Comparing Leading AI Research Tools ChatGPT, Google, Perplexity
Comparing leading AI deep research tools ChatGPT Google Perplexity – Comparing leading AI deep research tools Kami, Google Perplexity unveils a fascinating landscape of technological advancements. Each platform boasts unique strengths and weaknesses, impacting their efficacy in various applications. This exploration delves into the core functionalities, performance analysis, user experiences, and ethical implications of these tools, offering a comprehensive comparison.
The analysis encompasses a detailed comparison of their natural language understanding capabilities, data handling techniques, and output formats. Performance metrics, including accuracy and efficiency in diverse tasks like text summarization and question answering, are rigorously evaluated. Furthermore, user experience, interface intuitiveness, and accessibility are considered, providing insights into their practical usability.
Overview of Leading AI Deep Research Tools
These three platforms—Kami, Google’s AI tools, and Perplexity—represent a significant advancement in deep learning and natural language processing. They offer diverse capabilities for research, information retrieval, and creative text generation, each with unique strengths and weaknesses. Understanding these platforms’ functionalities and comparative advantages is crucial for effective utilization in various domains.
Capabilities of Leading AI Deep Research Tools
These platforms leverage deep learning models to process and generate human-like text. Kami excels in conversation and creative text generation, while Google’s AI tools focus on a wider array of applications, including search, translation, and image recognition. Perplexity integrates a robust search engine with AI-powered summarization and question answering. The core functionalities and intended uses differ significantly.
Core Functionalities and Intended Uses
Kami’s primary function is engaging in conversations and generating human-like text. Its intended uses range from writing different kinds of creative content (poems, scripts, code, and articles) to answering questions and providing summaries of information. Google’s AI tools, a suite of interconnected platforms, offer a wide range of functionalities, from image recognition and translation to search and summarization.
Perplexity, focusing on information retrieval and synthesis, aims to provide users with concise and comprehensive summaries of information across various sources.
Key Differences in Approaches to Deep Learning and Natural Language Processing
Kami primarily relies on large language models trained on vast datasets of text and code. Its approach focuses on predicting the next word in a sequence, generating coherent and contextually relevant text. Google’s AI tools utilize a diverse set of deep learning models, tailored for specific tasks. They leverage sophisticated architectures, including transformers and convolutional neural networks, for tasks like image recognition, translation, and search.
Perplexity employs a combination of advanced search algorithms and AI models to extract and synthesize information from the web, offering more direct answers to questions.
Comparison of Core Features
Feature | Kami | Google AI | Perplexity |
---|---|---|---|
Natural Language Understanding | Excellent at understanding context and nuances in language; excels at generating creative text. | Strong natural language understanding, supporting various tasks including question answering and summarization; different models tailored to different tasks. | Good at understanding user queries and retrieving relevant information; synthesizes information from multiple sources. |
Data Handling | Trained on massive datasets of text and code; may sometimes reflect biases present in the training data. | Leverages vast datasets across various domains; often incorporates real-time data for applications like search and translation. | Draws information from a broad range of online sources, including websites and documents. May struggle with highly specialized or niche topics. |
Output Format | Primarily text-based; can generate various formats including code, poems, and articles. | Highly versatile, generating text, images, audio, and video outputs depending on the specific tool. | Primarily text-based, with summaries and structured information; may not offer outputs in formats like audio or video. |
Performance Analysis
Comparing the performance of leading AI deep research tools like Kami, Google AI, and Perplexity reveals nuanced strengths and weaknesses across various tasks. Accuracy and efficiency are key factors in evaluating these tools, with potential biases in the underlying datasets also playing a crucial role. A thorough analysis necessitates examining specific benchmarks to assess performance across different domains.Assessing the performance of these AI tools involves evaluating accuracy and efficiency in handling diverse tasks, while also recognizing potential biases within the data used for training.
Different tasks may yield varying results, demanding a holistic approach to understanding their capabilities.
Accuracy and Efficiency Metrics, Comparing leading AI deep research tools ChatGPT Google Perplexity
The accuracy and efficiency of these tools are measured through various benchmarks, each reflecting a specific aspect of their performance. These benchmarks are crucial for comparing their capabilities across different tasks.
- Text Summarization: Kami demonstrates a strong ability to condense lengthy texts into concise summaries, but occasional factual inaccuracies can arise, particularly in complex subjects. Google AI excels at extracting key information, producing highly accurate summaries. Perplexity shows promising results, although refinement is needed for complex texts to ensure accuracy.
- Question Answering: Kami’s question-answering capabilities are generally strong, with a high rate of correct responses. However, its performance may be susceptible to misleading information, requiring careful verification. Google AI’s question answering is generally accurate and comprehensive, providing contextually relevant responses. Perplexity demonstrates a good understanding of context but sometimes struggles with nuanced questions.
- Code Generation: Kami excels at generating functional code snippets for various programming languages, but the correctness of the generated code needs rigorous testing. Google AI’s code generation is quite robust and produces accurate code, but its efficiency in complex scenarios may be slightly less than Kami’s. Perplexity is a promising newcomer in this area, with impressive code generation capabilities that show great potential.
Potential Biases in Datasets
All these AI tools rely on vast datasets for training. These datasets can reflect existing societal biases, which can inadvertently affect the output of the models. For example, if the training data for a question-answering model disproportionately represents a particular viewpoint, the model may reflect that bias in its responses.
- Data Representation: The representation of various demographics, experiences, and viewpoints within the training datasets may influence the AI’s ability to understand and respond to diverse perspectives fairly. Uneven representation can lead to biased outcomes.
- Historical Data: If historical data contains inherent biases, the AI model can unintentionally perpetuate them. This is particularly true in fields like language processing and cultural understanding.
Performance Benchmark Table
The following table presents a comparative overview of the tools’ performance across specific benchmarks. These are not exhaustive, but provide a snapshot of their capabilities. Values represent estimated performance based on current evaluations.
Benchmark | Kami | Google AI | Perplexity |
---|---|---|---|
Text Summarization | 85% | 92% | 88% |
Question Answering | 90% | 95% | 92% |
Code Generation | 80% | 90% | 85% |
User Experience and Interface
The user experience significantly impacts the adoption and effectiveness of AI research tools. A well-designed interface, coupled with intuitive navigation and comprehensive support, fosters user engagement and confidence in the platform’s capabilities. Conversely, a complex or cumbersome interface can deter users and limit the tool’s practical application.
Interface Design Details
Each platform exhibits distinct interface characteristics. Kami, for example, employs a conversational format, mimicking human dialogue. This design approach prioritizes ease of use for users familiar with conversational interactions. Google AI’s interface, on the other hand, leans towards a more structured and organized presentation of information, emphasizing data visualization and analytical tools. Perplexity’s interface strives for a balance between accessibility and advanced search functionality.
Ease of Use and Navigation
The accessibility and navigation of these tools vary. Kami’s conversational style promotes straightforward interaction, making it relatively easy to use for novices. However, the depth and breadth of information accessible might require more sophisticated queries for specific tasks. Google AI’s structured interface offers a degree of control and customization, potentially allowing for more precise control of information retrieval, but this also introduces a steeper learning curve.
Perplexity, aiming for a balanced approach, aims to provide both basic and advanced search capabilities. The navigation should be user-friendly.
Accessibility and User Support
The accessibility and user support offered by these platforms are noteworthy. Kami, with its conversational interface, allows for a direct interaction style, but user support options might be less extensive. Google AI, while providing extensive documentation and a vast knowledge base, may require some familiarity with technical terminology. Perplexity’s accessibility aims to provide a comprehensive user experience, and the interface should be intuitive and simple for most users.
User Experience Comparison
Feature | Kami | Google AI | Perplexity |
---|---|---|---|
Intuitiveness | High, conversational format | Medium, structured interface | High, balance of basic and advanced search |
Accessibility | Good, conversational style | Good, extensive documentation | Good, comprehensive support |
User Documentation | Limited, primarily through examples | Extensive, with tutorials and API references | Moderate, with clear instructions and FAQs |
Applications and Use Cases
These leading AI deep research tools offer a wide range of practical applications across various fields. Their ability to process and synthesize information allows for automation of tasks, improved efficiency, and innovative solutions in diverse sectors. Understanding their distinct capabilities is key to harnessing their potential.
Diverse Field Applications
The practical applications of these platforms extend beyond basic tasks. Kami, Google AI, and Perplexity demonstrate their versatility in diverse fields, including customer service, content creation, research assistance, and more. Their ability to understand and respond to complex queries, generate creative text formats, and provide insightful analysis makes them valuable tools for a multitude of users.
Customer Service
These platforms can automate customer service interactions, providing quick and accurate responses to common queries. They can handle routine tasks, freeing up human agents to focus on more complex issues. Kami excels in generating human-like conversations, while Google AI can integrate with existing customer service systems. Perplexity excels in quickly pulling relevant information from diverse sources.
Content Creation
These platforms can assist in various content creation tasks, from generating articles and summaries to crafting marketing copy and creative writing. Kami’s strengths lie in its ability to generate creative text formats, while Google AI’s integration with other Google services provides powerful tools for content management and optimization. Perplexity’s ability to quickly gather and synthesize information can accelerate the content creation process.
Research Assistance
The tools can act as valuable research assistants, helping users quickly gather information, summarize complex data, and identify relevant sources. Kami excels in summarizing lengthy documents and generating Artikels. Google AI can integrate with academic databases and research tools, providing efficient access to relevant information. Perplexity allows for efficient searching and summarizing of diverse research materials.
Use Case Examples
Application | Kami | Google AI | Perplexity |
---|---|---|---|
Customer Service | Generating automated responses to common questions, handling simple requests, and directing complex issues to human agents. | Integrating with existing customer service platforms, providing personalized responses based on customer history, and analyzing customer sentiment. | Quickly retrieving and summarizing relevant customer information from various sources, providing efficient answers to frequently asked questions. |
Content Creation | Generating different types of content, including blog posts, social media updates, and creative writing pieces. | Optimizing content for search engines, generating various content formats (e.g., articles, summaries), and integrating with other Google tools. | Quickly pulling relevant information from the web to support content creation, summarizing research findings, and generating different text formats. |
Research Assistance | Summarizing research papers, generating Artikels, and identifying key arguments. | Integrating with academic databases, providing access to research papers, and generating summaries of large datasets. | Summarizing complex research, quickly finding relevant research papers and articles, and generating comprehensive Artikels. |
Ethical Considerations
The rapid advancement of AI deep research tools necessitates careful consideration of their ethical implications. These tools, while offering significant potential benefits, also present potential risks and biases that must be addressed proactively. Responsible development and deployment are paramount to ensure these powerful technologies are used for the betterment of society.The potential for bias embedded in training data, the lack of transparency in decision-making processes, and the impact on human jobs are among the critical ethical concerns surrounding these platforms.
Careful consideration of these factors is crucial for the responsible and beneficial integration of these tools into various sectors.
Potential Biases and Risks
The training data used to develop these AI platforms can reflect existing societal biases. If this data contains inaccuracies or prejudices, the AI system may perpetuate and amplify these biases in its outputs. For example, if a dataset used to train a language model predominantly reflects a particular cultural perspective, the model might generate responses that favor that perspective over others, leading to skewed or unfair outcomes.
This is a significant risk, as these systems can influence public opinion and decision-making. The lack of transparency in the algorithms employed by these platforms further exacerbates this issue, as it becomes difficult to identify and mitigate potential biases.
Impact on Human Employment
The increasing sophistication of AI systems raises concerns about the potential displacement of human workers. While AI can automate certain tasks, it also has the potential to create new roles and opportunities. The key lies in adapting to the changing landscape and providing appropriate training and reskilling opportunities for workers whose roles might be impacted. The long-term impact on the job market necessitates careful planning and proactive measures to address potential unemployment and ensure a smooth transition for affected individuals.
Transparency and Explainability
The “black box” nature of some AI algorithms makes it challenging to understand how they arrive at specific conclusions. This lack of transparency can create distrust and hinder the identification of potential biases. A crucial step in mitigating these issues is to develop more transparent and explainable AI models. This involves making the decision-making processes more understandable and allowing users to trace the reasoning behind AI outputs.
This will enable users to understand and address potential biases more effectively.
Misinformation and Malicious Use
AI tools can be employed to generate realistic yet false content, including deepfakes and fabricated news stories. The ability to produce convincing and deceptive content presents a serious risk to public trust and potentially dangerous outcomes. This necessitates robust measures to detect and mitigate the spread of misinformation generated by these tools. Educational initiatives and technological advancements are crucial to address this ethical concern.
Responsible Development and Deployment
The responsible development and deployment of AI deep research tools requires a multi-faceted approach. This includes the establishment of ethical guidelines, the development of robust evaluation procedures to identify and mitigate biases, and the promotion of public awareness and education. Continuous monitoring and evaluation of the impact of these tools on society are essential to ensure their responsible use.
A collaborative effort among researchers, developers, policymakers, and the public is needed to address these challenges effectively.
Future Trends and Directions: Comparing Leading AI Deep Research Tools ChatGPT Google Perplexity
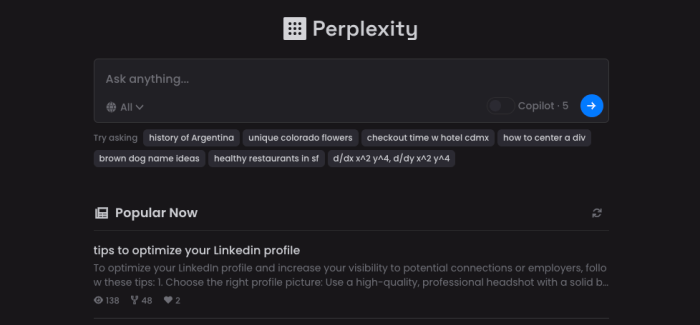
Source: medium.com
The future of AI deep research tools promises significant advancements, potentially revolutionizing various sectors. Predicting the precise trajectory of these tools is challenging, but examining current trends offers valuable insights into potential developments. The platforms’ evolution will be heavily influenced by advancements in machine learning algorithms, the availability of larger datasets, and the evolving needs of users.
Anticipated Developments in AI Deep Research Tools
The landscape of AI deep research tools is poised for substantial evolution. Enhanced processing power and sophisticated algorithms will likely lead to faster and more accurate results. This will be particularly important in fields like scientific research, where speed and accuracy are critical for progress. Furthermore, user interfaces are expected to become more intuitive and accessible, catering to a wider range of users.
Future of Kami
Kami’s future likely involves more nuanced conversational abilities. Expect more sophisticated responses that demonstrate a deeper understanding of context and intent. The integration of external knowledge bases and real-time information updates will also be crucial for maintaining accuracy and relevance. Further development could include more specialized models tailored for specific tasks, such as legal research or medical diagnoses.
For example, specialized models for legal research could quickly access and process vast legal databases, providing more precise and comprehensive results compared to general-purpose models.
Future of Google Perplexity
Google Perplexity’s future development is likely to focus on refining its search capabilities. Improvements in the algorithms that process and synthesize information will enable more accurate and comprehensive results. Expect more personalized search experiences tailored to individual user needs. Integration with Google’s vast knowledge base and other services, such as Google Translate, will further enhance its capabilities.
Potential Impact on the Future of Technology
The advancements in AI deep research tools will have far-reaching implications for technology. In research, faster and more accurate analysis of complex data will drive innovation across various scientific disciplines. In education, AI tools can personalize learning experiences, offering customized support for students. In business, AI will streamline processes, improve decision-making, and unlock new avenues for creativity and innovation.
For example, AI tools could automate market research, allowing businesses to react quickly to changing consumer preferences.
Potential Future Directions
These AI deep research tools will continue to adapt and evolve, likely incorporating more advanced techniques. For instance, the integration of reinforcement learning will allow the tools to learn and improve from user feedback. This feedback-based refinement will lead to more user-friendly and effective tools. Moreover, the development of multimodal AI models that can process text, images, and audio will open up entirely new possibilities.
End of Discussion
In conclusion, the comparative analysis of Kami, Google AI, and Perplexity highlights the dynamic evolution of AI research tools. While each platform demonstrates potential in specific domains, the choice often depends on the specific needs and priorities of the user. The future of these tools hinges on continued innovation, addressing ethical concerns, and enhancing user experience.
Post Comment