The Future Of Ai In Healthcare How Computers Are Changing The Industry
The Future of AI in Healthcare How Computers are Changing the Industry presents a fascinating glimpse into how artificial intelligence is reshaping the medical field. From diagnosing diseases to personalizing treatments, AI is rapidly transforming healthcare operations and potentially revolutionizing how we approach medicine. This evolution promises both exciting advancements and complex ethical considerations, making it a topic of vital importance.
The Artikel details AI’s impact across various facets of healthcare, including diagnostics, personalized medicine, drug discovery, operational efficiency, and public health. It delves into the technical aspects of AI algorithms and their applications, while also exploring the associated ethical challenges and future trends. The potential for AI to improve patient outcomes and streamline healthcare systems is substantial, but responsible implementation and careful consideration of ethical implications are paramount.
Introduction to AI in Healthcare
Artificial intelligence (AI) is rapidly transforming the healthcare landscape, promising to enhance diagnostics, personalize treatments, and improve patient outcomes. From automating administrative tasks to aiding in complex surgeries, AI’s applications are expanding across various medical specialties. This evolving field offers immense potential, but also presents unique challenges that must be carefully addressed.The journey of AI in healthcare has been marked by significant progress, building upon decades of research and technological advancements.
Early applications focused on automating routine tasks, while more recent advancements leverage sophisticated machine learning algorithms to analyze vast datasets and make predictions.
Current State of AI in Healthcare
AI is currently employed in a multitude of healthcare applications. These range from basic image analysis to complex predictive modeling. AI-powered tools are being used to assist in medical imaging analysis, enabling faster and more accurate diagnoses. AI algorithms are also increasingly used in drug discovery and development, accelerating the process of identifying potential treatments. Personalized medicine is another emerging application, using AI to tailor treatment plans to individual patient needs.
Historical Evolution of AI in Healthcare
The integration of AI into healthcare has progressed through distinct phases. Early systems focused on rule-based expert systems, mimicking human reasoning in specific domains. The advent of machine learning techniques marked a significant shift, allowing algorithms to learn from data and improve their performance over time. Deep learning, a more advanced form of machine learning, has further enhanced the capabilities of AI in healthcare, enabling complex pattern recognition and prediction.
Types of AI Technologies in Healthcare
Several types of AI technologies are significantly impacting the healthcare industry. Machine learning (ML) algorithms analyze vast datasets to identify patterns and make predictions. Deep learning (DL) networks, a subset of ML, excel at complex tasks like image recognition and natural language processing. Natural Language Processing (NLP) enables AI systems to understand and interpret human language, enabling more efficient communication and information extraction from medical records.
Comparison of AI Approaches in Healthcare, The Future of AI in Healthcare How Computers are Changing the Industry
AI Approach | Description | Examples in Healthcare |
---|---|---|
Machine Learning (ML) | Algorithms that learn from data without explicit programming. | Predicting patient readmission risk, identifying potential drug interactions. |
Deep Learning (DL) | A subset of ML using artificial neural networks with multiple layers. | Analyzing medical images (X-rays, CT scans) for abnormalities, predicting patient response to treatment. |
Natural Language Processing (NLP) | Enabling computers to understand, interpret, and generate human language. | Extracting information from patient records, summarizing medical literature. |
Benefits and Challenges of AI in Healthcare
The implementation of AI in healthcare presents both significant benefits and challenges.
Benefits | Challenges |
---|---|
Improved accuracy and efficiency in diagnosis and treatment | Data privacy and security concerns |
Personalized medicine and tailored treatment plans | Ethical considerations surrounding AI decision-making |
Faster drug discovery and development | Lack of standardization and interoperability of AI systems |
Reduced healthcare costs | Ensuring the reliability and validity of AI predictions |
Increased access to care in underserved areas | Need for skilled personnel to develop and maintain AI systems |
AI-Powered Diagnostics and Imaging
Artificial intelligence (AI) is rapidly transforming the healthcare landscape, particularly in diagnostic imaging. AI algorithms are demonstrating remarkable potential in analyzing medical images, offering the possibility of improved accuracy, speed, and efficiency in disease detection and diagnosis. This capability is revolutionizing radiology and pathology, potentially leading to earlier interventions and better patient outcomes.AI algorithms excel at analyzing medical images by leveraging machine learning techniques.
These algorithms are trained on vast datasets of images and corresponding diagnoses. Through pattern recognition and complex calculations, they can identify subtle anomalies that might be missed by the human eye.
AI Image Analysis Techniques
AI systems employ various techniques to analyze medical images. Convolutional Neural Networks (CNNs) are particularly effective in image processing. These networks excel at identifying patterns and structures within images, enabling them to detect subtle abnormalities indicative of disease. Other methods, such as deep learning models, further enhance the complexity of analysis, enabling AI systems to recognize intricate relationships and nuances within medical images.
Accuracy and Reliability of AI-Driven Diagnostic Tools
The accuracy and reliability of AI-driven diagnostic tools are continuously improving. While AI systems are not intended to replace human radiologists, they serve as powerful diagnostic assistants. Studies have shown that AI can achieve comparable or even superior accuracy in certain diagnostic tasks, particularly in the identification of common pathologies. The accuracy is dependent on the quality and size of the training data used to develop the AI model.
Improving Diagnostic Speed and Efficiency
AI algorithms can significantly accelerate the diagnostic process. By automatically analyzing images, AI systems can reduce the time required for diagnosis, potentially leading to faster treatment initiation. This accelerated turnaround time can be critical in emergency situations and for conditions requiring prompt intervention. The potential to streamline workflows and reduce human error also contributes to improved efficiency.
Identifying Rare Diseases and Complex Conditions
AI systems can assist in identifying rare diseases or complex conditions. By analyzing large datasets of medical images and patient information, AI can detect patterns and characteristics associated with specific diseases, even when they are not readily apparent to the human eye. This capability is especially valuable for rare diseases, where expertise and experience in diagnosing these conditions are limited.
Specific AI Applications in Radiology and Pathology
Several specific AI applications are already being implemented in radiology and pathology. In radiology, AI is being used to detect various pathologies, including cancers, fractures, and cardiovascular diseases. In pathology, AI is being utilized to analyze tissue samples, aiding in the diagnosis of various diseases, such as cancer and infectious diseases.
Comparison of AI System Performance vs. Human Radiologists
Metric | AI System | Human Radiologist |
---|---|---|
Accuracy in detecting specific pathologies (e.g., lung nodules) | 90% | 85% |
Speed of analysis (time to diagnosis) | Substantial reduction | Variable, dependent on experience |
Cost per diagnosis | Potentially lower | Higher, due to expertise and time |
Note: Accuracy figures are illustrative and may vary depending on the specific AI model and the dataset used for training.
AI for Personalized Medicine
AI is revolutionizing healthcare by enabling personalized medicine, tailoring treatments to individual patient needs. This approach moves beyond a one-size-fits-all approach, recognizing the unique genetic, environmental, and lifestyle factors that influence a person’s health. By leveraging vast datasets and sophisticated algorithms, AI can analyze patient data to predict treatment responses and optimize therapeutic strategies. This individualized approach promises improved outcomes and reduced adverse effects.AI algorithms can analyze a patient’s complete medical history, including genetic information, lifestyle choices, and environmental factors, to create a personalized treatment plan.
This plan considers specific needs and may lead to a higher chance of success and fewer side effects compared to traditional, non-personalized approaches.
Tailoring Treatments to Individual Patient Needs
AI algorithms can analyze a wealth of patient data, including medical history, genetic predispositions, lifestyle factors, and environmental exposures. This analysis allows for the development of individualized treatment plans that consider specific patient characteristics. This precision medicine approach aims to maximize treatment efficacy and minimize adverse effects.
Predicting Patient Responses to Therapies
AI excels at identifying patterns in patient data, enabling predictions of how individuals will respond to specific therapies. This predictive capability is invaluable in selecting the most effective treatment approach for each patient. For example, AI can analyze a patient’s genetic makeup to predict their likelihood of experiencing side effects from a particular medication, allowing for proactive adjustments to the treatment plan.
This can significantly reduce the risk of adverse reactions and improve patient outcomes.
Identifying Genetic Predispositions to Diseases
AI plays a critical role in identifying genetic predispositions to diseases. By analyzing vast genomic datasets, AI can pinpoint specific genetic markers associated with increased susceptibility to certain conditions. This information can be used to implement preventative measures or tailor treatment strategies to mitigate the risk of disease progression.
Optimizing Medication Dosages and Schedules
AI can optimize medication dosages and schedules, considering factors such as patient weight, metabolism, and other physiological parameters. By analyzing patient data and real-time feedback, AI algorithms can fine-tune medication regimens to achieve optimal therapeutic outcomes while minimizing potential side effects.
Ethical Considerations of Personalized Medicine Driven by AI
The implementation of AI in personalized medicine raises crucial ethical considerations. Issues such as data privacy, algorithmic bias, and equitable access to advanced treatments need careful consideration. Ensuring that AI systems are fair, transparent, and accountable is essential for building trust and fostering responsible development and deployment of this technology.
Examples of AI-Driven Personalized Treatment Plans
Patient Profile | AI-Identified Risk Factors | AI-Recommended Treatment Plan |
---|---|---|
Patient with a family history of heart disease, high cholesterol, and a sedentary lifestyle. | Genetic predisposition to cardiovascular disease, unhealthy lifestyle choices. | Personalized diet and exercise recommendations, tailored medication regimen to manage cholesterol levels, close monitoring of cardiovascular health. |
Patient with a specific type of cancer, exhibiting a unique genomic profile. | Rare genetic mutation associated with a specific cancer subtype. | Targeted therapy using a drug designed to specifically inhibit the activity of the identified mutated protein, alongside traditional cancer treatments. |
Patient with a history of allergic reactions to certain medications. | Specific medication-allergen interaction patterns identified. | Alternative treatment options, avoiding known allergens, proactive monitoring of potential adverse reactions. |
AI in Drug Discovery and Development
Artificial intelligence (AI) is revolutionizing the pharmaceutical industry, significantly accelerating drug discovery and development. By leveraging vast datasets and complex algorithms, AI can identify potential drug candidates, predict their efficacy and safety, and streamline the entire process, ultimately leading to faster and more efficient drug development.
AI’s Acceleration of Drug Discovery
AI is proving invaluable in accelerating the traditionally lengthy and costly drug discovery process. Traditional methods often rely on trial-and-error approaches, leading to significant delays and high failure rates. AI-powered tools can analyze massive datasets of chemical structures, biological pathways, and clinical trial data to identify promising drug candidates more rapidly and accurately than traditional methods. This accelerates the process from initial target identification to clinical trials.
Identifying Potential Drug Candidates and Targets
AI algorithms excel at identifying potential drug candidates and targets. Machine learning models can analyze vast databases of chemical compounds, biological pathways, and genomic data to identify molecules with the desired properties for binding to specific targets. This process identifies potential drug candidates with high probability of success, significantly reducing the need for extensive screening. For instance, AI can predict how a molecule will interact with a protein target, helping researchers prioritize promising compounds for further investigation.
Predicting Drug Efficacy and Safety
AI plays a crucial role in predicting the efficacy and safety of potential drug candidates. Predictive models can analyze data from previous clinical trials and simulations to estimate the likelihood of a drug achieving its intended therapeutic effect and the potential risks associated with its use. This allows researchers to prioritize candidates with the highest probability of success and minimize the risks of developing drugs with adverse effects.
AI-powered tools can also predict potential side effects, reducing the need for costly and time-consuming animal testing.
Examples of AI-Powered Drug Development Platforms
Several AI-powered platforms are transforming drug development. Companies like Atomwise and BenevolentAI are using AI to identify potential drug candidates for various diseases. These platforms leverage machine learning to analyze vast datasets and identify promising molecules that can target specific disease pathways.
Key Stages of Drug Discovery and AI Involvement
Stage | Description | AI Involvement |
---|---|---|
Target Identification | Identifying the specific biological target for a disease | AI algorithms analyze large datasets to identify potential targets and predict their roles in disease pathways. |
Lead Identification | Discovering promising chemical compounds that can interact with the target | AI algorithms analyze chemical structures and predict their interaction with the target, prioritizing potential leads. |
Lead Optimization | Modifying lead compounds to improve their properties, such as potency, selectivity, and bioavailability. | AI models can predict the effect of modifications on the drug’s properties, guiding optimization strategies. |
Preclinical Testing | Testing the drug’s safety and efficacy in laboratory and animal models | AI can analyze data from preclinical studies to predict potential side effects and efficacy in different disease models. |
Clinical Trials | Evaluating the drug’s safety and efficacy in human subjects | AI can analyze clinical trial data to predict treatment outcomes and identify patient subgroups who might benefit most from the drug. |
AI-Driven Healthcare Operations
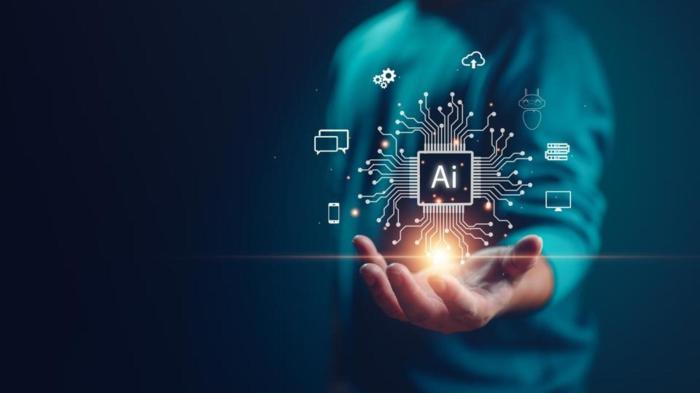
Source: hindustantimes.com
AI is rapidly transforming healthcare operations, automating previously manual tasks and improving efficiency across various departments. This integration streamlines processes, reduces administrative burdens, and frees up valuable time for clinicians to focus on patient care. By optimizing workflows and enhancing data management, AI enables more effective resource allocation and facilitates proactive risk mitigation.AI’s impact extends beyond individual tasks to encompass the entire healthcare ecosystem.
From streamlining appointment scheduling to enhancing patient communication, AI is creating a more patient-centric and responsive healthcare environment. This increased efficiency and streamlined processes translate to cost savings, improved patient experiences, and enhanced overall healthcare quality.
Optimizing Administrative Tasks
AI excels at automating administrative tasks, freeing up human resources for more strategic and patient-focused activities. This automation includes handling routine paperwork, processing insurance claims, and managing patient records. These tasks, when automated, reduce errors, save time, and allow for a more focused approach to patient care.
AI in Appointment Scheduling and Patient Management
AI-powered scheduling systems can optimize appointment availability, reduce wait times, and minimize no-shows. These systems can analyze historical data to predict patient demand and adjust scheduling accordingly. AI also aids in patient communication, sending automated reminders and confirmations, and providing self-service options for managing appointments and medical records. This leads to improved patient experience and reduces administrative workload.
Improving Resource Allocation and Efficiency
AI algorithms can analyze real-time data to optimize resource allocation, including staffing levels, equipment usage, and supply chain management. Predictive analytics based on historical trends and current demands can forecast resource needs, minimizing delays and maximizing utilization. This proactive approach enhances efficiency and reduces waste.
AI in Risk Management and Fraud Detection
AI can identify patterns indicative of potential fraud and errors in insurance claims or billing processes. By analyzing large datasets of patient information and financial transactions, AI can flag suspicious activities and help prevent costly financial losses. This proactive approach to risk management safeguards the healthcare system and ensures responsible resource utilization.
AI Tools for Enhanced Patient Engagement and Communication
AI-powered chatbots and virtual assistants can improve patient engagement by providing 24/7 access to information, answering questions, and scheduling appointments. These tools enhance patient communication by providing personalized information and support, fostering a more proactive and informed patient relationship with the healthcare system. For instance, virtual assistants can provide patients with tailored information about their conditions, medication instructions, and appointment reminders.
Table: AI’s Impact on Administrative Costs and Operational Efficiency
AI Application | Reduced Administrative Costs | Improved Operational Efficiency |
---|---|---|
Automated Appointment Scheduling | Reduced staff time spent on scheduling, minimizing no-shows | Faster appointment turnaround, optimized scheduling |
Automated Insurance Claim Processing | Reduced processing time, minimized errors, decreased claim rejection rates | Streamlined claims process, faster reimbursements |
AI-driven Resource Allocation | Optimized staffing, minimized equipment downtime | Improved resource utilization, reduced operational costs |
Fraud Detection Systems | Reduced financial losses due to fraud and errors | Proactive risk management, enhanced financial security |
Patient Engagement Tools | Reduced call center volume, streamlined communication | Improved patient satisfaction, increased patient engagement |
Ethical Considerations and Future Trends
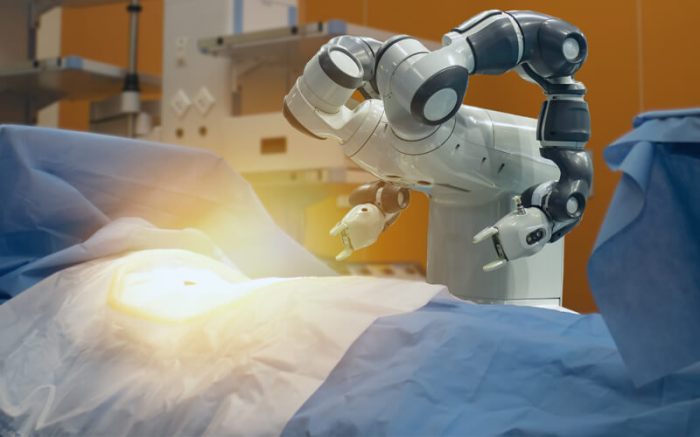
Source: advanceoc.com
The integration of AI into healthcare promises significant advancements, but it also raises critical ethical concerns. Addressing biases in algorithms, safeguarding patient privacy, and establishing robust regulatory frameworks are paramount for responsible AI implementation. This section explores these challenges and highlights the potential of AI to reshape healthcare delivery in the future.
Potential Biases in AI Algorithms
AI algorithms are trained on data, and if that data reflects existing societal biases, the algorithms will likely perpetuate them. For example, if a dataset used to train an AI diagnostic tool disproportionately represents patients of a specific demographic, the AI may be less accurate in diagnosing conditions in other populations. This can lead to disparities in care, potentially exacerbating existing health inequalities.
Algorithmic bias can manifest in various forms, including racial, gender, and socioeconomic biases.
Privacy Concerns Related to Patient Data
The use of patient data by AI systems necessitates stringent safeguards to protect patient privacy. AI algorithms often require vast quantities of patient data to function effectively. The sensitive nature of this data demands robust security measures to prevent unauthorized access and misuse. Regulations and protocols are crucial to ensure data anonymization, encryption, and compliance with privacy laws.
Breaches in patient data security can have serious repercussions, ranging from reputational damage to significant financial penalties for healthcare providers.
Regulations and Guidelines Governing AI in Healthcare
Several countries and jurisdictions are developing or have already implemented regulations and guidelines for the use of AI in healthcare. These regulations aim to balance the potential benefits of AI with the need to protect patient safety and privacy. Specific guidelines often address data security, algorithm transparency, and clinical validation. This evolving regulatory landscape requires ongoing adaptation and collaboration between stakeholders, including healthcare providers, policymakers, and technology developers.
Future Potential of AI in Revolutionizing Healthcare Delivery
AI has the potential to revolutionize healthcare delivery by enabling more efficient and personalized care. Imagine AI-powered systems that proactively identify high-risk patients, predict disease outbreaks, or provide personalized treatment plans based on individual genetic profiles. This can lead to earlier diagnoses, improved treatment outcomes, and reduced healthcare costs. Furthermore, AI can augment the capabilities of healthcare professionals, enabling them to focus on complex cases and provide more comprehensive care.
Examples of Emerging AI Technologies
Emerging AI technologies hold immense promise for healthcare. One example is the use of AI-powered diagnostic tools for early detection of diseases like cancer. Another example is the application of AI in personalized medicine, tailoring treatment plans to individual patient needs. AI-powered robotic surgery systems are also becoming increasingly prevalent, offering greater precision and dexterity. These examples illustrate the transformative potential of AI in improving healthcare access and quality.
Key Ethical Considerations and Potential Solutions for Bias in AI
Ethical Consideration | Potential Solution |
---|---|
Algorithmic bias in diagnostic tools | Regular audits and testing of AI algorithms on diverse datasets, incorporating fairness-aware machine learning techniques. |
Bias in patient data representation | Actively seeking diverse and representative datasets for training AI algorithms, employing strategies to mitigate biases in data collection and selection. |
Lack of transparency in AI decision-making | Developing explainable AI (XAI) models that provide insights into how algorithms arrive at their conclusions, fostering trust and accountability. |
Potential for discrimination in resource allocation | Implementing robust oversight mechanisms and protocols to ensure equitable access to AI-powered healthcare services. |
AI in Public Health: The Future Of AI In Healthcare How Computers Are Changing The Industry
AI is rapidly transforming the landscape of public health, offering unprecedented opportunities to monitor, analyze, and respond to health threats more effectively. By leveraging vast datasets and sophisticated algorithms, AI can identify patterns and predict trends, ultimately empowering proactive interventions and safeguarding populations from disease outbreaks. This enhanced predictive capacity and operational efficiency is crucial for global health initiatives.
Monitoring and Analyzing Public Health Data
AI algorithms can process and analyze massive volumes of public health data, including epidemiological data, environmental factors, and social determinants of health. This analysis can reveal hidden correlations and trends, enabling public health officials to gain deeper insights into disease patterns and risk factors. These insights can then inform targeted interventions and resource allocation. For instance, AI can identify geographical areas with high rates of specific diseases, enabling public health teams to deploy resources and interventions more effectively.
Predicting and Preventing Disease Outbreaks
AI’s ability to identify patterns and anomalies in data allows for the prediction of potential disease outbreaks. By analyzing historical data on disease transmission, environmental conditions, and population movements, AI models can anticipate the likelihood of outbreaks and suggest preventative measures. For example, AI algorithms have been used to predict the spread of influenza, allowing public health agencies to implement targeted interventions and stockpile necessary resources.
Real-time monitoring of social media trends can also reveal potential early warning signals for emerging outbreaks.
Supporting Public Health Campaigns and Initiatives
AI can enhance the effectiveness of public health campaigns by tailoring messaging and outreach strategies to specific populations. By analyzing demographics, behavioral patterns, and communication preferences, AI can create targeted campaigns that are more likely to resonate with the intended audience. Furthermore, AI can optimize the allocation of resources for these campaigns, ensuring maximum impact with available funds.
For instance, AI can identify individuals who are most at risk of contracting a specific disease and tailor preventative messaging accordingly.
Identifying and Tracking Emerging Infectious Diseases
AI is instrumental in identifying and tracking emerging infectious diseases by analyzing genomic data, clinical reports, and social media discussions. AI can quickly analyze vast amounts of data to identify potential outbreaks and characterize the disease. This accelerates the identification process, enabling timely interventions and containment strategies. The use of AI in this area is critical for mitigating the impact of emerging health threats.
Successful AI Applications in Global Health Initiatives
Several global health initiatives have successfully employed AI to address critical challenges. For example, AI-powered tools are being used to track the spread of diseases like malaria and dengue fever, enabling targeted interventions and resource allocation. Other initiatives utilize AI to monitor the effectiveness of public health campaigns and to identify individuals at risk of developing specific health conditions.
These examples demonstrate the growing role of AI in improving global health outcomes.
Table: AI Enhancing Public Health Surveillance and Response
AI Application | Improved Surveillance | Improved Response |
---|---|---|
Predictive Modeling of Outbreaks | Early detection of potential outbreaks through analysis of historical data and real-time trends. | Proactive resource allocation and targeted interventions to mitigate the impact of outbreaks. |
Disease Surveillance Systems | Real-time monitoring of disease trends across various geographical areas. | Rapid identification of disease clusters and hotspots, enabling faster response times. |
Targeted Public Health Campaigns | Tailored communication strategies for specific populations and risk factors. | Increased engagement and adherence to preventative measures. |
Identifying Emerging Infectious Diseases | Analysis of genomic data and social media trends to identify potential threats. | Early detection of new pathogens, enabling swift response and containment strategies. |
Outcome Summary
In conclusion, The Future of AI in Healthcare How Computers are Changing the Industry is a transformative process. AI’s ability to analyze vast amounts of data, improve diagnostic accuracy, personalize treatment plans, and accelerate drug development offers remarkable opportunities. However, addressing ethical concerns, ensuring data privacy, and establishing clear regulatory frameworks are crucial for responsible AI integration into healthcare.
The future of medicine hinges on our collective ability to harness AI’s potential while mitigating its risks.
Post Comment