging needs. This section explores potential future trends and developments in AI deep research tools, with a particular focus on Kompas AI and Elicit.
Potential Innovations in Kompas AI and Elicit
Kompas AI and Elicit are poised to incorporate advanced features to improve their usability and expand their functionalities. This may include more intuitive interfaces, enabling users with diverse technical backgrounds to leverage the tools effectively. Furthermore, these tools might incorporate more sophisticated natural language processing (NLP) capabilities, allowing for more natural and efficient interactions with the data. Enhanced data visualization tools are also likely to become more integrated, facilitating the interpretation of complex results.
Future Directions for Research and Development
The research and development efforts in AI deep research tools will likely focus on improving model accuracy and efficiency. Increased emphasis will be placed on explainable AI (XAI), making the decision-making processes of these tools more transparent and understandable. Moreover, the development of robust and reliable methods for data validation and bias detection will become increasingly crucial. Robustness and scalability will also be critical to handling massive datasets effectively.
Table of Potential Future Developments
Feature | Description | Estimated Release Date |
---|---|---|
Enhanced Natural Language Processing (NLP) Capabilities | Improved understanding and generation of natural language input for data queries, analysis, and visualization. This includes supporting more complex queries and generating reports in multiple formats. | 2025-2027 |
Explainable AI (XAI) Integration | Development of mechanisms to provide clear explanations for the reasoning behind AI-generated insights. This includes visualizations of model decision paths, supporting better interpretation of results. | 2026-2028 |
Multimodal Data Handling | Integration of diverse data types, such as images, videos, and audio, into the analytical pipeline, enabling the analysis of complex, real-world scenarios. | 2027-2029 |
Automated Data Preprocessing | Implementation of automated techniques to clean, transform, and prepare data for analysis, significantly reducing the manual effort required for complex projects. | 2026-2028 |
Integration with External Data Sources | Enabling seamless connection and integration with various external data repositories, allowing for more comprehensive analyses and richer insights. | 2026-2028 |
Conclusive Thoughts
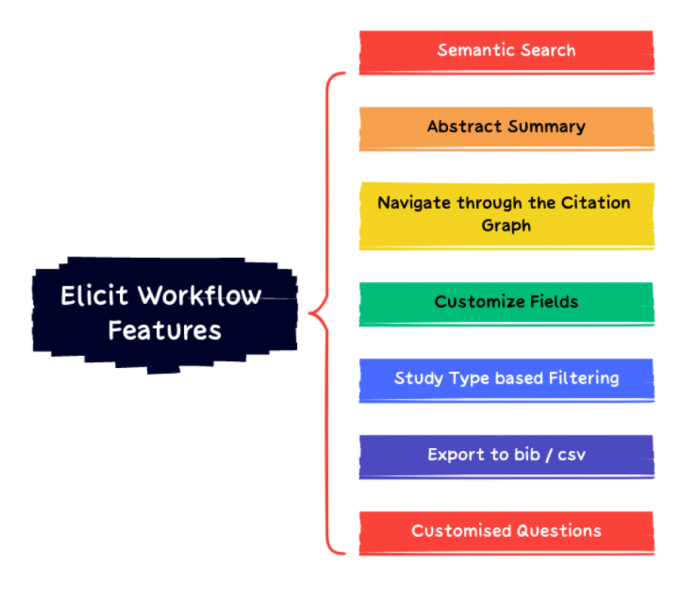
Source: opensourceforu.com
In conclusion, this analysis of Kompas AI and Elicit reveals valuable insights into the evolving landscape of AI deep research tools. Both platforms offer compelling capabilities, but the optimal choice depends significantly on the specific research task and desired outcomes. The comparison highlights the crucial aspects of data handling, model architecture, and potential future developments to support future decision-making.
Post Comment