Brief History Of Artificial Intelligence
Brief history of artificial intelligence, from its nascent conceptualizations to its modern-day applications, offers a fascinating journey through innovation and evolution. This exploration will trace the key milestones and influential figures that shaped the field, from early pioneers to contemporary researchers. We’ll delve into the motivations behind early AI research, the rise and fall of initial enthusiasm, and the revolutionary impact of machine learning and deep learning on the field.
The evolution of AI is a captivating story of human ingenuity and technological progress. From the philosophical underpinnings of early research to the practical applications of today, the narrative is one of both remarkable achievement and persistent challenges. This journey reveals the remarkable potential of AI while highlighting the crucial ethical considerations that accompany its advancement.
Early Concepts and Pioneers
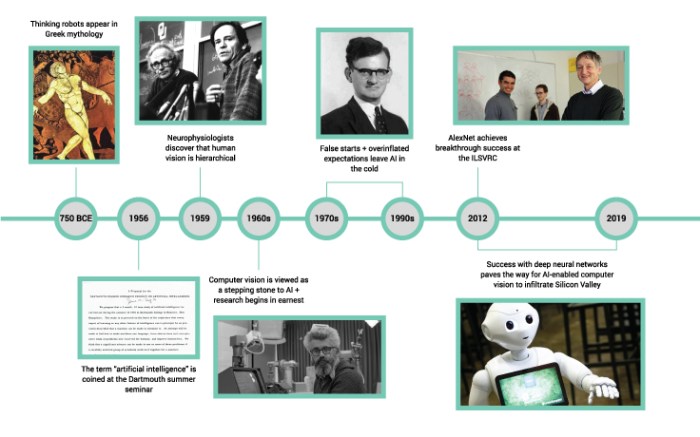
Source: askthecandidates2012.com
The seeds of artificial intelligence were sown long before the digital age, with philosophers and mathematicians pondering the nature of thought and its potential mechanization. Early explorations of intelligence, computation, and the human mind laid the groundwork for the field we know today. These foundational ideas, combined with advancements in computing power, ultimately fueled the development of the first AI systems.The philosophical underpinnings of early AI research were diverse, stemming from inquiries about the nature of consciousness, the possibility of creating thinking machines, and the very definition of intelligence.
Early researchers sought to understand the cognitive processes that underpin human intelligence, and sought to emulate these processes in machines.
Timeline of Key Figures and Contributions
Early figures like Alan Turing, with his seminal work on computability and the Turing Test, significantly shaped the field. His ideas, developed in the mid-20th century, remain foundational to the very concept of artificial intelligence. Other key figures include John McCarthy, considered a founding father of AI, who coined the term “artificial intelligence” and organized the Dartmouth Workshop, a pivotal event in the history of AI.
Claude Shannon, known for his contributions to information theory, also made significant contributions to early AI, particularly in game playing and problem solving.
Philosophical Underpinnings and Motivations
The motivations behind early AI research were multifaceted. Researchers were driven by a desire to understand the human mind, to create machines that could mimic human intelligence, and to explore the limits of computation. These motivations, combined with a growing understanding of computation and information processing, formed the basis for the nascent field of AI.
Differences Between Early Approaches to AI
Early approaches to AI varied considerably. Logic-based systems, a prominent approach, emphasized the use of formal logic and symbolic reasoning to solve problems. These systems represented knowledge using symbols and rules, enabling the manipulation of this knowledge to derive conclusions. Symbolic AI, a broader category encompassing logic-based systems, focused on representing knowledge and reasoning using symbols. These approaches stood in contrast to connectionist approaches, which attempted to model intelligence using networks of interconnected nodes, inspired by the structure of the human brain.
Connectionism emphasized the distributed nature of information processing and learning through patterns.
Examples of Early AI Systems
Early AI systems were rudimentary by today’s standards, but they demonstrated the potential of the field. One example is the Logic Theorist, a program developed by Allen Newell and Herbert Simon, which could prove theorems in symbolic logic. Another noteworthy example is ELIZA, a natural language processing program that simulated a Rogerian psychotherapist, showcasing the potential for machines to engage in rudimentary conversation.
These early systems, while limited in scope, paved the way for more sophisticated AI systems that followed.
Comparison of Early AI Schools of Thought
School of Thought | Key Concepts | Representation of Knowledge | Learning Mechanisms |
---|---|---|---|
Logic-Based Systems | Formal logic, symbolic reasoning | Symbols, rules | Deduction, inference |
Cybernetics | Feedback loops, control systems, self-regulation | Analog representations, continuous variables | Adaptation, learning through feedback |
Connectionism | Neural networks, distributed processing | Weights, connections between nodes | Learning through adjustment of weights |
Early AI research, encompassing logic-based systems, cybernetics, and connectionism, explored various avenues for creating intelligent machines. These different approaches reflected different understandings of intelligence and computation, and their combined contributions form a cornerstone of modern AI.
The Dartmouth Workshop and the Rise of AI
The Dartmouth Workshop, held in 1956, marked a pivotal moment in the nascent field of artificial intelligence. It brought together leading researchers from diverse backgrounds, laying the groundwork for decades of AI development. This meeting fostered a shared vision and set ambitious goals for the future of the field.The workshop’s significance lies not only in its specific achievements but also in its impact on shaping the trajectory of AI research.
The collaborative environment fostered by the event spurred groundbreaking research and paved the way for the development of more sophisticated AI systems. This initial enthusiasm and shared focus on a common goal set the stage for the decades of progress that followed.
Historical Significance of the Dartmouth Workshop
The Dartmouth Workshop, hosted at Dartmouth College, was a watershed moment in the history of artificial intelligence. It brought together prominent figures in the fields of mathematics, computer science, linguistics, and neuroscience. The workshop’s organizers, including John McCarthy, Marvin Minsky, Nathaniel Rochester, and Claude Shannon, envisioned a new era of intelligent machines. The workshop’s impact transcended the specific outcomes of the event, inspiring generations of researchers and laying the foundation for a new field of study.
Key Goals and Outcomes of the Workshop
The primary goal of the Dartmouth Workshop was to explore the feasibility of creating machines capable of simulating human intelligence. Participants aimed to make significant progress in areas like natural language processing, problem-solving, and machine learning. The workshop’s outcome included the development of early AI programs, discussions on key concepts, and the formation of a shared vision for the future of the field.
The workshop’s success stemmed from its collaborative spirit and the ambitious goals articulated by its organizers.
Initial Optimism and Expectations
The initial optimism surrounding the potential of AI was substantial. Participants believed that machines could replicate human cognitive abilities, leading to breakthroughs in problem-solving, decision-making, and automation. This initial optimism was fueled by the rapid advancements in computing technology and the burgeoning field of cybernetics. The attendees anticipated significant advancements in the years following the workshop. The expectation was for substantial progress and tangible applications of AI in various fields.
Early Successes and Limitations
Early AI systems, while limited in scope compared to modern systems, demonstrated some remarkable successes. Early programs could solve simple mathematical problems, play checkers, and translate basic phrases. However, these systems faced significant limitations. The computational power of the time was constrained, and algorithms were rudimentary. The understanding of human intelligence was also incomplete.
Early AI systems, though promising, had significant limitations in their capabilities. Their scope was narrow, their problem-solving abilities were constrained by the available computational power and algorithms, and they lacked a deep understanding of human intelligence.
Participants of the Dartmouth Workshop
Name | Area of Expertise |
---|---|
John McCarthy | Logic, programming languages, artificial intelligence |
Marvin Minsky | Artificial intelligence, cognitive science, computer science |
Nathaniel Rochester | Computer science, information theory |
Claude Shannon | Mathematics, information theory, cryptography |
Allen Newell | Artificial intelligence, computer science |
Herbert Simon | Artificial intelligence, computer science, economics |
Ray Solomonoff | Probability theory, artificial intelligence |
Oliver Selfridge | Pattern recognition, artificial intelligence |
… | … |
The table above provides a glimpse into the diverse expertise represented at the Dartmouth Workshop. These pioneers from various disciplines brought unique perspectives and contributed to the burgeoning field of artificial intelligence. This collaborative effort was crucial for the initial development of the field.
The AI Winter and the Revival: Brief History Of Artificial Intelligence
The initial enthusiasm surrounding artificial intelligence, fueled by early successes and ambitious predictions, eventually waned. This period of reduced funding and diminished interest, known as the AI winter, significantly impacted the field’s progress. Understanding the factors that contributed to this setback and the subsequent resurgence is crucial for appreciating the complex trajectory of AI development.The early AI efforts faced numerous challenges that contributed to the AI winter.
Overly optimistic predictions about the capabilities of early AI systems, combined with the limitations of the available computing power and knowledge representation techniques, often led to unrealistic expectations. The inability to deliver on these promises resulted in disillusionment and a decrease in funding.
Factors Contributing to the Initial AI Winter
The initial AI winter, a period of reduced funding and diminished interest in AI research, stemmed from several key factors. These included the inability to meet overly optimistic predictions, limitations in computing power, and a lack of suitable knowledge representation methods. Furthermore, the complexity of the problems tackled by AI researchers proved to be significantly greater than initially anticipated, leading to frustration and a decrease in enthusiasm.
- Exaggerated Expectations and Unrealistic Promises: Early AI researchers often made bold claims about the near-future capabilities of AI systems. These promises frequently outpaced the actual progress, leading to a gap between expectations and reality. This discrepancy fostered skepticism and disappointment.
- Limited Computing Power: The computational resources available in the early days of AI research were often insufficient to handle the complex tasks required by the algorithms. This limitation hindered the development and testing of sophisticated AI systems.
- Inability to Represent Knowledge Effectively: Early AI systems struggled to represent and manipulate knowledge in a way that was both meaningful and useful. This weakness hampered the ability of AI systems to perform complex reasoning and problem-solving tasks.
- Difficulty in Problem Solving: The problems tackled by AI researchers were often significantly more complex than anticipated. The inherent complexity of these problems, combined with the limitations of early AI techniques, often led to frustrating setbacks.
Challenges and Setbacks in Early AI Efforts
The early AI research faced significant hurdles. These challenges, combined with unrealistic expectations and the limited capabilities of the available technologies, led to the initial AI winter. Addressing these setbacks was crucial for the field’s eventual resurgence.
- Difficulty in Problem Solving: Early AI systems struggled with complex tasks such as natural language processing and image recognition. These difficulties were often exacerbated by limitations in knowledge representation and reasoning capabilities.
- Data Limitations: The availability of high-quality data was a significant constraint in the early days of AI. This scarcity of data often hampered the training and testing of AI models.
- Lack of Robust Algorithms: The algorithms used in early AI systems often lacked the robustness and efficiency required for practical applications. These limitations led to inconsistent performance and unreliable results.
Resurgence of AI Interest in the 1980s and Beyond
The resurgence of AI interest in the 1980s and beyond was driven by several key advancements and breakthroughs. These developments, including the emergence of expert systems and advancements in machine learning, significantly improved the capabilities of AI systems and broadened their applications.
- Expert Systems: The development of expert systems, which mimicked the decision-making processes of human experts, marked a significant advancement. These systems demonstrated the potential of AI for specific problem domains and sparked renewed interest in the field.
- Advances in Machine Learning: Improvements in machine learning techniques, such as backpropagation algorithms, significantly enhanced the ability of AI systems to learn from data. This progress paved the way for more sophisticated and versatile AI applications.
- Increased Computing Power: The growth in computing power, particularly the development of more powerful processors and larger storage capacities, provided the necessary infrastructure for running complex AI algorithms and models.
Advancements and Breakthroughs Leading to Revival, Brief history of artificial intelligence
Significant advancements in computing power, the development of expert systems, and improvements in machine learning techniques played a crucial role in the resurgence of AI. These factors fostered renewed interest and investment in the field.
Event/Factor | Impact on AI Winter/Revival |
---|---|
Increased computing power | Enabled the execution of more complex algorithms and models, leading to breakthroughs. |
Development of expert systems | Demonstrated the practical applicability of AI in specific domains, generating renewed interest. |
Improvements in machine learning | Provided more effective ways for AI systems to learn from data, paving the way for sophisticated applications. |
Increased funding and research opportunities | Stimulated further innovation and development, contributing to the revival. |
Machine Learning and Deep Learning
The journey of artificial intelligence has seen a significant shift with the emergence of machine learning, a subfield focused on enabling computers to learn from data without explicit programming. This evolution, further amplified by deep learning, has led to remarkable advancements in various domains, from image recognition to natural language processing. This section explores the key concepts and developments in machine learning and deep learning, highlighting their evolution and impact.
Early Machine Learning Algorithms
Machine learning’s foundation lies in algorithms capable of identifying patterns and making predictions from data. Early algorithms, such as decision trees and naive Bayes classifiers, were relatively simple but laid the groundwork for more sophisticated models. These initial approaches focused on rule-based systems and statistical methods, demonstrating the potential of automated learning. For example, decision trees can classify data based on a series of if-then-else rules, while naive Bayes utilizes probability calculations to predict class membership.
Supervised, Unsupervised, and Reinforcement Learning
Machine learning encompasses various paradigms, each addressing different learning scenarios. Supervised learning trains models on labeled data, where the input data is paired with corresponding output labels. Unsupervised learning, conversely, works with unlabeled data, aiming to discover inherent structures and patterns. Reinforcement learning, a third paradigm, involves training agents to interact with an environment and learn optimal actions through trial and error.
Each approach plays a crucial role in specific applications, adapting to different data characteristics and learning goals.
Deep Learning Architectures and Applications
Deep learning, a subset of machine learning, leverages artificial neural networks with multiple layers to extract hierarchical features from data. This layered structure allows for increasingly complex representations, enabling models to learn intricate patterns and make accurate predictions. Convolutional Neural Networks (CNNs) excel in image recognition tasks, Recurrent Neural Networks (RNNs) are well-suited for sequential data like text, and Long Short-Term Memory (LSTM) networks are specialized RNNs for handling long-term dependencies in sequences.
Deep learning has revolutionized fields like image recognition, natural language processing, and speech recognition. For example, self-driving cars leverage deep learning for object detection and scene understanding.
Key Breakthroughs in Deep Learning Research
Several key breakthroughs have propelled deep learning forward. The development of efficient algorithms like backpropagation for training deep neural networks was crucial. Advances in computing power, particularly the availability of GPUs, allowed for training increasingly complex models. The availability of massive datasets, such as ImageNet, further fueled the progress of deep learning. These breakthroughs have led to significant performance improvements and broadened the applications of deep learning.
For example, the development of the AlexNet architecture marked a significant milestone in image recognition.
Comparison of Machine Learning Algorithms
Algorithm | Type | Strengths | Weaknesses | Applications |
---|---|---|---|---|
Decision Tree | Supervised | Easy to understand, handles both categorical and numerical data | Prone to overfitting, may not perform well with complex relationships | Classification, prediction |
Naive Bayes | Supervised | Simple, fast, effective for text classification | Assumes feature independence, may not perform well with complex relationships | Spam filtering, text categorization |
Linear Regression | Supervised | Simple, easy to interpret, efficient for linear relationships | Not suitable for non-linear relationships | Predicting house prices, stock prices |
Support Vector Machines (SVM) | Supervised | Effective in high-dimensional spaces, robust to outliers | Computationally expensive for large datasets | Image classification, text classification |
This table provides a concise overview of key differences and similarities between various machine learning algorithms. Each algorithm has its own strengths and weaknesses, making it suitable for specific tasks and datasets.
AI in the Modern Era
Artificial intelligence (AI) has transitioned from theoretical concepts to a pervasive force shaping various aspects of modern life. Its impact is rapidly expanding across industries, demanding a nuanced understanding of its capabilities, limitations, and societal implications. The current landscape of AI research and development is characterized by a blend of innovation and cautious exploration, driven by both the promise of progress and the need for responsible implementation.
Current State of AI Research and Development
AI research today encompasses a wide spectrum of approaches. Significant progress has been made in areas like natural language processing, computer vision, and machine learning algorithms. Advanced techniques like deep learning, employing artificial neural networks, have achieved remarkable results in tasks such as image recognition, speech synthesis, and translation. These advancements are continuously pushing the boundaries of what AI can accomplish.
However, challenges remain in areas such as explainability and robustness, where efforts are focused on developing more transparent and reliable AI systems.
Applications of AI Across Industries
AI is finding applications across diverse sectors, leading to increased efficiency and innovation.
- Healthcare: AI-powered diagnostic tools are enhancing the accuracy and speed of disease detection, personalized medicine approaches are becoming more prevalent, and AI-driven drug discovery processes are accelerating the development of new therapies. For instance, AI algorithms can analyze medical images to identify patterns indicative of diseases like cancer with greater accuracy than human experts in some cases, leading to earlier diagnoses and improved patient outcomes.
AI is also supporting drug development by analyzing vast datasets to identify potential drug candidates and predict their efficacy, reducing the time and cost associated with traditional drug discovery methods.
- Finance: AI algorithms are used for fraud detection, risk assessment, and algorithmic trading. These applications often improve efficiency and accuracy compared to traditional methods, minimizing financial losses. For example, AI systems can detect fraudulent transactions in real-time, safeguarding customer accounts from potential harm.
- Transportation: Self-driving cars are a prominent example of AI’s impact on transportation. AI algorithms enable vehicles to navigate roads, avoid obstacles, and make decisions in complex traffic situations. AI is also enhancing logistics and optimizing delivery routes, leading to cost savings and reduced delivery times.
Impact of AI on Society and the Future of Work
AI’s growing presence is reshaping the future of work, creating both opportunities and challenges. Automation driven by AI can lead to increased productivity and efficiency in many sectors, but it also raises concerns about job displacement. Adapting to the changing job market will require workforce retraining and development programs to equip workers with the skills needed for the evolving job landscape.
AI can also lead to new jobs in areas such as AI development, maintenance, and ethical oversight.
Ethical Considerations Surrounding AI Development and Deployment
Ethical considerations are crucial in the development and deployment of AI systems. Bias in data can lead to discriminatory outcomes, and issues of accountability and transparency need careful consideration. Safeguarding privacy and data security are paramount. The potential for misuse of AI, particularly in areas like autonomous weapons, necessitates careful regulations and ethical guidelines.
Impact of AI in Different Sectors
Sector | Impact | Examples |
---|---|---|
Healthcare | Improved diagnosis, personalized treatment, accelerated drug discovery | AI-powered diagnostic tools, personalized medicine, AI-driven drug discovery |
Finance | Fraud detection, risk assessment, algorithmic trading | Fraud detection systems, risk assessment models, algorithmic trading platforms |
Transportation | Self-driving cars, optimized logistics, route optimization | Self-driving cars, delivery route optimization, logistics management |
Manufacturing | Automation, predictive maintenance, quality control | Automated assembly lines, predictive maintenance systems, quality control tools |
Key Milestones and Technological Advancements
The evolution of artificial intelligence has been profoundly shaped by pivotal milestones and advancements in computing, data, and algorithms. These breakthroughs have not only pushed the boundaries of AI capabilities but also profoundly impacted various sectors of modern life. From early symbolic reasoning systems to the sophisticated deep learning models of today, each advancement built upon the foundations laid by its predecessors.
Breakthroughs in Specific Areas
Significant advancements in AI have occurred across diverse domains, each with its own set of challenges and breakthroughs. Early successes in areas like expert systems demonstrated the potential of AI to automate complex tasks. These early systems, though limited, laid the groundwork for more sophisticated approaches. Later breakthroughs in natural language processing (NLP) and computer vision allowed machines to understand and interact with human language and interpret visual information, respectively.
This progress significantly broadened the applicability of AI.
Influence of Computing Power, Data Availability, and Algorithms
The development of AI has been intricately linked to the increasing power of computing hardware. More powerful processors and specialized hardware like GPUs have allowed for the execution of complex algorithms and the training of sophisticated models. Furthermore, the explosion of data availability has been a crucial factor. Massive datasets provide the necessary fuel for machine learning models to learn and improve their performance.
Sophisticated algorithms, like those underpinning deep learning, have unlocked new levels of performance and accuracy. The combination of these factors has been crucial in accelerating AI development.
Groundbreaking AI Applications and Impact
The practical applications of AI have revolutionized various industries. For example, advancements in computer vision are now powering self-driving cars, enabling them to perceive and react to their surroundings. Deep learning models have revolutionized medical diagnostics, assisting doctors in identifying diseases and predicting patient outcomes. AI is also playing a pivotal role in financial modeling, personalized recommendations, and many other fields.
Table of Key Technological Advancements
Technological Advancement | Description | Impact on AI |
---|---|---|
Increased Computing Power (e.g., GPUs) | Faster processing speeds and parallel processing capabilities | Enabled training of complex models and algorithms |
Explosion of Data Availability | Vast datasets from diverse sources | Provided rich learning materials for machine learning models |
Advancements in Algorithms (e.g., Deep Learning) | Improved learning capabilities and accuracy | Enabled breakthroughs in various AI applications |
Improved Sensors and Data Acquisition Techniques | High-quality data from various sources | Improved accuracy and reliability of AI systems |
Outcome Summary
In conclusion, the brief history of artificial intelligence is a testament to human curiosity and the relentless pursuit of progress. From its philosophical roots to its current state of advancement, AI’s journey has been marked by both remarkable breakthroughs and periods of stagnation. The future of AI promises continued innovation, and understanding its past provides crucial context for navigating its future applications and ethical implications.
Post Comment