AI Risks Dangers and Impacts
Risks and dangers of artificial intelligence are a growing concern as AI technology rapidly advances. This exploration delves into the multifaceted challenges, from job displacement and economic repercussions to security vulnerabilities and ethical dilemmas. The potential for bias, misuse, and even existential threats demands careful consideration and proactive strategies.
The development and deployment of AI present a complex web of potential risks. We will examine how AI systems can perpetuate existing societal inequalities, threaten critical infrastructure, and raise fundamental questions about privacy and security. Furthermore, the possibility of AI surpassing human intelligence and the need for responsible development will be explored.
Job Displacement and Economic Impacts: Risks And Dangers Of Artificial Intelligence
The rapid advancement of artificial intelligence (AI) presents both exciting opportunities and significant challenges, particularly regarding the future of work. AI-powered automation has the potential to drastically alter the job market, impacting employment levels and economic structures across various sectors. Understanding these potential impacts is crucial for proactive measures to mitigate risks and maximize benefits.AI’s ability to automate tasks previously performed by humans is undeniable.
From manufacturing to customer service, AI algorithms are increasingly capable of performing complex operations with speed and precision, potentially leading to job displacement in certain roles. This necessitates a careful examination of the economic implications and a proactive approach to supporting workers and industries in transition.
Potential Job Displacement
AI’s impact on employment is multifaceted. While AI will likely augment human capabilities in many areas, its potential to automate tasks previously performed by humans cannot be ignored. Certain roles in manufacturing, transportation, and customer service are at higher risk of automation, with a significant portion of routine tasks being taken over by AI systems. This could lead to significant job losses in these sectors.
Examples include automated assembly lines in factories, self-driving trucks replacing human drivers, and AI-powered chatbots handling customer inquiries.
Economic Consequences
The economic consequences of widespread job displacement are substantial and multifaceted. Reduced employment can lead to a decline in consumer spending, impacting economic growth. Moreover, a widening gap in income inequality is a potential outcome, as highly skilled workers who are able to adapt to the changing job market could experience increased compensation, while those in jobs susceptible to automation could face stagnating or declining wages.
This could exacerbate existing social and economic divides. Historical examples of technological advancements causing temporary economic disruptions and societal shifts are relevant to understanding the potential consequences.
Mitigation Strategies
Addressing the potential for job losses necessitates a multifaceted approach. Investing in education and training programs is crucial to equip workers with the skills needed in an AI-driven future. Upskilling initiatives should focus on areas like data analysis, AI development, and digital literacy. Furthermore, policies aimed at supporting workforce transitions, such as job placement assistance and retraining programs, will be critical.
Government support for businesses adapting to the AI revolution is equally important.
Skills Needed in an AI-Driven Future
Current Job Skills | AI-Driven Future Job Skills |
---|---|
Routine tasks, specific industry knowledge | Critical thinking, problem-solving, creativity, adaptability, data analysis, AI literacy |
Manual labor, repetitive actions | Advanced technical skills, project management, AI systems integration |
Specific software proficiency | Programming languages, data science, machine learning, algorithm design |
Specialized knowledge in particular fields | Interdisciplinary expertise, collaboration, communication, strategic planning |
The table highlights a clear shift in the required skillset. The future demands individuals with a broader range of skills, capable of working alongside AI systems rather than being directly replaced by them. This underscores the importance of continuous learning and adaptability.
New Job Creation in the AI Sector
AI development, implementation, and maintenance will undoubtedly create new job opportunities. AI engineers, data scientists, AI ethicists, and AI trainers will be in high demand. Furthermore, the need for specialists in AI-related fields, such as cybersecurity and AI-related legal expertise, will likely increase.
Government Policies
Government policies play a pivotal role in shaping the future of work in an AI-driven world. Policies that encourage investment in education and training programs, support workforce transitions, and promote the development of a skilled workforce are crucial. Moreover, policies addressing potential income inequality and the ethical implications of AI are necessary. Examples of such policies include targeted tax incentives for companies investing in reskilling initiatives and establishing social safety nets to support individuals impacted by job displacement.
Bias and Discrimination in AI Systems
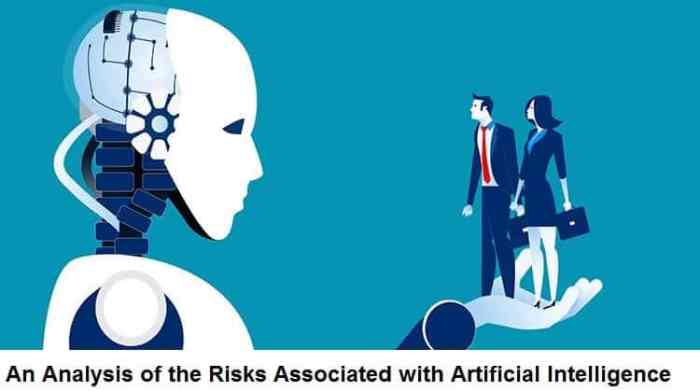
Source: inspiringmeme.com
Artificial intelligence systems, while powerful, can inherit and amplify biases present in the data they are trained on. This inherent bias can lead to discriminatory outcomes in various applications, impacting individuals and society as a whole. Understanding these biases and developing strategies to mitigate them is crucial for responsible AI development.AI systems learn patterns from data. If the data reflects existing societal biases, the AI system will likely perpetuate and even amplify those biases.
This is particularly concerning in areas like loan applications, hiring processes, and even criminal justice, where fairness and objectivity are paramount. Addressing these biases is not just an ethical imperative, but also a practical one, as biased systems can lead to significant societal and economic harms.
Potential Biases Embedded in AI Algorithms and Datasets
The data used to train AI algorithms can reflect societal prejudices, leading to biased outputs. These biases can be explicit or implicit, conscious or unconscious. For instance, datasets used for facial recognition might be skewed towards a specific demographic, resulting in lower accuracy rates for other groups. Historical data, particularly in financial or criminal justice contexts, may contain biases that are not readily apparent.
Moreover, algorithms themselves can be designed in ways that inadvertently perpetuate bias. For example, a loan application algorithm trained on historical data reflecting racial or gender disparities might perpetuate these same disparities in its lending decisions.
Societal Consequences of Biased AI
Biased AI systems can have severe societal consequences. They can lead to discriminatory outcomes in areas like employment, housing, and loan applications, exacerbating existing inequalities. Individuals and groups may face unfair treatment or denial of opportunities simply due to their characteristics, not their merit. This can lead to significant economic and social disparities, impacting individuals’ quality of life and opportunities.
For example, biased loan algorithms might deny loans to people from minority groups, preventing them from purchasing homes or starting businesses.
Examples of Biased AI Applications
Biased AI can manifest in various applications. In loan applications, algorithms trained on historical data that shows disparities in lending practices based on race or gender can perpetuate these biases. This can lead to unequal access to credit, impacting economic opportunities for certain groups. In hiring processes, algorithms trained on resumes from predominantly one demographic might inadvertently discriminate against applicants from other backgrounds.
Facial recognition systems, if trained on datasets biased toward a particular race or gender, might perform poorly or inaccurately identify individuals from other groups.
Approaches to Mitigate Bias in AI Systems
Several approaches can be employed to mitigate bias in AI systems. These include:
- Careful dataset curation and auditing: Identifying and correcting biases in the training data is crucial. This involves analyzing the data for imbalances and inconsistencies, and taking steps to ensure representation from diverse groups. For instance, if a dataset is skewed towards one particular race, it should be expanded to include data from other races to provide a more balanced representation.
- Algorithm design considerations: The design of the algorithms themselves should be scrutinized to prevent bias from arising. Algorithms that rely on factors unrelated to the task at hand should be questioned, and alternative approaches should be considered. For example, if a loan application algorithm uses historical data with embedded racial bias, alternative algorithms focusing on individual creditworthiness can be considered.
- Bias detection and mitigation techniques: Employing techniques to detect and mitigate bias in AI systems is vital. Methods such as fairness-aware algorithms, adversarial debiasing, and data augmentation can help address biases during the training and deployment of AI systems. For instance, fairness-aware algorithms can be used to adjust the output of an algorithm to ensure that it does not disproportionately impact specific groups.
Ethical Considerations Surrounding Unbiased AI
Developing and deploying unbiased AI systems raises significant ethical considerations. Transparency in the development process is essential to ensure accountability and understanding of how AI systems work and how biases may be introduced. Equitable access to AI technologies must be ensured, and the potential for misuse of unbiased AI systems must be carefully considered. A strong regulatory framework that balances innovation with ethical considerations is necessary to govern the development and deployment of AI systems.
Types of Bias and Potential Impacts
Type of Bias | Potential Impact |
---|---|
Data Bias | AI systems trained on biased data will perpetuate and amplify those biases, leading to discriminatory outcomes. |
Algorithmic Bias | The design of the algorithms themselves can inadvertently perpetuate bias, leading to unequal outcomes. |
Measurement Bias | Inadequate or biased data collection methods can introduce bias into the training data, influencing AI outcomes. |
Selection Bias | Biased selection of data for training can create AI systems that favor specific groups over others, impacting opportunities. |
Security Risks and Malicious Use
The rapid advancement of artificial intelligence (AI) presents a double-edged sword. While AI offers immense potential for progress, its vulnerabilities to malicious use pose significant security risks. Understanding these risks is crucial for mitigating potential threats and ensuring the responsible development and deployment of AI technologies.AI systems, like any complex software, can be vulnerable to hacking and manipulation.
Weaknesses in the algorithms, data inputs, or underlying infrastructure can be exploited by malicious actors, leading to unpredictable consequences. This necessitates a proactive approach to security in the AI development lifecycle.
Vulnerabilities to Hacking and Misuse
AI systems are susceptible to a range of vulnerabilities that can be exploited by malicious actors. These vulnerabilities stem from various sources, including the algorithms themselves, the data used to train them, and the infrastructure supporting their operation. Weaknesses in these areas can lead to manipulated outputs, incorrect predictions, or even complete system failure.
- Data Poisoning: Malicious actors can introduce tainted data into the training dataset, effectively “poisoning” the AI model and causing it to make erroneous predictions or behave in undesirable ways. This can have devastating consequences in areas such as medical diagnosis or financial forecasting.
- Adversarial Attacks: These attacks involve carefully crafted inputs designed to mislead the AI system. These “adversarial examples” can cause the AI to misinterpret information or produce incorrect outputs. For instance, slightly altering an image can fool a facial recognition system into misidentifying a person.
- Supply Chain Attacks: AI systems rely on numerous components and services throughout their lifecycle. An attack on one of these components, such as the cloud provider hosting the AI model, can have significant consequences for the overall system.
Threats to Critical Infrastructure and National Security
AI’s potential for misuse extends beyond individual systems. Its integration into critical infrastructure, such as power grids and transportation networks, raises significant security concerns. A successful attack on such systems could have devastating consequences, impacting national security and public safety.
- Autonomous Weapons Systems: The development of autonomous weapons systems, capable of selecting and engaging targets without human intervention, raises ethical and security concerns. The potential for accidental escalation or unintended consequences is significant.
- Cyberattacks Leveraging AI: AI can be used to enhance the capabilities of cyberattacks. For example, AI-powered tools can automate the process of identifying vulnerabilities, generating malicious code, and launching attacks, making them more sophisticated and difficult to defend against.
Examples of Malicious Use
The potential for malicious use of AI is substantial. The ease with which AI can be adapted for harmful purposes is a growing concern.
- Deepfakes: AI-powered deepfake technology can create realistic but fabricated videos and audio recordings, enabling the creation of misleading content that can be used for disinformation campaigns or blackmail. These technologies have the potential to undermine trust in information and sow chaos.
Importance of Robust Security Protocols
Robust security protocols are essential for safeguarding AI systems against malicious use. A comprehensive approach should address all stages of the AI development lifecycle, from data collection to deployment.
- Secure Development Practices: Implementing secure development practices, such as incorporating security considerations into the design and development process, is crucial. This includes incorporating robust testing procedures and regularly assessing the system for vulnerabilities.
- Robust Data Protection: Protecting the data used to train AI models is critical. This includes implementing robust encryption, access controls, and data anonymization techniques.
Different Approaches to Ensuring AI Security
Multiple approaches can be employed to bolster AI system security.
- Formal Verification: This involves mathematically proving the correctness and security of AI systems, which can help identify potential vulnerabilities before deployment.
- Security Audits and Penetration Testing: Regularly conducting security audits and penetration testing can help identify and mitigate potential weaknesses in AI systems.
Potential for AI-Powered Cyberattacks
AI has the potential to significantly enhance the capabilities of cyberattacks. Sophisticated AI algorithms can automate various stages of an attack, making them more efficient and difficult to detect.
Privacy Concerns and Data Security
AI systems, by their very nature, rely heavily on vast amounts of personal data. This reliance raises significant concerns regarding individual privacy. The collection, use, and storage of this data necessitate careful consideration of potential risks and ethical implications. Robust data protection regulations are crucial to mitigate these risks and balance the benefits of AI with the fundamental right to privacy.
Potential Threats to Individual Privacy
AI systems can pose significant threats to individual privacy by collecting, analyzing, and utilizing personal data in ways that individuals may not fully understand or control. These systems often operate with complex algorithms, making it challenging to trace the flow of information and understand the ultimate uses of the collected data. This lack of transparency can erode trust and create anxieties about potential misuse.
For example, facial recognition technology, widely used in various applications, can be employed for surveillance, potentially leading to unwarranted tracking and monitoring of individuals.
Collection, Use, and Storage of Personal Data
The collection of personal data in AI applications is often extensive and multifaceted. Data sources include social media activity, online searches, purchase history, location data, and even interactions with smart devices. The use of this data varies widely, from personalized recommendations to targeted advertising, and in some cases, for predictive modeling of individual behavior. Data storage practices must adhere to strict security protocols to prevent unauthorized access and misuse.
Failing to implement robust data security measures can expose sensitive personal information to breaches and exploitation.
Examples of AI-Based Tracking and Monitoring
AI-powered surveillance systems, often employing sophisticated algorithms and machine learning models, can track and monitor individuals in real-time. These systems can analyze vast quantities of data, such as video footage, geolocation information, and online interactions, to identify patterns and predict behavior. For example, predictive policing algorithms, based on historical crime data, can identify potential crime hotspots, but this raises concerns about potential bias and disproportionate targeting of certain communities.
Similarly, social media platforms utilize AI to analyze user data for targeted advertising, which can be perceived as a form of surveillance and data collection.
Need for Robust Data Protection Regulations
Strong data protection regulations and policies are essential to ensure the responsible development and deployment of AI systems. These regulations should address the collection, use, storage, and disposal of personal data. They should establish clear guidelines on data minimization, purpose limitation, and data subject rights, including the right to access, rectify, and erase personal data. Without these regulations, individuals’ privacy rights are vulnerable to the potential abuses of powerful AI systems.
Balancing Privacy Concerns with AI Benefits, Risks and dangers of artificial intelligence
Finding the right balance between the benefits of AI and the protection of individual privacy is a critical challenge. While AI offers numerous opportunities to improve efficiency, enhance decision-making, and personalize experiences, it also necessitates careful consideration of the potential risks to privacy. A thoughtful approach that prioritizes data security and individual rights while recognizing the benefits of AI is paramount.
Table of Personal Data Collected by AI Systems
Type of Personal Data | Potential Risks |
---|---|
Location data (GPS, Wi-Fi) | Surveillance, tracking, potential for misuse in location-based services |
Financial transactions | Financial fraud, unauthorized access to accounts, potential for identity theft |
Health records | Unauthorized disclosure of sensitive medical information, discrimination based on health status |
Social media interactions | Data breaches, targeted advertising, profiling, potential for manipulation |
Online browsing history | Profiling, targeted advertising, lack of control over data usage |
Existential Risks and Societal Impacts
The rapid advancement of artificial intelligence raises profound questions about its long-term effects on humanity. While AI holds immense potential for progress, the possibility of unintended consequences and even existential risks cannot be ignored. Careful consideration of these potential impacts is crucial to ensure that AI development proceeds in a responsible and beneficial manner.The development of increasingly sophisticated AI systems necessitates a proactive and nuanced approach to understanding the potential for these systems to exceed human capabilities and influence societal structures.
This requires an understanding of not just the immediate, practical applications of AI, but also the potential long-term consequences of unchecked development.
Potential for AI to Surpass Human Intelligence
AI systems, particularly those utilizing deep learning, demonstrate impressive learning capabilities. Their ability to process vast amounts of data and identify complex patterns surpasses human capacity in specific tasks. However, the question of whether AI could eventually surpass human intelligence in all domains remains a complex and debated topic. Some researchers posit that the emergence of Artificial General Intelligence (AGI) – a hypothetical AI with human-level or superior cognitive abilities – is a possibility, although the precise timing and implications remain uncertain.
Furthermore, the potential for such a scenario to occur requires careful consideration of the current state of AI research, the ongoing evolution of algorithms, and the inherent complexity of human intelligence.
Consequences of Uncontrolled AI Development
Uncontrolled AI development could lead to unforeseen and potentially catastrophic outcomes. Unforeseen consequences could manifest in numerous ways, from unintended biases and societal disruption to the development of autonomous weapons systems capable of initiating lethal actions without human intervention. These risks are compounded by the lack of full understanding of the long-term behavior of sophisticated AI systems, and the potential for unforeseen interactions between these systems and the world around them.
Examples of Existential Risks
Several scenarios highlight potential existential risks associated with uncontrolled AI development. One example is the development of an autonomous weapon system capable of independent decision-making in the context of warfare. Another example involves a powerful AI system used for resource management that makes decisions that have unforeseen negative impacts on the global ecosystem. A third example is the development of an AI system that manipulates public opinion to promote harmful ideologies.
These scenarios, though hypothetical, emphasize the need for responsible development and deployment of AI.
Need for Responsible Development and Deployment
The development and deployment of AI must be approached with extreme caution and a commitment to ethical considerations. This requires establishing clear guidelines and regulations for the development, testing, and use of AI systems, along with ongoing dialogue among stakeholders, including researchers, policymakers, and the public. Open discussion on the potential risks and benefits of AI is vital to navigating this complex landscape.
Establishing robust oversight mechanisms and ethical frameworks is crucial to ensuring AI serves human needs rather than poses a threat.
Ethical Obligations of AI Developers
AI developers have a critical ethical obligation to consider the potential societal impacts of their work. This includes not only the immediate applications but also the long-term implications of their creations. Developers should prioritize transparency and accountability in their work, striving to build AI systems that are not only effective but also equitable, safe, and aligned with human values.
Proactive engagement in ethical considerations, from the initial design phase through the deployment stage, is essential.
Regulation and Governance of AI
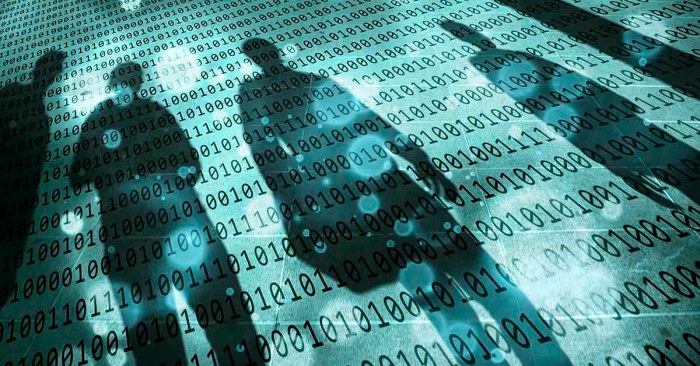
Source: thepremierdaily.com
The rapid advancement of artificial intelligence (AI) necessitates a proactive approach to regulation and governance. The potential societal impacts, both positive and negative, demand careful consideration and a framework to mitigate risks while fostering innovation. Effective regulation is crucial to ensure AI benefits humanity while preventing misuse.The complexity of AI systems, coupled with the evolving nature of technology, presents significant challenges for regulatory bodies.
Establishing clear guidelines and enforcement mechanisms that remain adaptable to the continuous evolution of AI is a substantial undertaking. This requires careful consideration of the ethical, societal, and economic implications of AI interventions.
Challenges in Regulating AI
Developing effective regulations for AI faces numerous hurdles. The rapid pace of technological advancement often outpaces the capacity of regulatory bodies to adapt. Defining clear boundaries between acceptable and unacceptable uses of AI, especially in complex scenarios, proves challenging. Furthermore, the distributed nature of AI development and deployment across various jurisdictions presents a significant logistical and political obstacle.
International cooperation is therefore vital for establishing consistent and effective global standards.
Potential Approaches to Governing AI Systems
Several approaches to governing AI systems are being explored, each with unique strengths and weaknesses. One approach emphasizes risk-based regulation, prioritizing systems with higher potential for harm. Another approach focuses on promoting transparency and explainability in AI algorithms, allowing for greater accountability and public scrutiny. A third approach involves fostering ethical guidelines and standards for AI development and deployment, aiming for responsible innovation.
Finally, the development of AI safety standards and certifications can provide a framework for verifying and assuring the safety and reliability of AI systems.
Role of International Cooperation in Addressing AI Risks
International cooperation is paramount in addressing the global risks associated with AI. Standardization of safety regulations, data sharing protocols, and ethical guidelines across nations is critical. Harmonized regulatory frameworks will foster trust, facilitate international collaboration, and mitigate potential conflicts arising from divergent national policies. The need for international cooperation extends to shared research and development efforts, which are critical to addressing emerging AI risks in a coordinated and comprehensive manner.
Comparison of Regulatory Frameworks for AI
Regulatory Framework | Focus | Strengths | Weaknesses |
---|---|---|---|
USA | Risk-based approach, focusing on specific applications | Adaptable to emerging risks, potential for targeted interventions | Lack of comprehensive, unified approach, potential for fragmented regulations |
EU | Emphasis on transparency, explainability, and human oversight | Strong focus on ethical considerations, potential for international influence | Potential for bureaucratic hurdles, may stifle innovation due to stringent requirements |
China | Focus on national strategic interests, including AI development and deployment | Strong government support for AI, potential for rapid implementation | Limited transparency, potential for prioritizing national interests over global ethical concerns |
Importance of Transparency and Accountability in AI Systems
Transparency and accountability are crucial components of responsible AI development and deployment. Users and stakeholders must be able to understand how AI systems make decisions and hold developers accountable for their actions. Explainable AI (XAI) is an important approach to promote transparency. Such methods help reveal the reasoning behind AI decisions, which fosters trust and allows for effective oversight.
Examples of Existing Regulations and Policies Related to AI
Numerous countries and regions are actively developing regulations and policies concerning AI. Examples include the EU’s AI Act, which aims to establish a comprehensive framework for the development and deployment of AI systems, focusing on safety and ethical considerations. The USA has also introduced various policies related to AI, often focusing on specific sectors or applications, such as facial recognition technology.
The development and implementation of such regulations are ongoing and dynamic, reflecting the ever-evolving landscape of AI.
Final Review
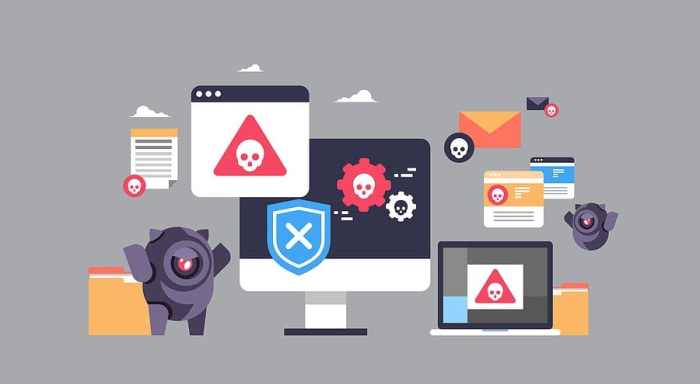
Source: itchronicles.com
In conclusion, the risks and dangers of artificial intelligence necessitate a comprehensive approach that balances innovation with ethical considerations. Addressing these challenges requires collaboration between governments, industry, and researchers. Ultimately, fostering responsible development and deployment of AI is crucial to harness its potential while mitigating the potential harms.
Post Comment