Quantum Computing Impact On Medical Advancements
Quantum computing impact on medical advancements promises revolutionary breakthroughs in healthcare. This emerging field leverages the unique principles of quantum mechanics to tackle complex medical problems currently intractable for classical computers. From accelerating drug discovery to enhancing diagnostic capabilities, quantum computing offers the potential to transform the way we approach healthcare.
Quantum computing operates on principles unlike classical computers, using quantum bits (qubits) to perform calculations. This allows for the simulation of molecular interactions at an unprecedented level of detail, potentially leading to the development of highly effective drugs and personalized therapies. The ability to process vast amounts of medical data quickly and accurately will be crucial in improving diagnostic accuracy and precision medicine.
Introduction to Quantum Computing in Healthcare
Quantum computing, a burgeoning field, promises revolutionary advancements across various sectors, including healthcare. Its unique approach to data processing, leveraging quantum phenomena, could unlock solutions to complex medical challenges that classical computers struggle to address. This emerging technology holds the potential to accelerate drug discovery, personalize treatment plans, and enhance diagnostic accuracy.Quantum computing leverages the principles of quantum mechanics, such as superposition and entanglement, to manipulate information in ways classical computers cannot.
This allows for the exploration of vastly larger solution spaces, potentially leading to breakthroughs in areas where classical methods fall short. This fundamental difference in data manipulation profoundly impacts how we approach medical problems.
Quantum Computing Principles Relevant to Medical Applications
Quantum mechanics provides the theoretical foundation for quantum computing. Key principles include superposition, where a quantum bit (qubit) can exist in multiple states simultaneously, and entanglement, where two or more qubits are linked, regardless of the distance separating them. These phenomena enable quantum computers to explore numerous possibilities simultaneously, a capability unavailable to classical computers. This simultaneous exploration of multiple states is crucial for tackling the complexity of biological systems.
Quantum Algorithms for Medical Problems
Quantum algorithms are specialized procedures designed to exploit the unique capabilities of quantum computers. These algorithms are tailor-made for tasks that prove computationally intractable for classical computers, including simulating molecular interactions, optimizing treatment plans, and identifying patterns in large datasets. The application of quantum algorithms to medical problems could lead to significant improvements in areas like drug discovery, disease diagnosis, and personalized medicine.
Quantum Computers and Their Architectures for Healthcare
Quantum computers are still under development, with various architectures emerging. Superconducting qubits, trapped ions, and photonic qubits are among the leading contenders.
- Superconducting qubits utilize superconducting circuits to create and manipulate qubits. These systems are relatively mature and have demonstrated high qubit counts. The control and maintenance of these systems can be challenging.
- Trapped ions employ electromagnetic fields to trap and manipulate individual ions, enabling precise control over qubits. These systems excel in maintaining coherence but face limitations in scaling to large numbers of qubits.
- Photonic qubits leverage photons (particles of light) to carry quantum information. Their ability to transmit information over long distances makes them promising for distributed quantum computing applications. They are still in early development stages, posing challenges in maintaining qubit coherence.
Quantum Computing’s Difference from Classical Computing
Quantum computing differs from classical computing in its fundamental approach to data processing. Classical computers use bits, which can only represent a 0 or a 1. Quantum computers use qubits, which can represent both 0 and 1 simultaneously, thanks to superposition. This ability to explore multiple possibilities simultaneously allows quantum computers to tackle problems that are computationally intractable for classical computers.
Quantum algorithms can potentially solve complex problems in medicine, such as simulating molecular interactions with unprecedented accuracy.
Drug Discovery and Development: Quantum Computing Impact On Medical Advancements
Quantum computing promises a significant leap forward in drug discovery and development, offering unprecedented speed and efficiency in identifying potential drug candidates and optimizing their properties. Traditional methods often face limitations in simulating complex molecular interactions, leading to lengthy and costly processes. Quantum algorithms, however, can potentially revolutionize this field by tackling these limitations head-on.Quantum computing’s ability to simulate molecular interactions with remarkable accuracy is poised to dramatically accelerate the identification of novel drug candidates.
This acceleration will likely reduce the time and resources required for bringing new treatments to market, potentially impacting healthcare in profound ways.
Potential of Quantum Algorithms in Drug Simulation
Quantum algorithms, particularly those leveraging quantum annealing and variational quantum eigensolver (VQE), are showing promise in simulating molecular interactions. These simulations can predict the behavior of molecules, including their binding affinity to target proteins, which is crucial for drug design. Accurate predictions of molecular behavior can significantly reduce the number of compounds that need to be experimentally tested, thereby significantly decreasing the time and cost of drug development.
Comparison with Traditional Methods
Traditional drug discovery relies heavily on empirical methods and high-throughput screening. While these methods have yielded numerous breakthroughs, they often prove inefficient in tackling complex molecular interactions. Quantum simulations, on the other hand, offer the potential to simulate these interactions with significantly higher accuracy and speed. This superior accuracy is particularly important in identifying subtle differences in molecular structures that could affect drug efficacy.
Consequently, quantum simulations can be a game changer, leading to more effective and targeted drug therapies.
Integration of Quantum Computing into Drug Discovery Stages
Stage of Drug Discovery | How Quantum Computing Can Be Integrated |
---|---|
Target Identification | Quantum simulations can predict the binding affinity of potential drug candidates to target proteins with greater accuracy and speed. This can help identify promising targets and prioritize them for further investigation. |
Lead Optimization | Quantum simulations can explore a wider range of molecular structures and predict the effects of modifications on drug properties, such as stability and efficacy. This can guide the optimization of lead compounds to enhance their desired characteristics. |
Drug Design | Quantum simulations can model the interaction of the drug candidate with its target at an atomic level, allowing for a deeper understanding of the molecular mechanisms. This can lead to the design of more potent and specific drugs. |
Toxicity Assessment | Quantum simulations can predict the potential toxicity of drug candidates by analyzing their interactions with other molecules in the body. This can identify potential side effects and help design safer drugs. |
Clinical Trials | Quantum simulations can assist in predicting drug response in different patient populations, helping to refine clinical trial design and target the most promising candidates. |
Diagnostics and Imaging
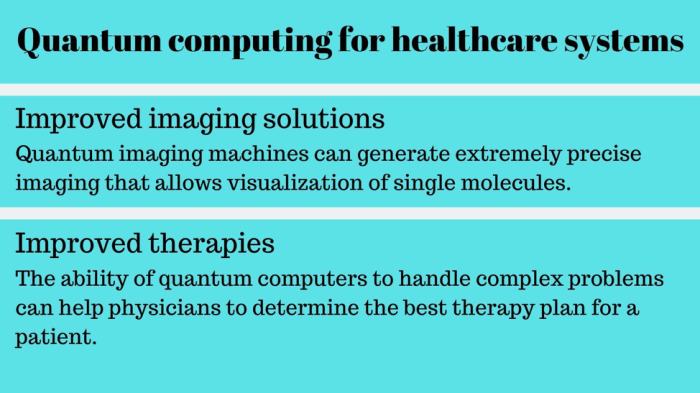
Source: allerin.com
Quantum computing promises a revolutionary transformation in medical diagnostics and imaging, offering unprecedented accuracy and efficiency. Current methods, while valuable, often face limitations in speed, sensitivity, and data interpretation. Quantum computing’s unique capabilities, leveraging principles of superposition and entanglement, can address these limitations and pave the way for more precise and faster diagnoses.
Potential for Enhanced Diagnostic Accuracy
Quantum algorithms can analyze complex medical images, such as X-rays, CT scans, and MRIs, with significantly greater precision than traditional methods. This heightened accuracy can lead to earlier detection of diseases, more accurate staging, and ultimately, improved patient outcomes. Quantum algorithms can identify subtle patterns and anomalies that might be missed by the human eye or conventional algorithms, enhancing the ability to detect diseases like cancer in their early stages.
Quantum Sensing and Metrology
Quantum sensing techniques, leveraging quantum phenomena like entanglement and superposition, offer the potential for highly sensitive and precise measurements in medical diagnostics. These sensors can measure biophysical parameters with unprecedented accuracy, providing detailed insights into biological processes and disease states. For example, quantum sensors can detect minute changes in tissue density or blood flow, enabling early diagnosis of conditions like atherosclerosis or tumors.
Quantum Algorithms for Medical Data Analysis
Quantum algorithms excel at processing vast datasets and identifying complex patterns, which is crucial for medical data analysis. Quantum machine learning algorithms, for instance, can be trained on massive datasets of patient records, genetic information, and imaging data to predict disease risk, identify potential drug targets, and develop personalized treatment plans. This approach allows for the development of highly sophisticated diagnostic tools that adapt to individual patient needs and characteristics.
Comparison of Current Diagnostic Limitations and Quantum Solutions
Current Diagnostic Tool Limitation | Quantum Computing Solution |
---|---|
Limited resolution and sensitivity in medical imaging | Quantum sensors and algorithms can achieve higher resolution and sensitivity, detecting subtle changes that traditional methods might miss. |
Time-consuming analysis of large datasets | Quantum algorithms can process vast amounts of medical data much faster, enabling quicker diagnoses. |
Difficulty in identifying subtle patterns in complex data | Quantum machine learning algorithms can analyze complex medical data, identifying intricate patterns and anomalies that might be missed by human interpretation or conventional algorithms. |
Limited ability to personalize diagnostics | Quantum algorithms can analyze patient-specific data to provide tailored diagnostic insights and treatment recommendations. |
High cost of advanced diagnostic equipment | Quantum computing could potentially lead to more cost-effective diagnostic solutions by enabling faster and more accurate analysis of existing data, reducing the need for costly equipment. |
Personalized Medicine
Personalized medicine leverages individual patient characteristics, including genetic makeup, lifestyle, and environmental factors, to tailor treatment strategies. This approach promises improved efficacy and reduced adverse effects compared to traditional, one-size-fits-all treatments. Quantum computing’s potential to analyze vast datasets and identify complex patterns within individual patient data presents an exciting opportunity to refine personalized medicine further.
Quantum Computing’s Role in Personalized Treatment Strategies
Quantum algorithms can process the intricate details of individual patient data with remarkable speed and accuracy. This includes genomic data, medical imaging, and lifestyle information, allowing for a more comprehensive understanding of each patient’s unique biology and response to various treatments. By analyzing these intricate patterns, quantum computing can help predict individual responses to specific drugs, identify optimal treatment combinations, and even anticipate potential side effects.
Analysis of Individual Patient Data
Quantum machine learning algorithms can effectively analyze complex datasets associated with individual patients, potentially uncovering hidden correlations and insights. This analysis can go beyond traditional statistical methods by identifying intricate patterns in large datasets. For instance, analyzing patient genetic profiles, alongside lifestyle factors and environmental exposures, could reveal subtle indicators of disease predisposition or responses to therapies, allowing for more targeted and precise interventions.
This enhanced data analysis can lead to tailored treatment plans, predicting the efficacy of specific drugs and therapies for individual patients.
Challenges in Large-Scale Implementation
Implementing quantum computing for large-scale personalized medicine faces several challenges. Firstly, the availability of high-quality, standardized patient data is crucial for training and validating quantum algorithms. Secondly, the computational resources required for quantum computing are currently limited, impacting the speed and scalability of the process. Furthermore, the expertise needed to develop and interpret quantum algorithms within the healthcare context is still evolving, demanding specialized training and support.
Ethical Considerations and Privacy Concerns
Utilizing quantum computing in personalized medicine raises crucial ethical and privacy concerns. The analysis of sensitive patient data necessitates stringent data security measures to protect patient confidentiality and prevent unauthorized access. Clear guidelines and regulations are essential to ensure the responsible use of quantum computing in personalized medicine. Additionally, the potential for biases in the data used to train quantum algorithms must be carefully considered and mitigated to avoid exacerbating existing health disparities.
Transparency and patient consent are paramount in ensuring that patients are fully informed about how their data is used and that their privacy is protected. The development and implementation of robust ethical frameworks are vital to navigate the ethical implications of this emerging technology.
Bioinformatics and Genomics
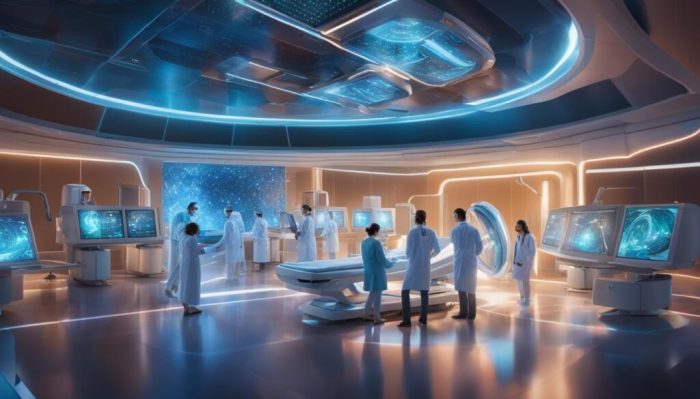
Source: augmentedqubit.com
Genomic data, representing the complete set of genetic instructions within an organism, is crucial for understanding health and disease. This data, often voluminous and complex, presents significant analytical challenges for traditional computing approaches. Quantum computing offers the potential to revolutionize bioinformatics and genomics by enabling the analysis of intricate patterns within vast datasets.Quantum algorithms can potentially speed up the process of identifying disease-related genetic variations, predicting patient responses to treatments, and ultimately leading to more personalized and effective healthcare strategies.
This involves analyzing genetic variations, gene expression patterns, and protein interactions to understand disease mechanisms and develop new therapeutic targets.
Quantum Analysis of Genomic Datasets
Vast genomic datasets, often exceeding the capacity of classical computers, pose significant challenges in identifying subtle disease patterns and predicting patient outcomes. Quantum computing, with its unique capabilities, offers a potential solution. Quantum algorithms can leverage superposition and entanglement to explore the immense search space within genomic data far more efficiently than classical methods. This allows for faster identification of disease-related genes, mutations, and regulatory elements.
Examples of Quantum Algorithms
Several quantum algorithms are being explored for their potential application in genome sequencing and analysis. Variational Quantum Eigensolver (VQE) algorithms are useful for studying protein-protein interactions, critical for understanding disease mechanisms. Quantum Approximate Optimization Algorithm (QAOA) can be applied to identify key genetic markers associated with specific diseases. Quantum machine learning algorithms can analyze complex genomic relationships, uncovering hidden patterns and improving disease prediction accuracy.
Quantum vs. Classical Computing for Genomics
Classical computers, while powerful, face limitations when dealing with the sheer volume and complexity of genomic data. Classical algorithms often require significant time and resources for analyzing large datasets, hindering the pace of research and development. Quantum computers, leveraging quantum phenomena, promise to significantly accelerate these processes, enabling the identification of novel disease patterns and personalized treatment strategies.
While classical algorithms can analyze data sets with certain limitations, quantum computing can address this, potentially leading to breakthroughs in understanding disease mechanisms and developing personalized therapies.
Quantum Algorithms Summary
Algorithm | Description | Application |
---|---|---|
Variational Quantum Eigensolver (VQE) | Optimizes a parameterized quantum circuit to approximate the ground state energy of a molecule or system. | Protein-protein interactions, understanding disease mechanisms. |
Quantum Approximate Optimization Algorithm (QAOA) | Approximates the solution to combinatorial optimization problems. | Identifying key genetic markers, predicting patient responses. |
Quantum Machine Learning Algorithms | Leverage quantum principles for tasks such as classification and clustering. | Analyzing complex genomic relationships, improving disease prediction accuracy. |
Quantum Simulation of Biological Systems
Quantum computers offer a unique capability to simulate biological systems at a molecular level, a task intractable for even the most powerful classical supercomputers. This ability opens exciting avenues for understanding complex biological processes and diseases, ultimately accelerating drug discovery and development. By simulating the intricate interactions within molecules and cells, quantum computers can reveal insights that would otherwise remain hidden.Simulating biological systems at a molecular level is crucial for comprehending the intricacies of life’s processes.
Quantum computers can delve into the intricate dance of electrons and molecules, revealing interactions that are difficult to perceive using traditional methods. This detailed understanding can lead to breakthroughs in fields like medicine, agriculture, and materials science.
Modeling Biological Pathways
Understanding the complex biological pathways involved in diseases is essential for developing effective treatments. Quantum simulations can model these pathways, revealing the intricate interactions of molecules and the steps involved in disease progression. This allows for a deeper comprehension of the disease mechanism, enabling the design of targeted therapies. By analyzing the intricate interplay of molecules in a pathway, quantum simulations can uncover hidden connections and vulnerabilities, offering new strategies for intervention.
Predicting Treatment Effects
Quantum simulations can predict the effects of various treatments on biological systems, accelerating drug discovery and personalized medicine. By simulating how molecules interact with biological targets, researchers can anticipate the efficacy and potential side effects of a drug candidate, significantly reducing the time and resources required for experimental testing. This predictive capability can accelerate the development of more effective and safer treatments, leading to a faster pace of progress in medicine.
Quantum Simulation of Biological Processes
Biological Process | Quantum Simulation Approach | Potential Insights |
---|---|---|
Protein Folding | Simulating the interactions between amino acids to predict protein structure and function. | Understanding how proteins misfold in diseases like Alzheimer’s and Parkinson’s, leading to potential drug targets. |
Enzyme Catalysis | Modeling the interactions of enzymes with substrates to understand reaction mechanisms. | Developing new enzyme inhibitors with higher efficacy and fewer side effects. |
DNA Replication | Simulating the intricate steps of DNA replication to understand the process and potential errors. | Identifying potential targets for therapies aimed at correcting DNA replication errors and preventing diseases. |
Cellular Signaling Pathways | Modeling the interactions of molecules in signaling cascades to understand disease progression. | Developing drugs that target specific steps in a signaling pathway to treat diseases. |
Drug-Target Interactions | Simulating the binding of drugs to their target molecules to predict efficacy and potential side effects. | Identifying promising drug candidates with high efficacy and low toxicity, significantly accelerating drug development. |
Challenges and Future Directions
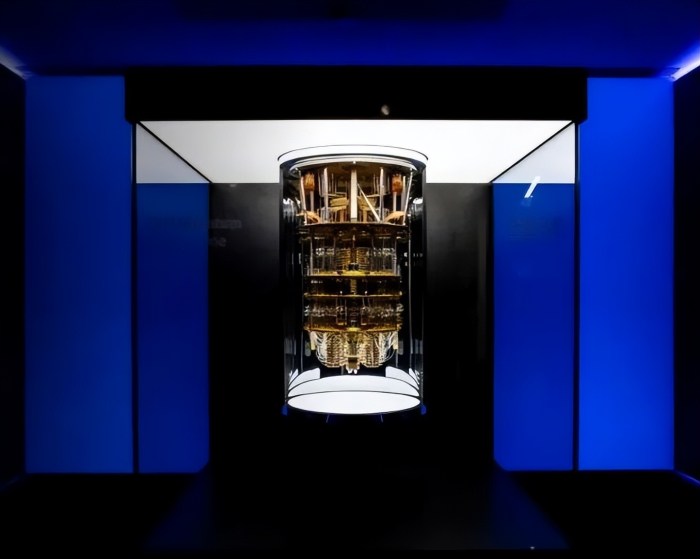
Source: byteimg.com
The burgeoning field of quantum computing presents a revolutionary opportunity to reshape medical advancements, but numerous hurdles remain in its practical application. Overcoming these technical and logistical challenges is crucial for realizing the full potential of quantum computing in healthcare. This section explores the significant obstacles and proposes potential pathways for future development.
Technical Challenges in Implementation
Current quantum computers are notoriously fragile and prone to errors. These “quantum errors,” often caused by environmental interactions, significantly limit the size and complexity of problems they can handle. Maintaining the delicate quantum states required for computation is a major technical hurdle. Moreover, the specialized expertise required to program and operate these machines is a significant barrier to wider adoption.
Limitations of Current Quantum Hardware, Quantum computing impact on medical advancements
The current generation of quantum computers is limited in terms of qubit count and coherence time. The number of qubits directly impacts the complexity of problems that can be addressed. The coherence time, which dictates how long quantum information can be maintained without decoherence, also restricts the duration of computations. These limitations prevent the effective simulation of complex biological systems, a critical aspect of drug discovery and personalized medicine.
For example, simulating the intricate interactions within a protein structure to predict its function or design a new drug requires significantly more qubits and extended coherence time than current hardware allows.
Need for Future Advancements
Future advancements in quantum hardware are paramount for widespread adoption in medicine. Increased qubit counts, improved coherence times, and reduced error rates are essential. Development of more robust qubit technologies, such as superconducting circuits or trapped ions, is crucial. Further research in quantum error correction techniques will be vital to mitigating the impact of errors.
Potential Solutions to Overcome Challenges
Several strategies can accelerate the integration of quantum computing into healthcare. The development of more sophisticated quantum error correction protocols can enhance the reliability and stability of quantum computations. Improved control and manipulation techniques for qubits will lead to more efficient and accurate calculations. Furthermore, developing more user-friendly interfaces and programming languages for quantum algorithms will make quantum computing more accessible to researchers in diverse fields.
Table: Current State of Quantum Computing Hardware and Limitations in Medical Applications
Hardware Type | Qubit Count | Coherence Time | Error Rate | Limitations in Medical Applications |
---|---|---|---|---|
Superconducting circuits | ~100-1000 | ~µs – ms | High | Limited ability to simulate large biological systems, high error rate hinders accuracy |
Trapped ions | ~10-100 | ~ms – s | Moderate | Scalability challenges, complex setup |
Photonic qubits | ~10-100 | ~ns – µs | High | Challenges in qubit connectivity and maintaining coherence |
Illustrative Examples
Quantum computing holds immense potential to revolutionize medical research and treatment. Its ability to simulate complex biological systems and analyze vast datasets promises breakthroughs in drug discovery, diagnostics, and personalized medicine. This section explores concrete examples of how quantum computing is already impacting, and will further impact, medical advancements.
Quantum Simulation of Protein Folding
Accurate protein folding prediction is crucial for understanding diseases and developing new therapies. Classical computers struggle with the sheer complexity of protein structures and interactions. Quantum computers, with their ability to explore multiple possibilities simultaneously, can model protein folding with unprecedented accuracy. A significant advantage is the ability to simulate the intricate interplay of forces within a protein.
- Example: Researchers are using quantum simulations to model the folding of proteins involved in Alzheimer’s disease. This allows for a deeper understanding of the disease mechanism, potentially paving the way for targeted therapies.
- Process: The process involves using quantum algorithms to map the protein’s amino acid sequence onto a quantum system. The quantum computer then simulates the protein’s folding dynamics, identifying the stable conformations. This information can then be used to design drugs that interact with the protein in a specific manner.
Drug Discovery and Development
Quantum computing offers the potential to dramatically accelerate drug discovery by significantly reducing the time and resources needed to identify promising drug candidates. It can simulate molecular interactions with unparalleled speed and precision, allowing for the screening of millions of potential drug molecules in a fraction of the time it would take classical methods.
- Example: Quantum computers can predict the binding affinity of a molecule to a target protein. This information can guide the design of new drugs with higher efficacy and fewer side effects.
- Detailed Process: Quantum algorithms can simulate the interactions between a drug molecule and a target protein. The output data reveals the binding energy, which predicts the drug’s ability to interact with the target protein and the probability of success.
- Result: This accelerates the drug development process by significantly reducing the number of experiments needed to identify promising drug candidates.
Diagnostics and Imaging
Quantum sensing techniques offer the potential to improve diagnostic tools, providing highly sensitive and accurate measurements. Quantum algorithms can process and interpret medical imaging data, potentially enabling earlier and more precise diagnoses.
- Example: Quantum sensors could be integrated into medical imaging devices like MRI machines, enabling higher resolution images and more accurate measurements of biological parameters.
- Quantum Algorithm Example: A quantum algorithm could be designed to identify subtle changes in tissue density or blood flow, potentially detecting early-stage diseases like cancer.
Personalized Medicine
Quantum computing can facilitate personalized medicine by analyzing individual genetic data and tailoring treatments to specific patient needs. This approach considers individual genetic variations, environmental factors, and lifestyle choices to provide the most effective treatment strategy.
- Example: Quantum algorithms can analyze a patient’s genome to identify specific genetic mutations associated with a particular disease. This allows for more precise diagnosis and treatment planning.
Final Review
In conclusion, quantum computing’s impact on medical advancements is poised to fundamentally reshape healthcare. While significant challenges remain, including the development of more powerful quantum hardware and the integration of these technologies into existing clinical workflows, the potential benefits are immense. From personalized medicine to advanced diagnostics, quantum computing promises to bring about a new era of medical innovation and improved patient outcomes.
Post Comment