The Importance Of Data Privacy In The Age Of Ai And Cloud Computing
The Importance of Data Privacy in the Age of AI and Cloud Computing is paramount in today’s interconnected world. AI systems, increasingly reliant on vast amounts of personal data, and cloud computing, storing and processing this information globally, raise significant privacy concerns. Understanding the intricacies of data protection, legal frameworks, and ethical considerations is essential for individuals, organizations, and governments to navigate this rapidly evolving technological landscape.
This comprehensive exploration dives deep into the multifaceted challenges and opportunities surrounding data privacy. From the foundational principles of data protection to the practical implications of AI and cloud technologies, we will examine the critical issues facing our digital future. We’ll investigate how to create robust systems and practices that prioritize user data privacy while maximizing the potential of these transformative technologies.
Introduction to Data Privacy
Data privacy, in the context of AI and cloud computing, refers to the protection of personal information collected, processed, and stored by these technologies. This encompasses the right to control how one’s data is used, shared, and secured, especially as it becomes increasingly intertwined with decision-making processes and storage in remote locations. It’s critical to balance the benefits of these technologies with the inherent risks to individual privacy.Fundamental principles of data protection are crucial for building trust and ensuring responsible innovation in the age of AI and cloud computing.
These principles are essential for upholding ethical practices and mitigating the potential for misuse or breaches of sensitive data. In the interconnected world of today, data privacy is no longer confined to a single organization or jurisdiction; its scope extends across various platforms and geographical boundaries. This necessitates a global approach to safeguarding personal information.
Defining Data Privacy Concerns
Data privacy concerns in the age of interconnected systems are multifaceted and dynamic. As AI algorithms learn from vast datasets, the potential for bias and discrimination increases. The cloud’s distributed nature complicates data security, necessitating robust encryption and access controls. The growing volume, velocity, and variety of data further exacerbate the challenges of ensuring privacy. These factors highlight the need for proactive measures and a holistic approach to data protection.
Data Privacy Framework
A robust data privacy framework must address several key elements. This framework should be adaptable to evolving technologies and data usage practices. The table below Artikels fundamental principles, their descriptions, examples, and implications within the context of AI and cloud computing.
Principle | Description | Example | Implications |
---|---|---|---|
Purpose Limitation | Data should be collected only for specified, explicit, and legitimate purposes. | Collecting customer data for targeted marketing campaigns but not for political profiling. | Reduces risk of misuse and enhances transparency. Requires clear data collection policies. |
Data Minimization | Only the necessary data for the stated purpose should be collected. | Collecting only essential customer details for order fulfillment, avoiding excessive data collection. | Reduces storage costs, enhances security, and protects personal information. |
Accuracy | Data should be accurate, complete, and up-to-date. | Maintaining accurate customer contact information, promptly updating records. | Improves decision-making, prevents errors in AI systems, and builds user trust. |
Storage Limitation | Data should be stored only for the necessary duration and not retained longer than required. | Deleting customer data after a defined period for abandoned accounts, ensuring data retention policies are clear. | Reduces storage costs, minimizes risks, and prevents data breaches due to outdated information. |
Integrity and Confidentiality | Data should be protected from unauthorized access, use, disclosure, alteration, or destruction. | Using strong encryption for sensitive data in transit and at rest within cloud storage. | Ensures data security, safeguards personal information, and builds user trust in cloud services. |
Accountability | Organizations must be accountable for complying with data protection principles. | Implementing clear data governance policies, establishing mechanisms for data breaches, and providing transparency regarding data usage. | Promotes ethical data handling, fosters trust in organizations, and helps meet regulatory requirements. |
The Impact of AI on Data Privacy
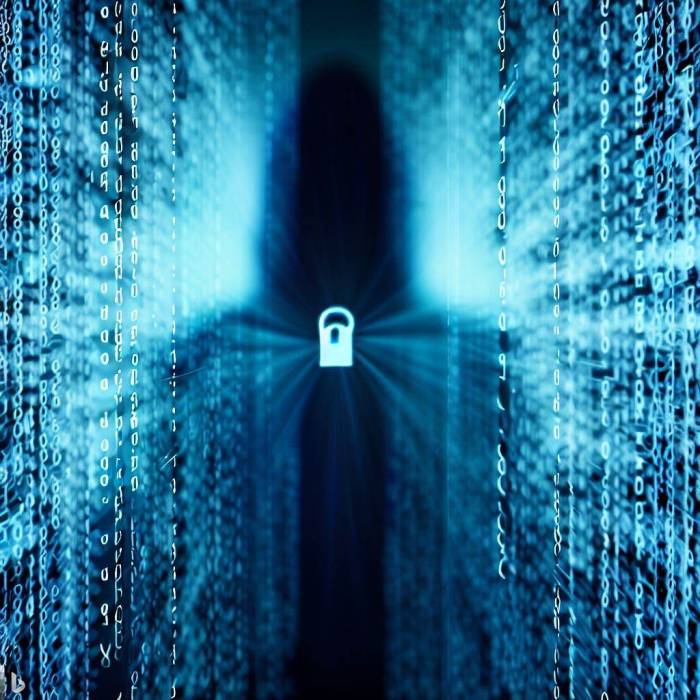
Source: medium.com
Artificial intelligence (AI) systems are increasingly reliant on vast amounts of personal data to function effectively. This dependence necessitates a careful examination of the implications for data privacy. The collection, use, and processing of personal information by AI systems raise significant ethical and legal questions, particularly in light of evolving regulations and societal concerns.AI systems employ various methods to collect, analyze, and interpret personal data, often without explicit user consent or understanding.
This raises concerns about the potential for misuse and the erosion of individual privacy rights. The methods used, the data collected, and the algorithms employed all have significant implications for data privacy.
Data Collection and Usage Methods by AI Systems
AI systems employ diverse methods to collect personal data, ranging from user-generated content to sensor data and publicly available information. These methods include, but are not limited to, social media monitoring, online browsing activity tracking, and the analysis of location data. The scope of data collection varies considerably depending on the specific AI application.
Comparison of Data Privacy Implications Across AI Algorithms and Applications
Different AI algorithms and applications have varying impacts on data privacy. Supervised learning algorithms, for instance, require labeled datasets, potentially exposing sensitive information during training. Unsupervised learning methods, on the other hand, can identify patterns and relationships within large datasets, raising concerns about the privacy implications of discovering sensitive user information. Reinforcement learning algorithms, which learn through trial and error, may involve iterative experimentation on user data, demanding stringent privacy controls.
Potential Risks of Bias and Discrimination in AI Systems
AI systems trained on biased datasets can perpetuate and amplify existing societal biases, leading to discriminatory outcomes. This bias can manifest in various forms, including racial, gender, or socioeconomic bias. These biases can result in unfair or inaccurate predictions, impacting individuals’ access to services or opportunities. For instance, facial recognition systems trained on predominantly white datasets may perform poorly on individuals with darker skin tones.
Challenges in Ensuring Data Privacy with the Increasing Use of Machine Learning
The increasing reliance on machine learning (ML) poses significant challenges to data privacy. The complex nature of ML algorithms can make it difficult to understand how personal data is being used and processed. Moreover, the sheer volume of data used in ML training can raise concerns about data security and the potential for breaches. The “black box” nature of some algorithms further complicates the task of auditing and ensuring data privacy compliance.
Examples of AI-Driven Systems Raising Data Privacy Concerns
The table below illustrates some AI-driven systems that raise data privacy concerns.
AI System | Data Collection Methods | Privacy Concerns |
---|---|---|
Facial Recognition Systems | Camera footage, images from social media | Potential for misuse, lack of transparency in decision-making, potential for bias |
Predictive Policing Systems | Crime data, demographic information | Potential for racial profiling, lack of accountability, skewed resource allocation |
Personalized Advertising Platforms | User browsing history, purchase data | Potential for targeted manipulation, lack of user control over data usage |
Credit Scoring Algorithms | Financial transactions, credit history | Potential for discrimination based on historical biases, lack of transparency in scoring |
The Role of Cloud Computing in Data Privacy

Source: tildeloop.com
Cloud computing has revolutionized data storage and processing, offering scalability and accessibility. However, this shift also brings new challenges regarding data privacy. Understanding the security considerations and best practices for data protection in cloud environments is paramount in this evolving landscape. Robust security measures are crucial to ensure the confidentiality, integrity, and availability of sensitive data.Cloud computing platforms, acting as centralized repositories for data, must be secured effectively.
This includes protecting data from unauthorized access, breaches, and misuse, while ensuring compliance with data privacy regulations. Different cloud service models, each with varying levels of control and responsibility, introduce unique considerations for data privacy. The importance of implementing stringent access control mechanisms cannot be overstated. The same principles of data security apply to cloud-based AI applications, demanding even more scrutiny given the intricate nature of AI algorithms.
Security Considerations in Cloud Environments
Data storage and processing in cloud environments necessitate careful consideration of security vulnerabilities. Potential threats include unauthorized access, data breaches, and loss of data integrity. Malicious actors, accidental data loss, and natural disasters are all potential risks that must be mitigated. Implementing strong security measures is crucial for protecting sensitive data, preventing unauthorized access, and ensuring data integrity.
Security Measures for Protecting Sensitive Data
Robust security measures are employed to safeguard sensitive data in cloud environments. These measures include encryption at rest and in transit, access control lists, intrusion detection systems, and regular security audits. Strong authentication methods, like multi-factor authentication, are crucial to prevent unauthorized access. Regular security assessments, penetration testing, and incident response plans are vital components of a comprehensive security strategy.
Comparison of Cloud Service Models and Data Privacy Implications
Different cloud service models – Infrastructure as a Service (IaaS), Platform as a Service (PaaS), and Software as a Service (SaaS) – offer varying degrees of control and responsibility. IaaS provides the most control, allowing for customization of security measures but demanding a higher level of technical expertise from the user. PaaS offers a more managed environment with pre-built security features, while SaaS provides the most limited control but often includes robust security measures built into the platform.
Each model has specific data privacy implications that need to be carefully evaluated based on the sensitivity of the data being processed.
Importance of Robust Access Control Mechanisms
Access control mechanisms are fundamental to data security in cloud environments. Fine-grained access control, enabling granular permissions and restrictions, is essential to prevent unauthorized access to sensitive data. Least privilege access, granting only the necessary permissions, minimizes the impact of a security breach. Multi-factor authentication adds an extra layer of security to prevent unauthorized access.
Best Practices for Securing Data Privacy in Cloud-Based AI Applications
Securing data privacy in cloud-based AI applications demands a layered approach. Data anonymization and pseudonymization techniques can help protect sensitive information used in training AI models. Regular monitoring of AI models for bias and fairness is essential to prevent discrimination or other unintended outcomes. Thorough documentation of data flows and processing steps is crucial for compliance and auditing purposes.
Comparison of Cloud Providers and their Data Privacy Policies
Provider | Data Security Measures | Data Privacy Policy | Customer Support |
---|---|---|---|
Amazon Web Services (AWS) | Advanced encryption, Identity and Access Management (IAM), Security Hub | Comprehensive data privacy policy addressing various aspects of data protection. | Highly regarded for its comprehensive and responsive customer support. |
Microsoft Azure | Azure Active Directory, Azure Information Protection, Security Center | Detailed policy outlining data protection principles and compliance with regulations. | Widely praised for its technical support and assistance with implementation. |
Google Cloud Platform (GCP) | Data encryption, Identity-Aware Proxy, Cloud Security Command Center | Robust data privacy policy aligning with international standards. | Known for its proactive approach to addressing customer concerns and queries. |
Legal and Regulatory Frameworks for Data Privacy: The Importance Of Data Privacy In The Age Of AI And Cloud Computing
Global data privacy is a complex landscape, shaped by a multitude of legal and regulatory frameworks. These frameworks aim to strike a balance between protecting individuals’ rights and enabling the smooth functioning of businesses, particularly in the rapidly evolving realms of AI and cloud computing. Understanding these regulations is crucial for both organizations seeking to comply and individuals seeking to safeguard their data.
Key Global Data Privacy Laws
A variety of legal frameworks address data privacy globally. These laws differ in their specific provisions, but generally aim to ensure that data is collected, processed, and used responsibly. Different jurisdictions have varying priorities, and the regulations themselves reflect those priorities. Some prioritize the rights of individuals, while others focus on promoting economic activity.
- General Data Protection Regulation (GDPR), EU: The GDPR is a comprehensive regulation that sets stringent standards for the processing of personal data of EU citizens. Key provisions include the rights of data subjects to access, rectify, and erase their data, as well as the right to object to processing. Organizations must demonstrate compliance through transparent data practices, data security measures, and explicit consent.
- California Consumer Privacy Act (CCPA), USA: The CCPA grants California residents specific rights regarding their personal information. These rights include the right to know what personal information is collected, the right to delete personal information, and the right to opt-out of the sale of their personal information. This law has set a precedent for other US states considering similar legislation.
- Personal Information Protection and Electronic Documents Act (PIPEDA), Canada: PIPEDA governs the collection, use, and disclosure of personal information in the private sector in Canada. It establishes principles for fair information practices, emphasizing transparency and accountability.
Roles and Responsibilities in Data Protection
Data protection involves the collaborative efforts of individuals, organizations, and governments. Individuals have the right to expect their data to be handled responsibly, while organizations have a duty to ensure compliance with relevant regulations. Governments play a critical role in establishing and enforcing the legal frameworks. These shared responsibilities are essential for maintaining trust and ensuring data privacy.
- Individuals: Individuals have a right to know how their data is being used and have the power to exercise their rights, including the right to access, rectify, or erase their data.
- Organizations: Organizations are responsible for implementing appropriate measures to safeguard data and complying with all applicable regulations. They need to establish policies, procedures, and security protocols to protect sensitive information. They must be aware of the implications of their data practices, particularly in the context of AI and cloud computing.
- Governments: Governments are responsible for enacting and enforcing data protection laws, and for ensuring that regulations remain relevant and effective in a constantly evolving technological environment. They also need to adapt their policies to new challenges and technologies like AI and cloud computing.
Specific Regulations Impacting AI and Cloud Computing
Data privacy regulations are being adapted to address the unique challenges posed by AI and cloud computing. The use of AI in processing personal data necessitates heightened scrutiny. Cloud computing raises concerns about data security and the location of data processing. Regulations are evolving to incorporate these concerns, and it is a dynamic area of legal development.
Legal Challenges in AI Data Privacy
Implementing data privacy measures in AI systems presents several challenges. AI systems often use complex algorithms and processes that are not fully understood, making it difficult to identify potential privacy risks. Establishing accountability for errors or biases in AI systems is another key challenge. The concept of “explainable AI” is emerging as a possible solution, aiming to enhance transparency and trust in AI decision-making.
This area will require significant legal and ethical developments.
Table: Key Provisions of Prominent Data Privacy Laws
Law | Jurisdiction | Key Provisions | Enforcement Mechanisms |
---|---|---|---|
GDPR | European Union | Rights of data subjects, data minimization, data security, and accountability of controllers. | EU data protection authorities (DPAs) with investigation and enforcement powers. |
CCPA | California, USA | Consumer rights to know, delete, and opt-out of the sale of personal information. | California Attorney General with investigation and enforcement powers. |
PIPEDA | Canada | Fair information practices, principles of accountability and transparency. | Office of the Privacy Commissioner of Canada with investigation and enforcement powers. |
Ethical Considerations of Data Privacy
Data privacy is paramount in the age of AI and cloud computing, where vast amounts of personal data are collected, processed, and analyzed. Ethical considerations are not merely theoretical; they have tangible impacts on individuals and society. These concerns must be addressed proactively to ensure responsible data handling.
Ethical Dilemmas in AI and Cloud Computing
The use of personal data in AI and cloud computing presents complex ethical dilemmas. These dilemmas arise from the potential for misuse, the lack of transparency in algorithmic decision-making, and the difficulties in ensuring accountability for data breaches. Furthermore, the very nature of AI, with its potential for bias and discrimination, introduces significant ethical challenges. Addressing these concerns requires a multifaceted approach involving stakeholders across the spectrum of data handling.
Potential for Misuse and Exploitation
AI systems trained on biased or incomplete datasets can perpetuate and amplify existing societal biases. This can lead to discriminatory outcomes in areas like loan applications, hiring processes, and even criminal justice. Similarly, the vast storage capabilities of cloud computing platforms make them attractive targets for malicious actors. Unauthorized access or data breaches can expose sensitive personal information, leading to identity theft, financial losses, and reputational damage.
Careful consideration of data security protocols and the prevention of malicious activities are crucial.
Transparency and Accountability in Data Handling
Transparency in data handling is essential for building trust. Individuals should have clear visibility into how their data is collected, used, and shared. Likewise, accountability mechanisms are necessary to ensure that those responsible for data breaches or violations are held responsible. Establishing clear guidelines and regulations regarding data handling is paramount to ensuring trust and preventing misuse.
Comparison of Ethical Data Governance Approaches
Various approaches to ethical data governance exist. Some emphasize user consent and control, allowing individuals to actively participate in decisions regarding their data. Others prioritize data minimization, limiting the collection of data to only what is strictly necessary. There are also approaches that focus on the development of robust data security measures to protect against malicious actors.
The choice of approach often depends on the specific context and the potential risks involved.
Ethical Considerations and Principles for Responsible Data Use in AI
Consideration | Description | Example | Implementation Strategies |
---|---|---|---|
Data Bias Mitigation | Identifying and mitigating biases in data sets used to train AI models. | An AI system used for loan applications consistently favors applicants from certain demographic groups, leading to discriminatory outcomes. | Employ diverse datasets for training, use algorithmic auditing tools to identify potential biases, and implement fairness-aware algorithms. |
Data Security and Privacy | Implementing robust security measures to protect personal data from unauthorized access and breaches. | A cloud-based service storing sensitive medical records experiences a data breach, exposing the personal information of thousands of patients. | Implementing encryption, multi-factor authentication, and access controls. Regularly auditing security measures and updating them. |
Transparency and Explainability | Ensuring users understand how AI systems make decisions. | A recommendation engine suggests products to a user without clear explanation of the rationale behind the recommendations. | Develop methods to explain the decision-making process of AI systems. Providing users with access to their data and control over how it is used. |
Accountability and Responsibility | Establishing clear lines of responsibility for data handling and decision-making. | A company is unclear about who is responsible for data breaches and lacks a clear process for handling complaints. | Establishing clear policies and procedures for data handling, implementing an independent oversight body, and providing channels for user feedback and complaints. |
Data Privacy Best Practices for Organizations
Protecting user data in the age of AI and cloud computing necessitates proactive and robust data privacy practices. Organizations must prioritize data minimization, secure storage, and transparent data handling to maintain user trust and comply with regulations. A comprehensive framework, encompassing technical safeguards and employee training, is crucial for mitigating risks and ensuring ethical data use.
Data Minimization and Security Protocols in Cloud Environments, The Importance of Data Privacy in the Age of AI and Cloud Computing
Data minimization, the practice of collecting only the necessary data, is paramount. Organizations should meticulously define the specific data required for their operations and ensure data is stored securely within the cloud environment. This involves implementing strong access controls and encryption protocols to protect sensitive information. Data encryption, both in transit and at rest, is a critical component of this strategy.
Employing robust encryption algorithms and adhering to industry best practices for key management are essential. Organizations should also regularly audit their data storage practices to ensure compliance and identify any vulnerabilities. This proactive approach minimizes the potential for breaches and the associated reputational damage. By storing only necessary data and encrypting it thoroughly, organizations safeguard user privacy while maintaining operational efficiency.
Data Security Protocols
Organizations must implement comprehensive data security protocols, including multi-factor authentication (MFA), regular security audits, and intrusion detection systems. These measures are vital to deter unauthorized access and protect sensitive information. Regular security audits and penetration testing help identify vulnerabilities and assess the effectiveness of existing security measures. A proactive approach to data security, including regular updates of security software and employee training, strengthens the organization’s defenses against potential threats.
Employee Training on Data Privacy Policies and Procedures
Employee training is a crucial aspect of a robust data privacy program. All employees, from data analysts to customer service representatives, need to understand the organization’s data privacy policies and procedures. This training should cover the legal and ethical implications of data handling, the importance of confidentiality, and the proper procedures for reporting suspected data breaches. Consistent training and reinforcement of these policies help to cultivate a culture of data privacy awareness within the organization, reducing the risk of accidental or intentional data breaches.
Key Strategies to Address Data Breaches in AI and Cloud Computing Systems
A proactive approach to data breaches is essential. A robust incident response plan is a critical element. This plan should detail the steps to be taken in case of a breach, including notification procedures, legal counsel engagement, and communication protocols with affected parties. Furthermore, a well-defined process for identifying and mitigating vulnerabilities is crucial. Regular security assessments, penetration testing, and vulnerability scanning are essential tools in this process.
Moreover, maintaining a robust backup and recovery strategy is vital to ensure business continuity in the event of a data breach. Organizations should employ strategies to proactively identify potential threats, such as security awareness training for employees and implementing intrusion detection systems.
- Establish an incident response team: This team should be responsible for coordinating the response to any data breach, including containment, investigation, and recovery.
- Develop a comprehensive incident response plan: This plan should detail the steps to be taken in case of a breach, including notification procedures, legal counsel engagement, and communication protocols with affected parties.
- Implement robust access controls and authentication mechanisms: This includes multi-factor authentication (MFA) to verify user identities and limit unauthorized access.
- Regularly update security software and systems: Keeping software and systems patched and up-to-date is critical to minimizing vulnerabilities.
- Conduct regular security audits and penetration testing: These assessments identify potential vulnerabilities and weaknesses in the system.
- Maintain a strong backup and recovery strategy: This ensures business continuity in the event of a data breach.
- Implement intrusion detection and prevention systems: These systems monitor network traffic for suspicious activity and help to prevent breaches.
Data Privacy and the User Experience
Prioritizing user data privacy is crucial in the age of AI and cloud computing. Users must feel confident that their information is handled responsibly and with respect. This involves designing systems that are transparent, empower users with control, and integrate privacy policies seamlessly into the user experience. This section explores best practices for creating a positive and secure user experience around data privacy.
Designing AI Systems and Cloud Services for Data Privacy
AI systems and cloud services should be designed with data privacy in mind from the outset. This proactive approach helps avoid privacy breaches and fosters user trust. A robust privacy design considers data minimization, secure storage, and encryption. Furthermore, systems should be designed to limit data collection to only what is necessary for the intended purpose.
User Transparency and Control
User transparency and control are key elements of a positive data privacy experience. Clear explanations of how data is collected, used, and shared are essential. Users should have readily available tools to manage their data, including the ability to access, modify, and delete their information. This fosters a sense of ownership and control over personal data.
User Guide for Data Protection
This guide Artikels how users can protect their data in the age of AI and cloud computing. Understanding data privacy policies and practices is the first step. Actively reviewing privacy settings and adjusting them to meet personal needs is crucial. Choosing services with strong privacy policies and practices is also recommended. Finally, users should be aware of phishing scams and other threats targeting personal data.
Integrating Privacy Policies into the User Interface
Privacy policies should not be buried in lengthy documents. Instead, they should be accessible and integrated directly into the user interface. Clear, concise summaries and easily navigable links to complete policies are vital. Consider incorporating interactive elements within the user interface to provide immediate answers to privacy questions. Visual cues, like icons or badges, can also highlight the privacy features of the service.
User Rights Related to Data Access, Correction, and Deletion
Understanding user rights regarding data access, correction, and deletion is essential. These rights empower users to maintain control over their personal data. The table below Artikels these rights and how they might be reflected in a user interface.
Right | Description | Example | User Interface Considerations |
---|---|---|---|
Data Access | The right to request and obtain a copy of the personal data held about them. | A user requests a copy of their browsing history from a website. | A dedicated “View My Data” section within the user account, allowing users to download their data in a standard format. |
Data Correction | The right to request corrections or updates to inaccurate or incomplete personal data. | A user discovers an error in their address on a social media platform and requests a correction. | A “Edit Profile” section with clear fields for correcting information, with validation to ensure accuracy. Immediate confirmation of changes. |
Data Deletion | The right to request the deletion of their personal data. | A user wants their account deleted from a service they no longer use. | A “Delete Account” option in the user account settings, with a confirmation step to prevent accidental deletions. Clear instructions on the implications of deletion. |
End of Discussion
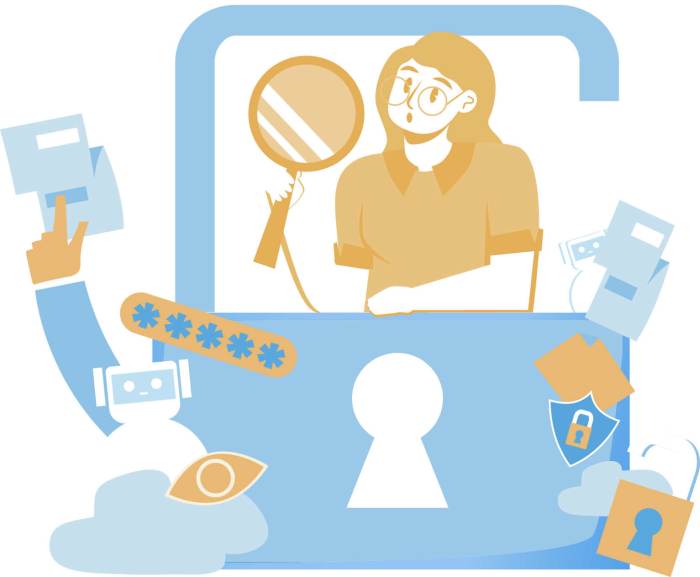
Source: inquirer.net
In conclusion, safeguarding data privacy in the era of AI and cloud computing demands a holistic approach. Addressing the legal, ethical, and practical aspects of data protection is critical for building trust and ensuring a responsible digital future. This discussion highlights the intricate interplay between technological advancement and human rights, emphasizing the importance of proactive measures to protect personal data in this evolving landscape.
Post Comment